Detecting Wind Turbine Anomalies Using Nonlinear Dynamic Parameters-Assisted Machine Learning with Normal Samples.
Reliability engineering & systems safety(2023)
摘要
Anomaly detection is critical for the reliability and safety of wind turbine. Toward this objective, this paper proposes an anomaly detection scheme for wind turbine using only normal samples. Specifically, a novel nonlinear tool, generalized multiscale Poincare plots (GMPOP), is firstly developed to capture the behavior changes of vibration signals through scales. Subsequently, support vector data description (SVDD) is established to learn the acceptance region defined for anomaly detection from the GMPOP information under normal con-ditions. The feasibility of GMPOP is initially studied on simulated signals. Further, two datasets, from an experimental bearing and a 2 MW wind turbine, are analyzed to illustrate the evolution process. Comparing to such the state-of-the-art indicators as root mean square, permutation entropy and dispersion entropy, the GMPOP-based method can successfully detect the state anomalies of before the occurrence of the outer race fault and inner race fault, and provide anomaly alarms in advance. The study suggests that the proposed GMPOP is an effective way to assess the dynamical alteration of wind turbine. Moreover, compared with other baselines: auto-encoder, principal components analysis, minimum covariance determinant and exponentially weighted moving average control chart, the proposed detection model GMPOP-SVDD produces favorable performance regarding multiple aspects.
更多查看译文
关键词
Condition monitoring,Anomaly detection,Rotating machine,Wind turbine,Generalized multiscale Poincare plots,SVDD
AI 理解论文
溯源树
样例
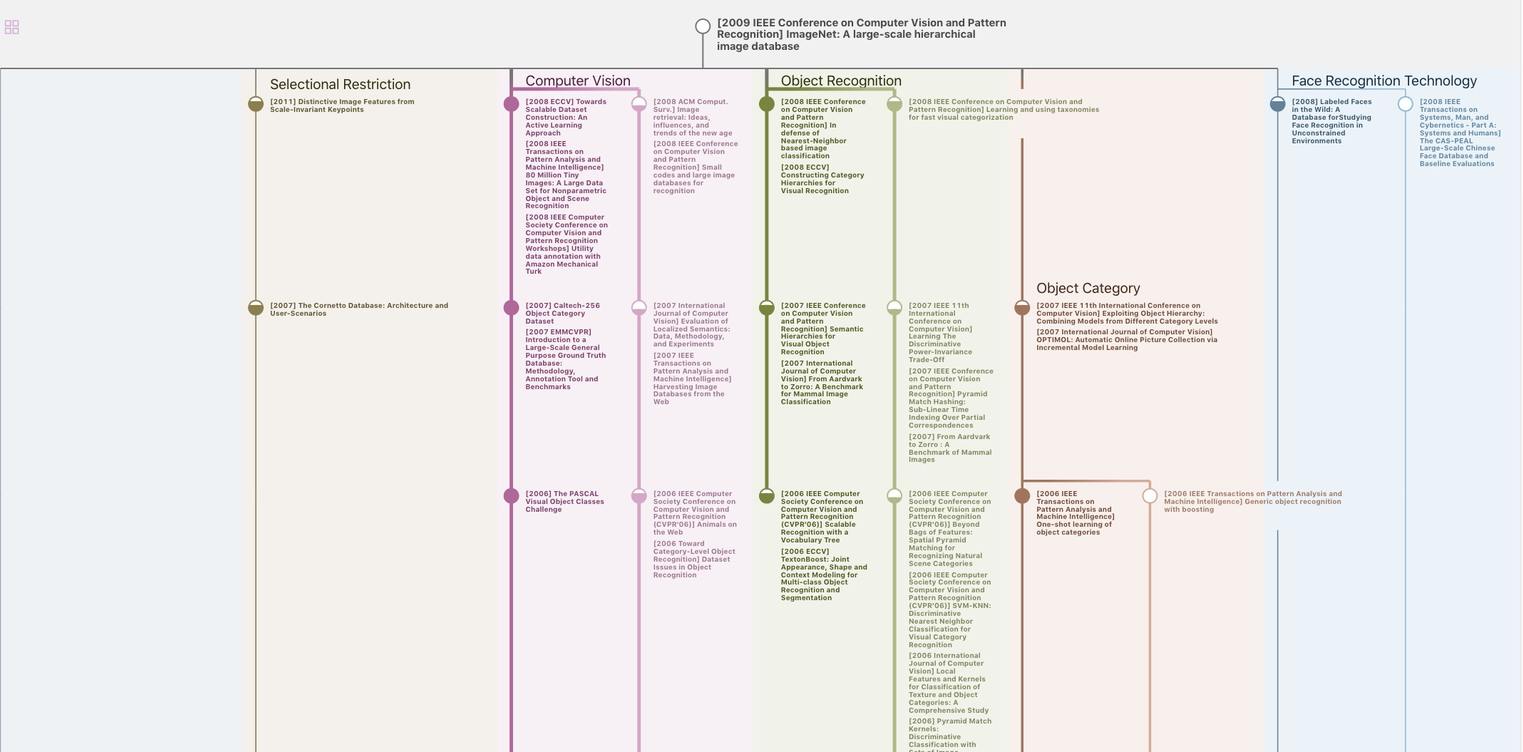
生成溯源树,研究论文发展脉络
Chat Paper
正在生成论文摘要