Supervised Machine Learning Applied to Non-Invasive EMG Signal Classification for an Anthropomorphic Robotic Hand
2022 IEEE ANDESCON(2022)
摘要
Advances in hardware development have created robotic hands able to mimic the appearance and functionality of the human counterpart (i.e., Anthropomorphic Robotic Hand (ARH)). Non-invasive signal identification and classification to control the robotic hands remains a challenge for real scenarios. Myoelectric sensors provide valuable information of muscle activity. However, the subject’s gesture identification through myoelectric signals is a complex task. In this work, we implemented a non-invasive EMG-Based control system for an ARH based on artificial neural nets. Electromyography (EMG) data were pre-processed using filtering and scaling. Then, for each subject, 20 relevant features were extracted and selected. Next, eight machine learning architectures were used for gesture identification. Finally, the control system was implemented using custom scripts in Python, communicating with a six DoF robotic hand using an ESP32 microcontrolled card. As a result of the evaluation with the classifiers, the Multi-layer Perceptron obtained the highest accuracy of 84.78%. The implemented control system was open-loop, based on multiple inputs with a single output for each servomotor, with two seconds of delay. The EMG data classification based on artificial neural networks could be an essential resource to increase the number of independent movements performed by robotic hands.
更多查看译文
关键词
EMG Data Classification,EMG Machine Learning,Anthropomorphic Robotic Hand,Artificial Neural Network
AI 理解论文
溯源树
样例
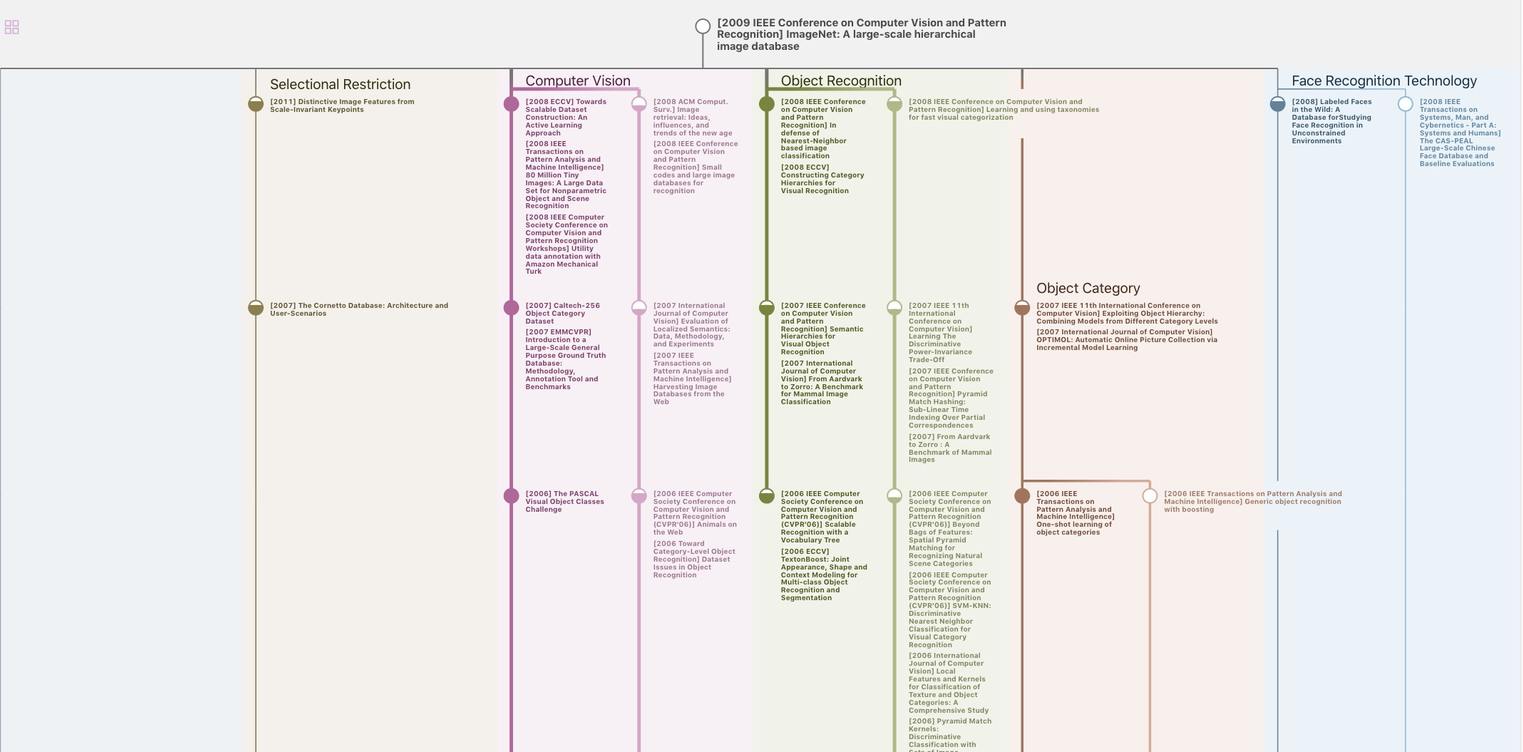
生成溯源树,研究论文发展脉络
Chat Paper
正在生成论文摘要