Wdt-SCAN: Clustering Decentralized Social Graphs with Local Differential Privacy
COMPUTERS & SECURITY(2023)
摘要
Social network users scatter geographically, where each maintains a self-centered star graph (i.e., relationships directed related to them). Clustering on such subgraphs can provide valuable insights into the graph structure. Despite its significance, one could not simply collect the unfettered star graph information from users due to privacy concerns. Local differential privacy (LDP), as an emerging privacy model, has been widely adopted in privacy-preserving data collection and analysis. Most existing LDP-based graph analysis methods encode star graphs via adjacency bit vector and suffer from heavy noise due to the sparsity of social networks. Besides, they cluster all nodes indifferently yet ignore that nodes with different degrees are affected differently by noise injection, making the clustering results unsatisfactory. To tackle these issues, we propose Wdt-SCAN, a degree vector based private graph clustering scheme to achieve high quality clustering without compromising individual privacy. Concretely, we design a degree vector encoding model with optimal length to represent star graphs, which reduces the massive noise caused by sparsity. To improve the clustering accuracy, a two-round agglomeration-based decentralized graph clustering method is proposed, which partitions nodes into core nodes and ordinary nodes, and then clusters core nodes to form a graph skeleton as prior knowledge to alleviate the impact of noise injection on ordinary nodes. Theoretical analysis and experiments on real-world datasets show that our proposed method can obtain desirable clustering result while satisfying edge LDP.
更多查看译文
关键词
Local differential privacy,Social network,Graph clustering,Data mining,Privacy and security
AI 理解论文
溯源树
样例
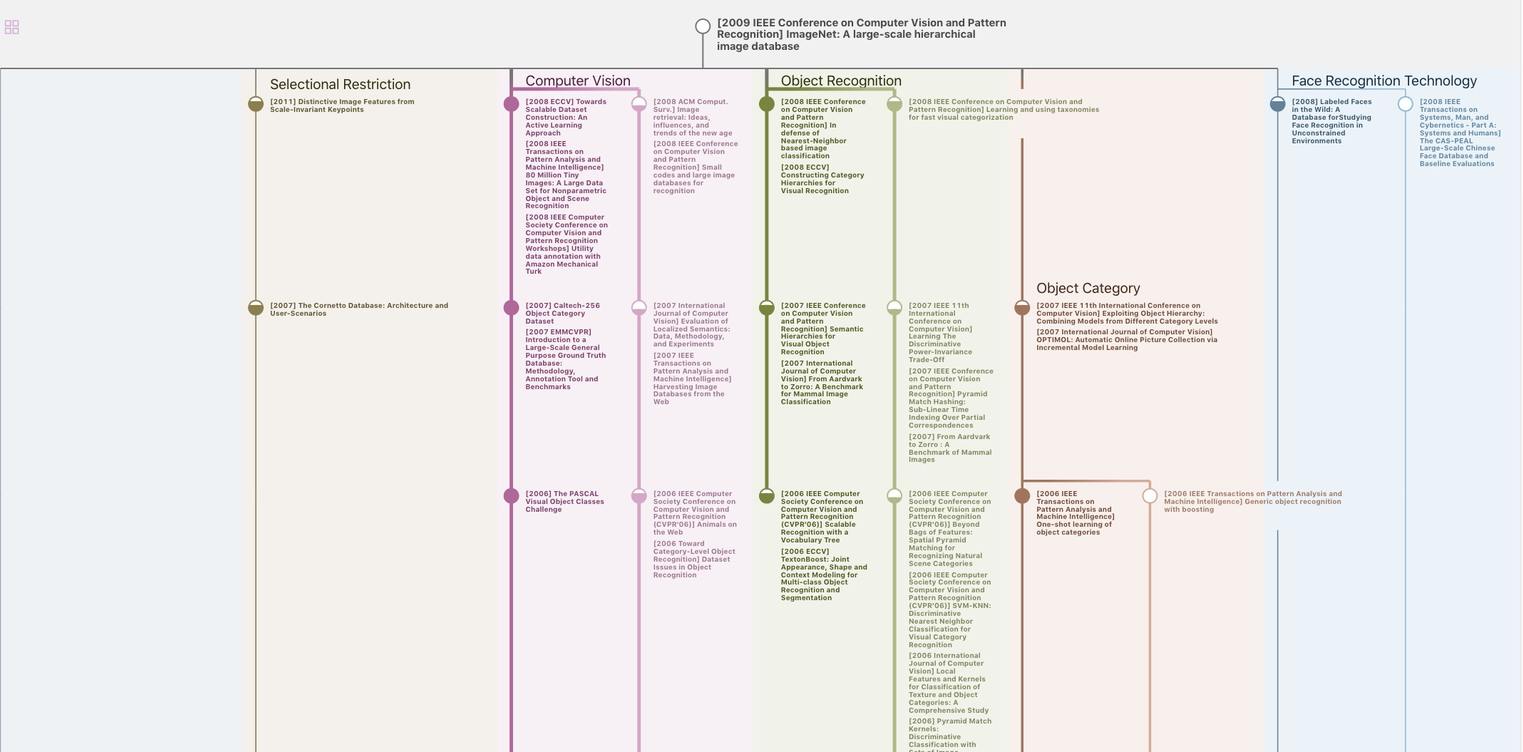
生成溯源树,研究论文发展脉络
Chat Paper
正在生成论文摘要