Granger causality test for heteroskedastic and structural-break time series using generalized least squares
arXiv (Cornell University)(2023)
Abstract
This paper proposes a novel method (GLS Granger test) to determine causal relationships between time series based on the estimation of the autocovariance matrix and generalized least squares. We show the effectiveness of proposed autocovariance matrix estimator (the sliding autocovariance matrix) and we compare the proposed method with the classical Granger F-test with via a synthetic dataset and a real dataset composed by cryptocurrencies. The simulations show that the proposed GLS Granger test captures causality more accurately than Granger F-tests in the cases of heteroskedastic or structural-break residuals. Finally, we use the proposed method to unravel unknown causal relationships between cryptocurrencies.
MoreTranslated text
Key words
structural-break
AI Read Science
Must-Reading Tree
Example
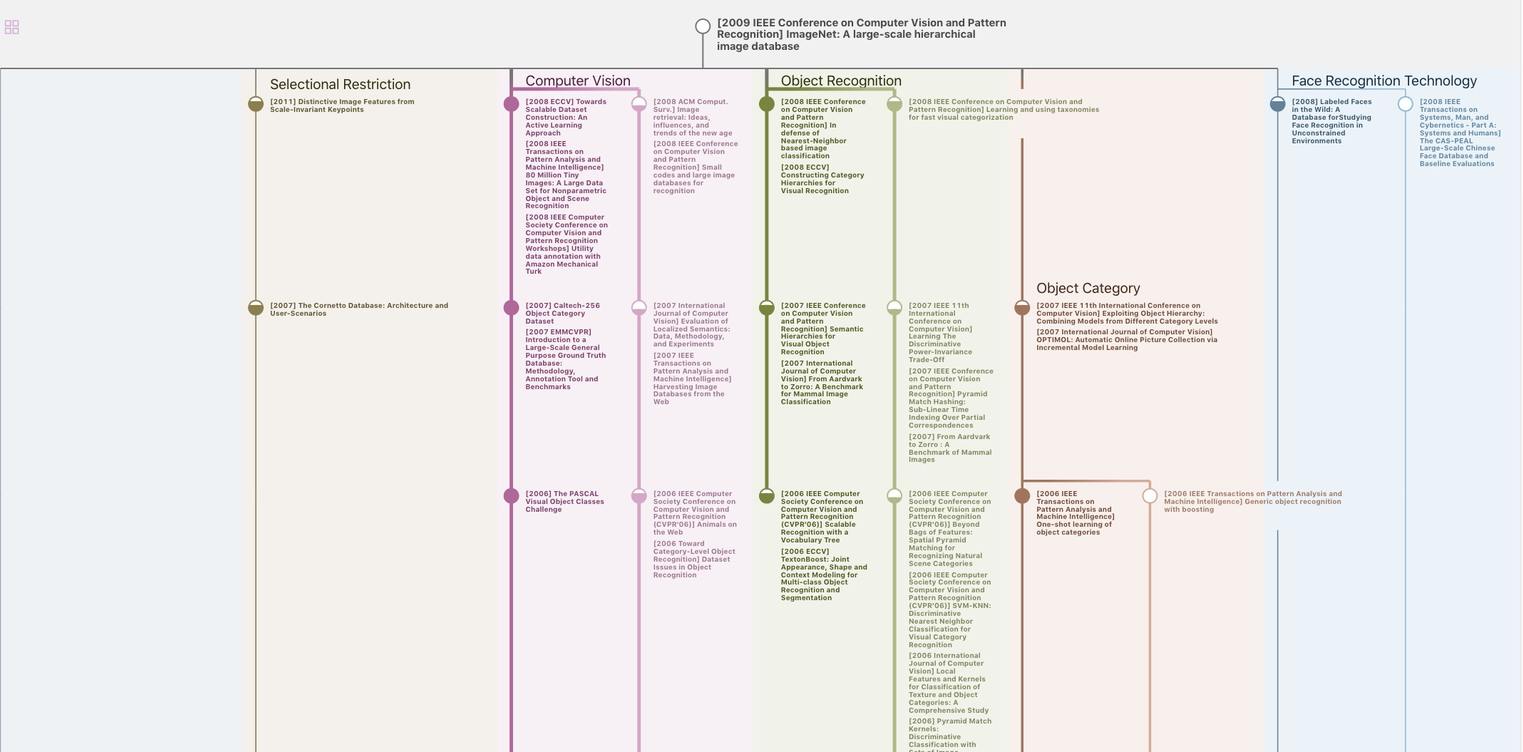
Generate MRT to find the research sequence of this paper
Chat Paper
Summary is being generated by the instructions you defined