Metapath-aggregated Heterogeneous Graph Neural Network for Drug-Target Interaction Prediction
Briefings in Bioinformatics(2023)
Nankai Univ | 38 Tongyan Rd | Nanjing Univ
Abstract
Drug-target interaction (DTI) prediction is an essential step in drug repositioning. A few graph neural network (GNN)-based methods have been proposed for DTI prediction using heterogeneous biological data. However, existing GNN-based methods only aggregate information from directly connected nodes restricted in a drug-related or a target-related network and are incapable of capturing high-order dependencies in the biological heterogeneous graph. In this paper, we propose a metapath-aggregated heterogeneous graph neural network (MHGNN) to capture complex structures and rich semantics in the biological heterogeneous graph for DTI prediction. Specifically, MHGNN enhances heterogeneous graph structure learning and high-order semantics learning by modeling high-order relations via metapaths. Additionally, MHGNN enriches high-order correlations between drug-target pairs (DTPs) by constructing a DTP correlation graph with DTPs as nodes. We conduct extensive experiments on three biological heterogeneous datasets. MHGNN favorably surpasses 17 state-of-the-art methods over 6 evaluation metrics, which verifies its efficacy for DTI prediction. The code is available at https://github.com/Zora-LM/MHGNN-DTI.
MoreTranslated text
Key words
Drug-target interaction prediction,heterogeneous graph,graph neural network,metapath
求助PDF
上传PDF
View via Publisher
AI Read Science
AI Summary
AI Summary is the key point extracted automatically understanding the full text of the paper, including the background, methods, results, conclusions, icons and other key content, so that you can get the outline of the paper at a glance.
Example
Background
Key content
Introduction
Methods
Results
Related work
Fund
Key content
- Pretraining has recently greatly promoted the development of natural language processing (NLP)
- We show that M6 outperforms the baselines in multimodal downstream tasks, and the large M6 with 10 parameters can reach a better performance
- We propose a method called M6 that is able to process information of multiple modalities and perform both single-modal and cross-modal understanding and generation
- The model is scaled to large model with 10 billion parameters with sophisticated deployment, and the 10 -parameter M6-large is the largest pretrained model in Chinese
- Experimental results show that our proposed M6 outperforms the baseline in a number of downstream tasks concerning both single modality and multiple modalities We will continue the pretraining of extremely large models by increasing data to explore the limit of its performance
Upload PDF to Generate Summary
Must-Reading Tree
Example
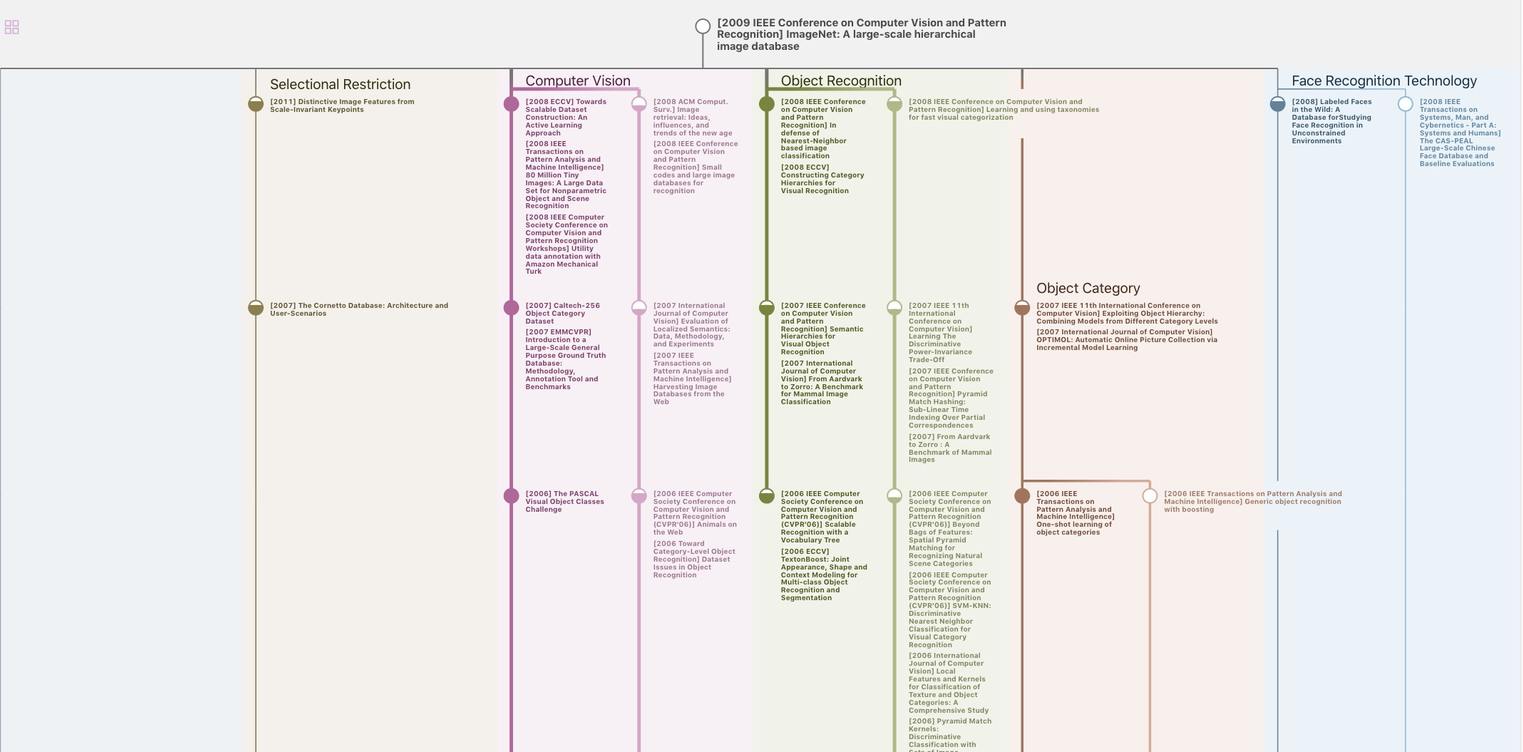
Generate MRT to find the research sequence of this paper
Related Papers
BIOINFORMATICS 2023
被引用7
Applications of Machine Learning in Microbial Natural Product Drug Discovery
EXPERT OPINION ON DRUG DISCOVERY 2023
被引用1
Drug Repositioning Based on Tripartite Cross-Network Embedding and Graph Convolutional Network
Expert Systems with Applications 2024
被引用1
FUTURE GENERATION COMPUTER SYSTEMS-THE INTERNATIONAL JOURNAL OF ESCIENCE 2024
被引用0
Expert Systems with Applications 2024
被引用0
JOURNAL OF COMPUTATIONAL BIOPHYSICS AND CHEMISTRY 2024
被引用1
Connecting the Dots: Computational Network Analysis for Disease Insight and Drug Repurposing
CURRENT OPINION IN STRUCTURAL BIOLOGY 2024
被引用0
Data Disclaimer
The page data are from open Internet sources, cooperative publishers and automatic analysis results through AI technology. We do not make any commitments and guarantees for the validity, accuracy, correctness, reliability, completeness and timeliness of the page data. If you have any questions, please contact us by email: report@aminer.cn
Chat Paper