Crack-SegNet: Surface Crack Detection in Complex Background Using Encoder-Decoder Architecture.
International Conference on Sensors, Signal and Image Processing (SSIP)(2021)
摘要
Timely and accurate detection of the initiation and expansion of crack is of great significance for improving safe operation of civil infrastructures. Image-based visual surface inspection has been an indispensable way for long-time infrastructure monitoring. However, existing crack detection methods generally suffer from the interference of complex background, leading to obvious performance drops. To tackle this, an improved encoder-decoder architecture based on SegNet is proposed in this paper, namely crack-SegNet. The encoder network hierarchically learns visual features from the original image, and the decoder network gradually up-samples and maps the encoded features to the input size for the pixel-level classification. In order to enhance the feature capacity of cracks in complex background, a channel attention mechanism is integrated into the encoder, as well as a spatial attention module in the decoder to improve the feature representation of cracks. Meanwhile, a spatial pyramid pooling is also attached to the last convolutional layer of the encoder to capture crack with different scales. To better validate the proposed method, a challenging metal surface crack dataset with much more complex background is collected. Experimental results on the datasets show that the proposed crack-SegNet outperforms other state-of-the-art crack detection methods, especially in complex background.
更多查看译文
AI 理解论文
溯源树
样例
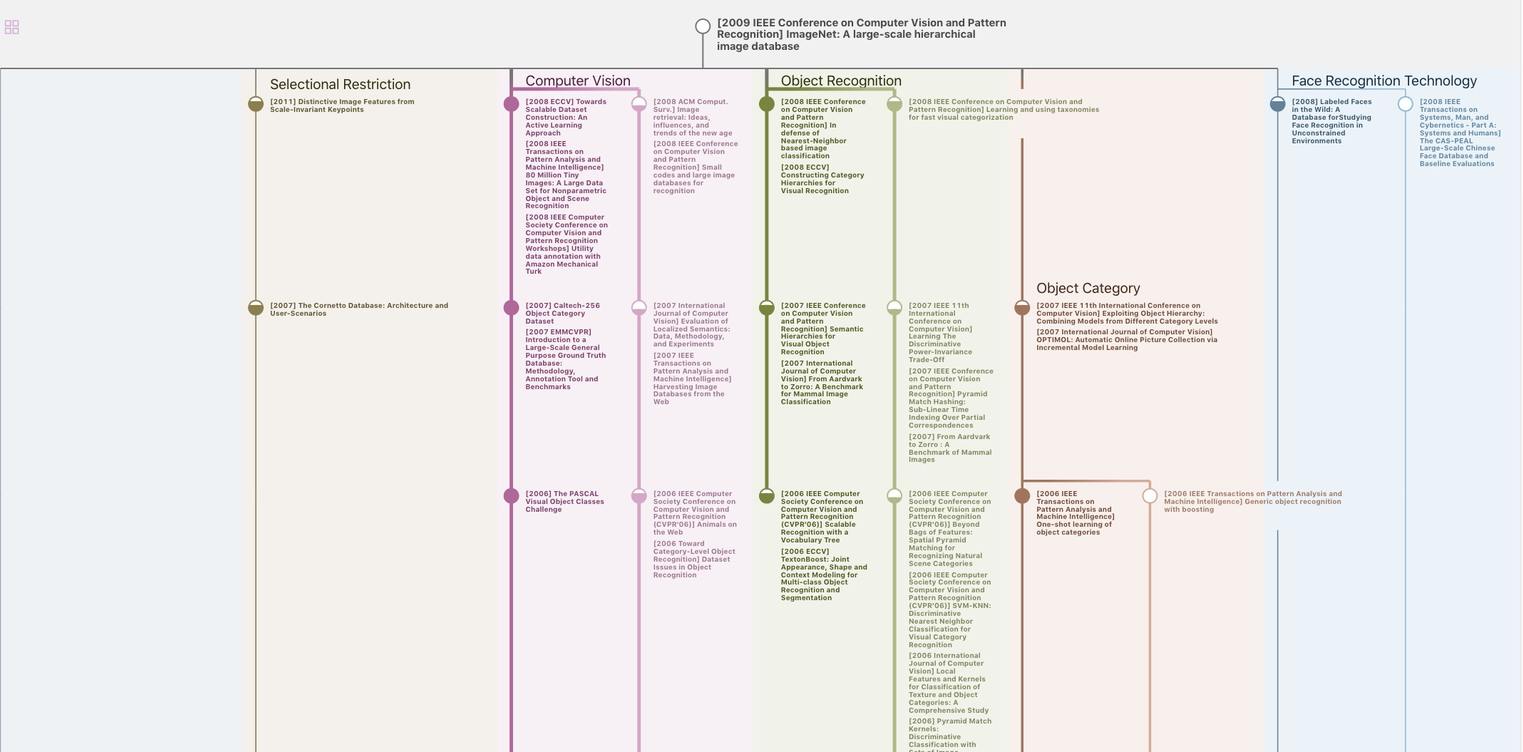
生成溯源树,研究论文发展脉络
Chat Paper
正在生成论文摘要