Cross-lingual and Cross-domain Transfer Learning for Automatic Term Extraction from Low Resource Data.
LREC 2022 THIRTEEN INTERNATIONAL CONFERENCE ON LANGUAGE RESOURCES AND EVALUATION(2022)
摘要
Automatic Term Extraction (ATE) is a key component for domain knowledge understanding and an important basis for further natural language processing applications. Even with persistent improvements, ATE still exhibits weak results exacerbated by small training data inherent to specialized domain corpora. Recently, transformers-based deep neural models, such as BERT, have proven to be efficient in many downstream NLP tasks. However, no systematic evaluation of ATE has been conducted so far. In this paper, we run an extensive study on fine-tuning pre-trained BERT models for ATE. We propose strategies that empirically show BERT's effectiveness using cross-lingual and cross-domain transfer learning to extract single and multi-word terms. Experiments have been conducted on four specialized domains in three languages. The obtained results suggest that BERT can capture cross-domain and cross-lingual terminologically-marked contexts shared by terms, opening a new design-pattern for ATE.
更多查看译文
关键词
ATE,automatic term extraction,terminology,low resource,multilingual
AI 理解论文
溯源树
样例
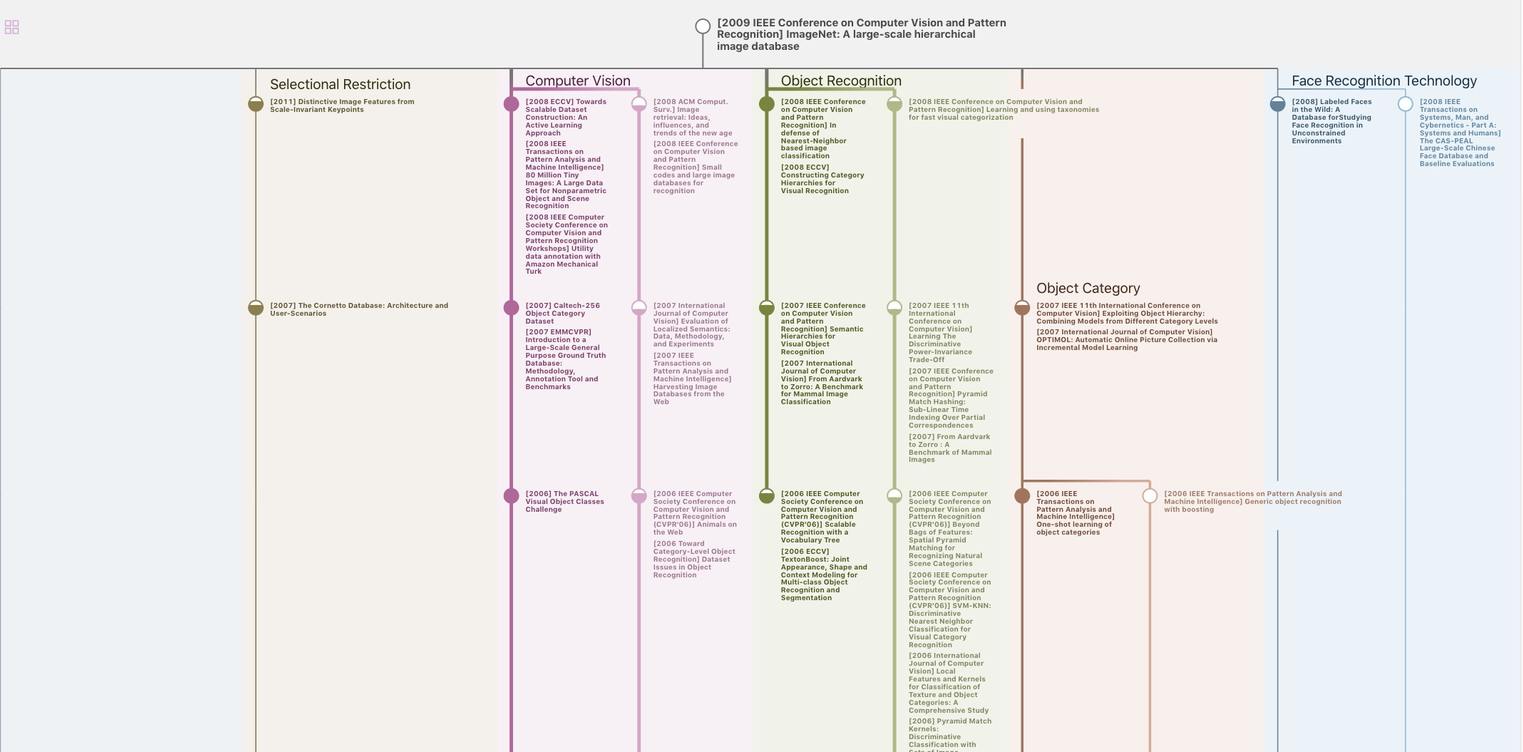
生成溯源树,研究论文发展脉络
Chat Paper
正在生成论文摘要