Multi-Modal Motion Prediction with Graphormers.
International Conference on Intelligent Transportation Systems (ITSC)(2022)
摘要
Urban road traffic is a highly dynamic environment due to its many rules, complex road layouts and road user interactions. Consequently, accurate prediction of future positions remains a challenging task. Inspired by achievements in the Natural Language Processing domain recent motion prediction models leverage the Transformer architecture. Although the models produce state-of-the-art results, they completely neglect the graph semantics inherent in the data. Graphormer is a promising architecture that was proposed recently for tackling this challenge in the field of molecule science. In this paper, we show how the Graphormer architecture can be leveraged for addressing the motion prediction task. We propose a novel encoding strategy to create a locality aware Graphormer. In addition, we extend the architecture to be able to handle edge features with the self-attention mechanism. To demonstrate the effectiveness of all components we evaluate our model on the publicly available urban motion forecasting dataset from Argoverse. Quantitative and qualitative evaluations show that our model is able to make precise state-of-the-art predictions.
更多查看译文
关键词
multimodal motion prediction,graphormers,urban road traffic,highly dynamic environment,complex road layouts,road user interactions,future positions,Natural Language Processing domain recent motion prediction models leverage,Transformer architecture,models produce state-of-the-art results,Graphormer architecture,motion prediction task,locality aware Graphormer,publicly available urban motion forecasting dataset,precise state-of-the-art predictions
AI 理解论文
溯源树
样例
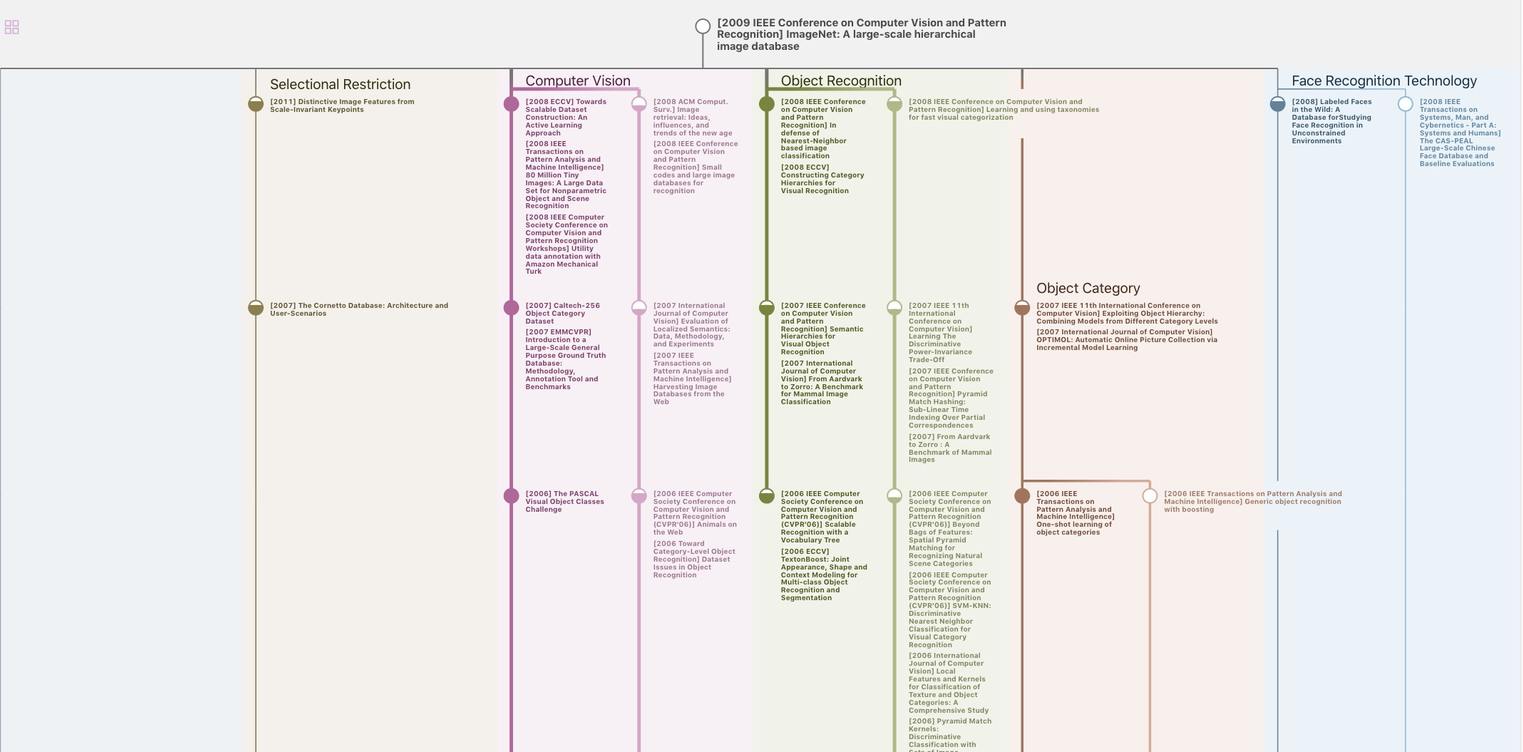
生成溯源树,研究论文发展脉络
Chat Paper
正在生成论文摘要