Adversarial VAE with Normalizing Flows for Multi-Dimensional Classification.
Chinese Conference on Pattern Recognition and Computer Vision (PRCV)(2022)
摘要
Exploiting correlations among class variables and using them to facilitate the learning process are a key challenge of Multi-Dimensional Classification (MDC) problems. Label embedding is an efficient strategy towards MDC problems. However, previous methods for MDC only use this technique as a way of feature augmentation and train a separate model for each class variable in MDC problems. Such two-stage approaches may cause unstable results and achieve suboptimal performance. In this paper, we propose an end-to-end model called Adversarial Variational AutoEncoder with Normalizing Flow (ADVAE-Flow), which encodes both features and class variables to probabilistic latent spaces. Specifically, considering the heterogeneity of class spaces, we introduce a normalizing flows module to increase the capacity of probabilistic latent spaces. Then adversarial training is adopted to help align transformed latent spaces obtained by normalizing flows. Extensive experiments on eight MDC datasets demonstrate the superiority of the proposed ADVAE-Flow model over state-of-the-art MDC models.
更多查看译文
关键词
normalizing flows,classification,multi-dimensional
AI 理解论文
溯源树
样例
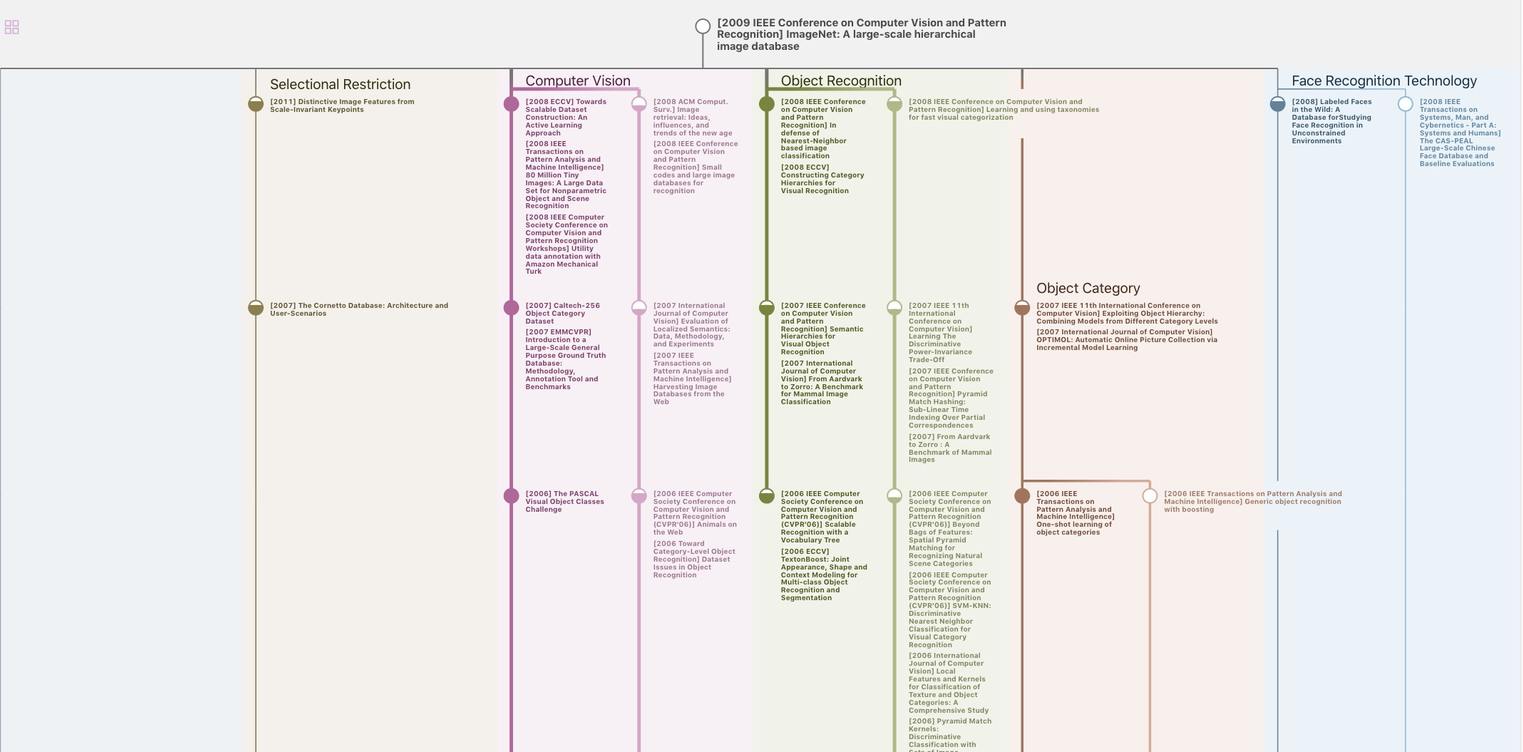
生成溯源树,研究论文发展脉络
Chat Paper
正在生成论文摘要