Making Powerful Enemies on NVIDIA GPUs
IEEE Real-Time Systems Symposium (RTSS)(2022)CCF A
Abstract
Graphics Processing Units (GPUs) are widely used in safety-critical real-time systems such as autonomous vehicles due to their high performance on artificial intelligence (AI) work-loads. As the computing power of recent GPUs keeps growing, it becomes increasingly possible to allow multiple independent programs to access the GPU concurrently. This complicates timing analysis, as contention for shared GPU resources renders execution times less predictable and worst-case execution times (WCETs) difficult to estimate. This paper provides a method for producing enemy programs that intentionally contend for GPU resources in order to enable more confident measurement-based WCET estimations. This paper provides an experiment-driven method to design effective enemy programs for several different interference channels—specific shared resources within the GPU through which concurrent computations may impact others' execution times. The method is flexible and can be applied to different GPU sharing mechanisms. The enemies are evaluated against a large number of real GPU applications, and the results indicate that these enemies cause higher slowdowns for GPU tasks than other baseline resource-stressing methods.
MoreTranslated text
Key words
real-time systems,graphics processing units,measurement-based timing analysis,interference channels
求助PDF
上传PDF
View via Publisher
AI Read Science
AI Summary
AI Summary is the key point extracted automatically understanding the full text of the paper, including the background, methods, results, conclusions, icons and other key content, so that you can get the outline of the paper at a glance.
Example
Background
Key content
Introduction
Methods
Results
Related work
Fund
Key content
- Pretraining has recently greatly promoted the development of natural language processing (NLP)
- We show that M6 outperforms the baselines in multimodal downstream tasks, and the large M6 with 10 parameters can reach a better performance
- We propose a method called M6 that is able to process information of multiple modalities and perform both single-modal and cross-modal understanding and generation
- The model is scaled to large model with 10 billion parameters with sophisticated deployment, and the 10 -parameter M6-large is the largest pretrained model in Chinese
- Experimental results show that our proposed M6 outperforms the baseline in a number of downstream tasks concerning both single modality and multiple modalities We will continue the pretraining of extremely large models by increasing data to explore the limit of its performance
Upload PDF to Generate Summary
Must-Reading Tree
Example
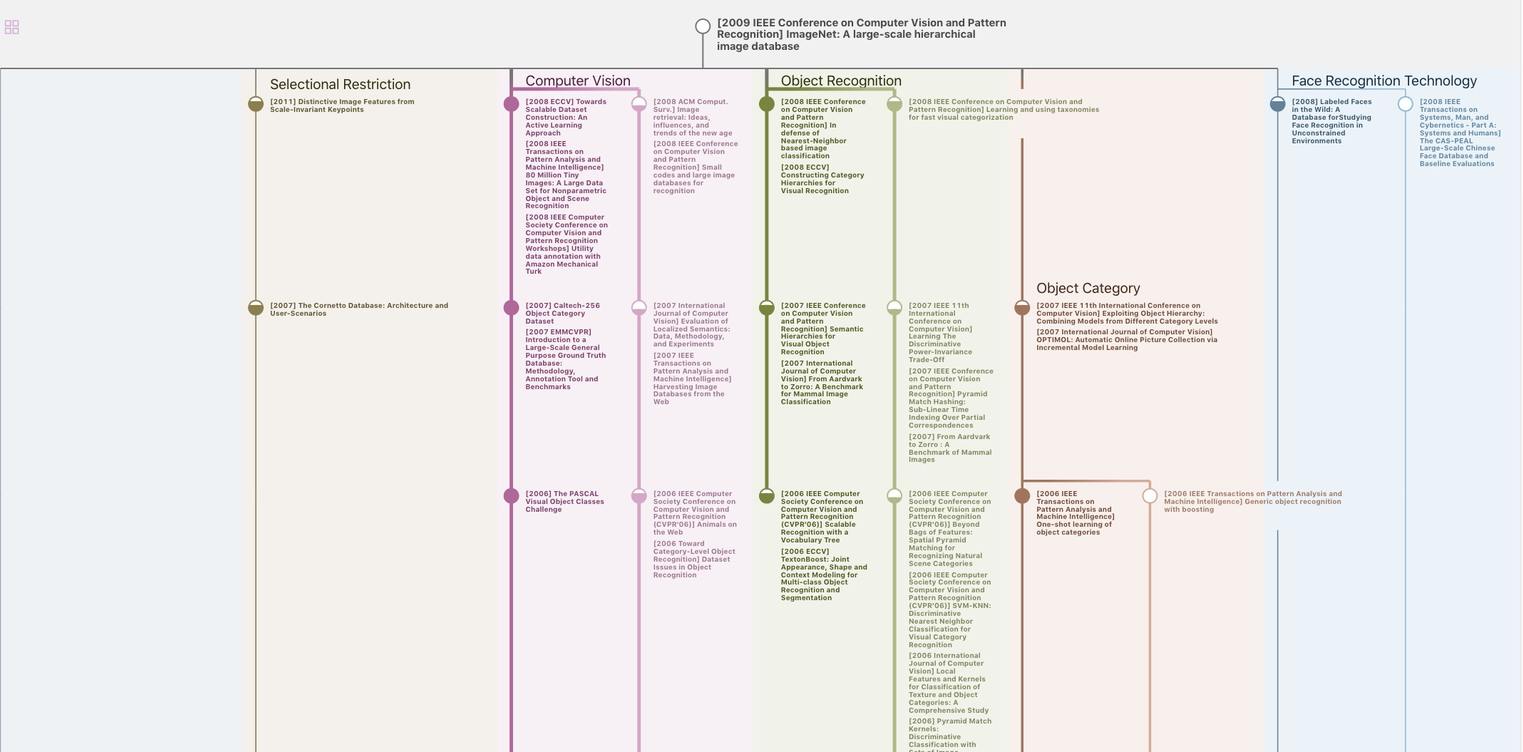
Generate MRT to find the research sequence of this paper
Related Papers
IEEE TRANSACTIONS ON COMPUTERS 2024
被引用0
Hardware Compute Partitioning on NVIDIA GPUs.
2023 IEEE 29TH REAL-TIME AND EMBEDDED TECHNOLOGY AND APPLICATIONS SYMPOSIUM, RTAS 2023
被引用1
JOB SCHEDULING STRATEGIES FOR PARALLEL PROCESSING, JSSPP 2023 2023
被引用0
REVISTA IBEROAMERICANA DE AUTOMATICA E INFORMATICA INDUSTRIAL 2024
被引用0
Data Disclaimer
The page data are from open Internet sources, cooperative publishers and automatic analysis results through AI technology. We do not make any commitments and guarantees for the validity, accuracy, correctness, reliability, completeness and timeliness of the page data. If you have any questions, please contact us by email: report@aminer.cn
Chat Paper