Automatic Damage Segmentation Framework for Buried Sewer Pipes Based on Machine Vision: Case Study of Sewer Pipes in Zhengzhou, China
Journal of infrastructure systems(2023)
摘要
Aging buried sewer pipes are at risk of rupture, corrosion, scaling, and other damages, and they may cause urban waterlogging, road collapse, environmental pollution, and other urban safety problems. Therefore, it is very important to accurately detect and segment sewer pipe damages regularly for safety evaluation. This paper proposes an intelligent damage detection method to segment and measure five types of sewer pipe damages, namely, staggered joints, deposits, corrosion, scaling, and fracture, leveraging a fine-tuned fully convolutional network (FCN) algorithm. A case study on Zhengzhou's sewer pipes is conducted. Then, a dataset of 3,558 images is built, and the images are collected from Zhengzhou's sewer pipes. Subsequently, the encoder of the FCN is replaced with a pretrained VGG-19, and the upsampling part has spatial and channel squeeze and excitation (SCSE) modules added. The evaluation matrix of the pixel accuracy (PA), mean pixel accuracy (MPA), mean intersection over union (MIoU), and frequency weighted intersection over union (FWIoU) of the proposed method is 91.42%, 81.44%, 73.65%, and 87.16%, respectively. Subsequently, damage areas are measured at a pixel level to quantify the pipe damage. Compared with the existing mask region-based convolutional neural networks (RCNN) method, the proposed FCN-based method shows effective performance for multiple and similar sewer pipe damage detection under complex backgrounds. Moreover, a real-time damage detection platform for sewer pipes is proposed, realizing the data uploading, data storage, data analysis, and data visualization.
更多查看译文
关键词
Damage detection,Sewer pipes,Segmentation and measurement,Machine vision,Deep learning
AI 理解论文
溯源树
样例
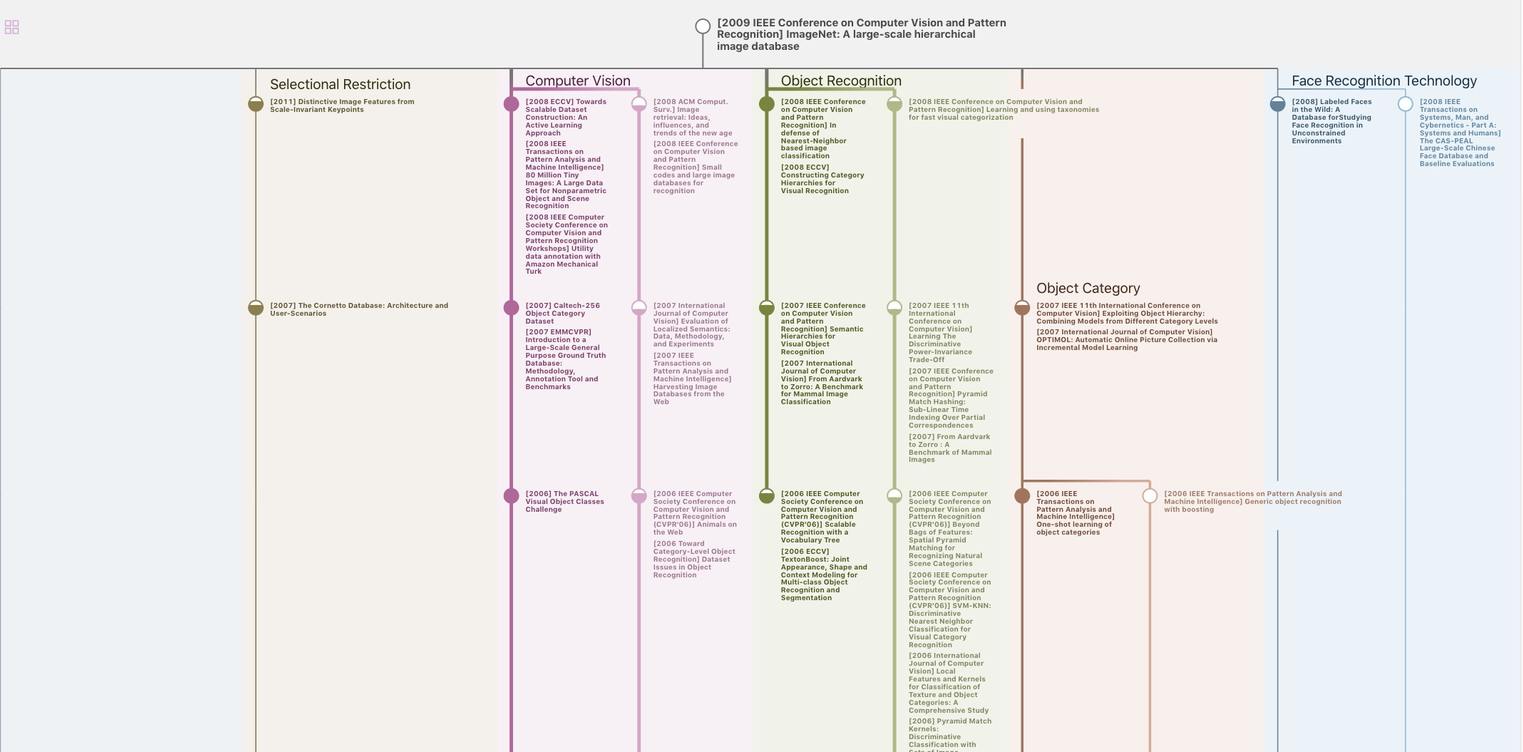
生成溯源树,研究论文发展脉络
Chat Paper
正在生成论文摘要