Unsupervised Representation Learning from Pre-trained Diffusion Probabilistic Models
NeurIPS 2022(2022)
摘要
Diffusion Probabilistic Models (DPMs) have shown a powerful capacity of generating high-quality image samples. Recently, diffusion autoencoders (Diff-AE) are proposed to explore DPMs for representation learning via autoencoding and succeed in various downstream tasks. Their key idea is to jointly train an encoder for discovering meaningful representations from images and a conditional DPM as the decoder for reconstructing images. Considering that training DPMs from scratch will take a long time and there have existed numerous pre-trained DPMs, we propose \textbf{P}re-trained \textbf{D}PM \textbf{A}uto\textbf{E}ncoding (\textbf{PDAE}), a general method to adapt existing pre-trained DPMs to the decoders for image reconstruction, with better training efficiency and performance than Diff-AE. Specifically, we find that the reanson that pre-trained DPMs fail to reconstruct an image from its latent variables is due to the information loss of forward process, which causes a gap between their predicted posterior mean and the true one. From this perspective, the classifier-guided sampling method can be explained as computing an extra mean shift to fill the gap, reconstructing the lost class information in samples. These imply that the gap corresponds to the lost information of the image, and we can reconstruct the image by filling the gap. Drawing inspiration from this, we employ a trainable model to predict a mean shift according to encoded representation and train it to fill as much gap as possible, in this way, the encoder is forced to learn as much information as possible from images to help the filling. By resuing a part of network of pre-trained DPMs and redesigning the weighting scheme of diffusion loss, PDAE can learn meaningful representations from images efficiently. Extensive experiments denonstrate the effectiveness, efficiency and flexibility of PDAE.
更多查看译文
关键词
Diffusion Probabilistic Models,Representation Learning,Autoencoders
AI 理解论文
溯源树
样例
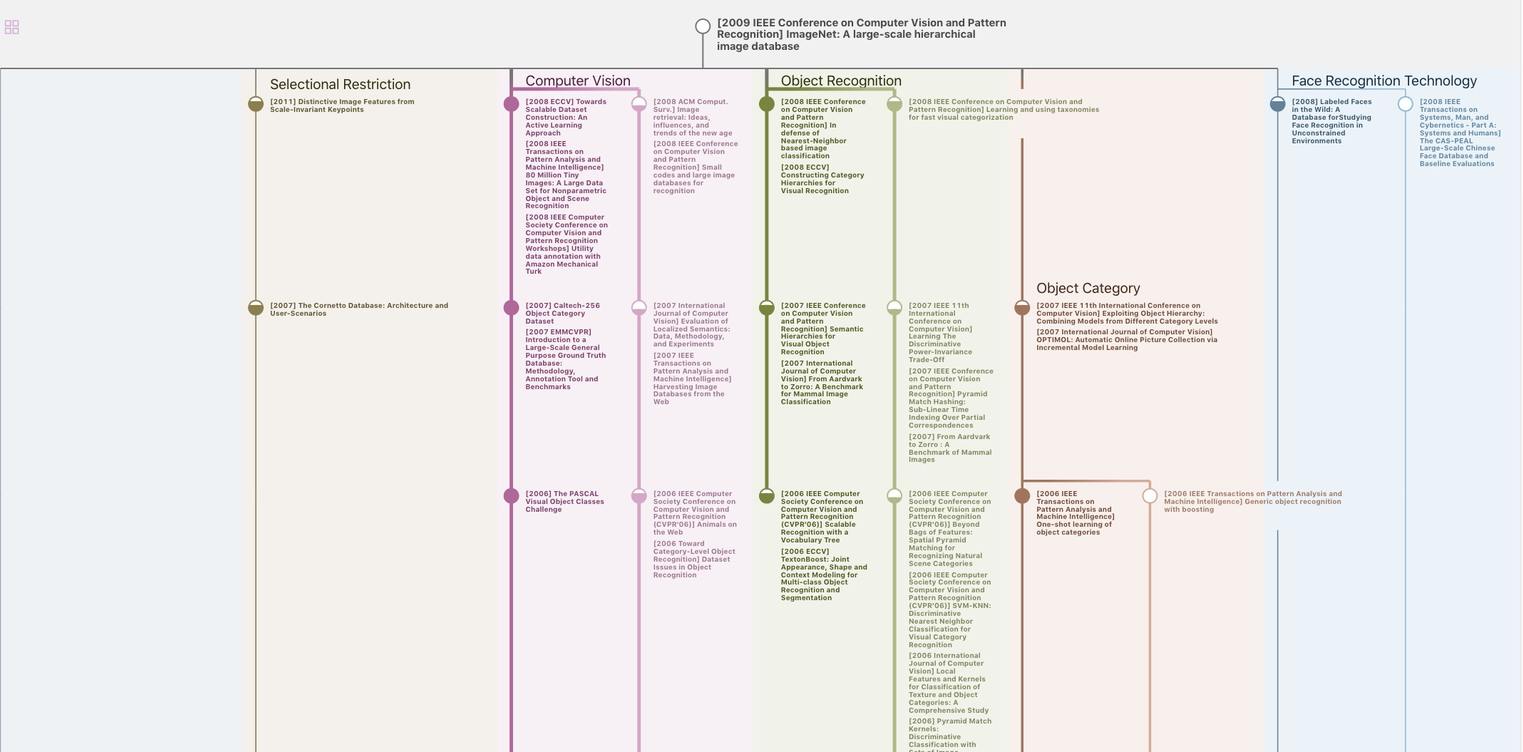
生成溯源树,研究论文发展脉络
Chat Paper
正在生成论文摘要