Semantically-informed Hierarchical Event Modeling
SEMACL(2022)
摘要
Prior work has shown that coupling sequential latent variable models with semantic ontological knowledge can improve the representational capabilities of event modeling approaches. In this work, we present a novel, doubly hierarchical, semi-supervised event modeling framework that provides structural hierarchy while also accounting for ontological hierarchy. Our approach consists of multiple layers of structured latent variables, where each successive layer compresses and abstracts the previous layers. We guide this compression through the injection of structured ontological knowledge that is defined at the type level of events: importantly, our model allows for partial injection of semantic knowledge and it does not depend on observing instances at any particular level of the semantic ontology. Across two different datasets and four different evaluation metrics, we demonstrate that our approach is able to out-perform the previous state-of-the-art approaches, demonstrating the benefits of structured and semantic hierarchical knowledge for event modeling.
更多查看译文
关键词
modeling,semantically-informed
AI 理解论文
溯源树
样例
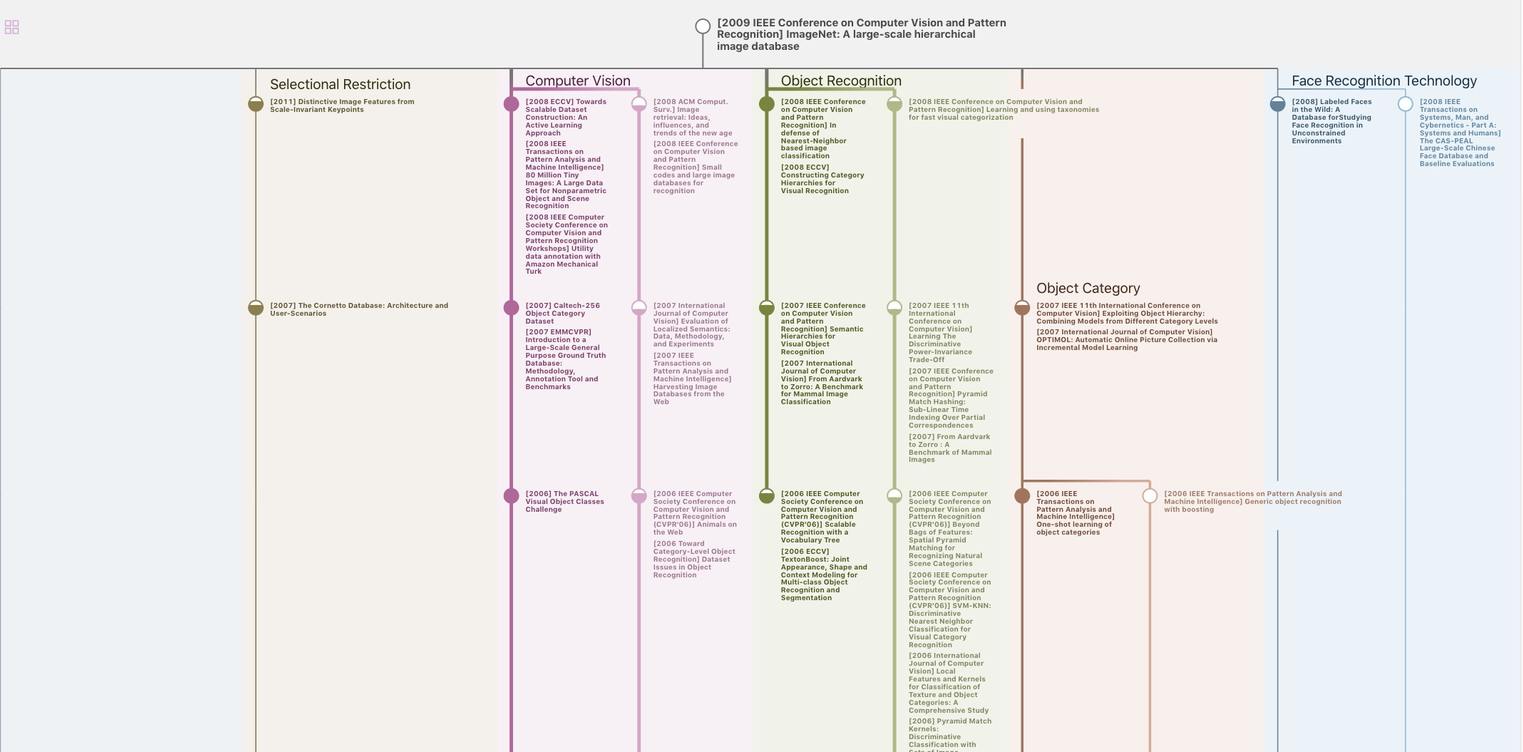
生成溯源树,研究论文发展脉络
Chat Paper
正在生成论文摘要