A Deep Learning Approach for Distributed QoS Prediction in Beyond 5G Networks
2022 IEEE 33rd Annual International Symposium on Personal, Indoor and Mobile Radio Communications (PIMRC)(2022)
摘要
Beyond 5G networks bring a new era in system automation, by introducing new and demanding, in terms of Quality of Service (QoS), use cases and applications. Predicting the QoS for end users in a timely manner and enabling service adaptation methods to react in advance in case of QoS degradation is of high importance, especially for safety-critical applications such as in vehicular communications. Current state-of-the-art approaches propose solutions towards the identification of potential QoS deterioration in a centralized manner. However, centralized solutions may raise privacy issues, since sensitive user information may need to be transmitted to communication network entities for processing and analysis. Other practical limitations of centralized solutions may also arise, such as the computational bottleneck and the fast increase of signaling overhead with number of end users. This study proposes a distributed QoS prediction scheme based on the well-known Long Short-Term Memory (LSTM) architecture to account for the natural high correlation of samples closely located in time. The primary target of the proposed scheme is to provide accurate QoS predictions up to several seconds, while preserving data privacy and reducing signaling overheads related to the exchange of information between the involved nodes. The evaluation of the proposed scheme indicates its potential gains and effectiveness compared to centralized state-of-the-art OoS prediction solutions.
更多查看译文
关键词
Beyond 5G,6G,QoS Prediction,Distributed Prediction,Machine Learning
AI 理解论文
溯源树
样例
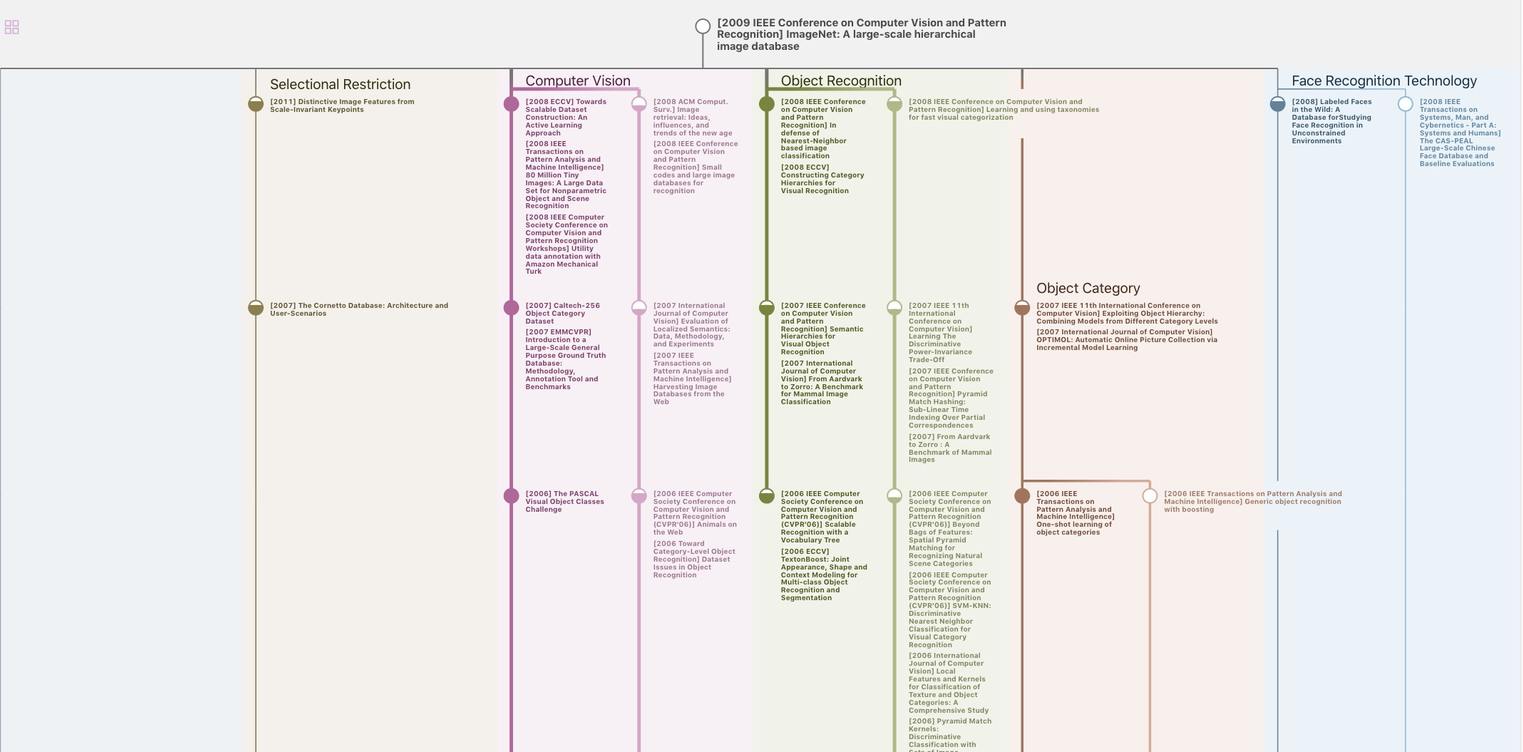
生成溯源树,研究论文发展脉络
Chat Paper
正在生成论文摘要