Real-time Human Action Recognition Using Raw Depth Video-Based Recurrent Neural Networks
Multimedia tools and applications(2022)
摘要
This work proposes and compare two different approaches for real-time human action recognition (HAR) from raw depth video sequences. Both proposals are based on the convolutional long short-term memory unit, namely ConvLSTM, with differences in the architecture and the long-term learning. The former uses a video-length adaptive input data generator ( stateless ) whereas the latter explores the stateful ability of general recurrent neural networks but is applied in the particular case of HAR. This stateful property allows the model to accumulate discriminative patterns from previous frames without compromising computer memory. Furthermore, since the proposal uses only depth information, HAR is carried out preserving the privacy of people in the scene, since their identities can not be recognized. Both neural networks have been trained and tested using the large-scale NTU RGB+D dataset. Experimental results show that the proposed models achieve competitive recognition accuracies with lower computational cost compared with state-of-the-art methods and prove that, in the particular case of videos, the rarely-used stateful mode of recurrent neural networks significantly improves the accuracy obtained with the standard mode. The recognition accuracies obtained are 75.26% (CS) and 75.45% (CV) for the stateless model, with an average time consumption per video of 0.21 s, and 80.43% (CS) and 79.91%(CV) with 0.89 s for the stateful one.
更多查看译文
关键词
ConvLSTM,Action recognition,Depth maps,Video-surveillance
AI 理解论文
溯源树
样例
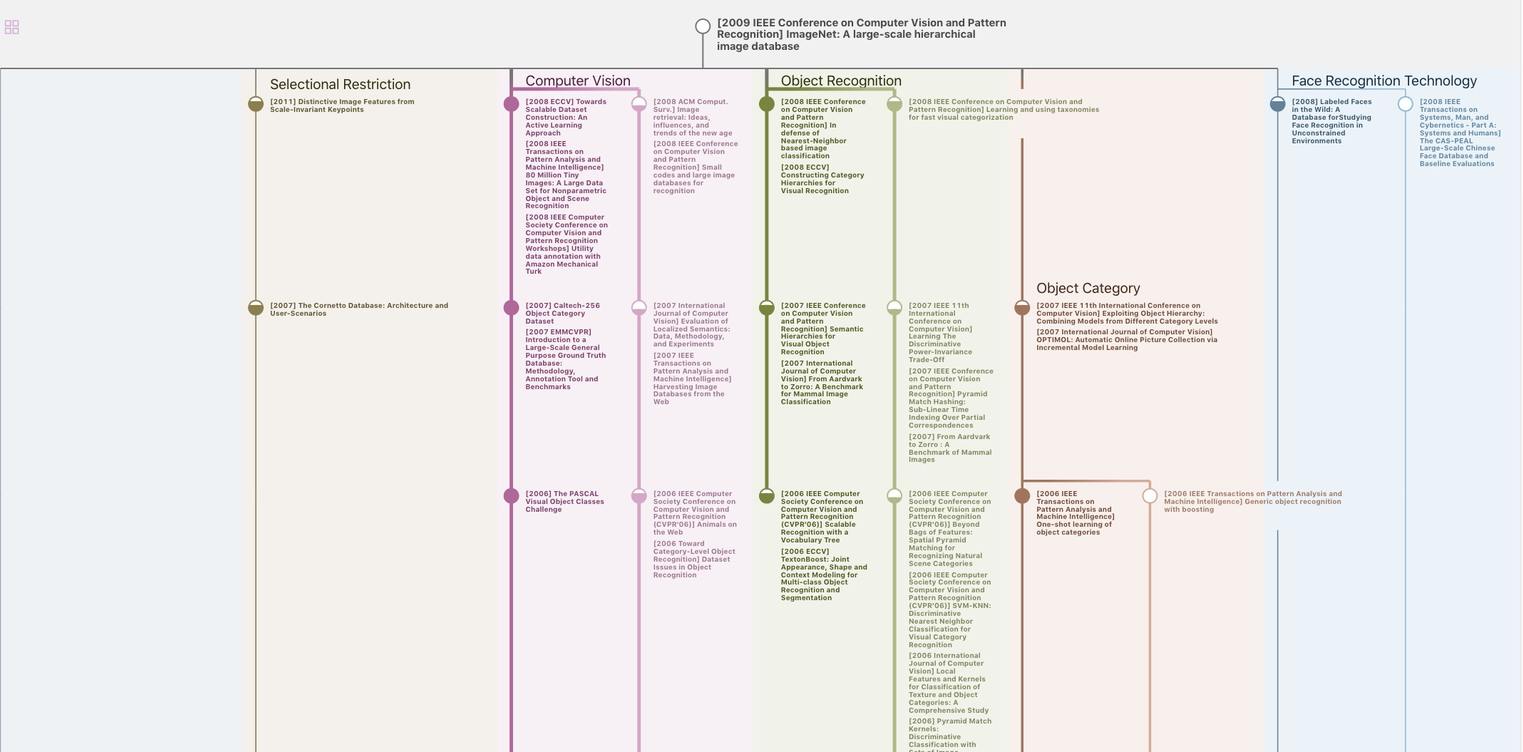
生成溯源树,研究论文发展脉络
Chat Paper
正在生成论文摘要