An Effective Industrial Defect Classification Method under the Few-Shot Setting Via Two-Stream Training
Optics and lasers in engineering(2023)
摘要
Visual surface defect inspection provides an important tool for product quality assessment in a wide range of industrial applications. In recent years, diverse convolutional neural network (CNN) models have been devel-oped for the high-accuracy image classification of natural objects. However, it remains a challenging task to develop deep-learning-based approaches for surface defect inspection of industrial products. The main challenge is that surface defects of industrial products occur in low probability, therefore it is impractical to build a large image dataset containing different types of defects to train/fine-tune deep CNN models to achieve satisfactory performance when applied to industrial inspection tasks. To overcome the above-mentioned problem, we build a light-weight defect classification model based on the pre-trained SqueezeNet architecture and present three effective techniques to achieve high-accuracy defect classification using very few collected defective training samples. First, we experimentally demonstrate that it is reasonable to freeze shallower convolutional layers and only fine-tune deeper layers in the last convolutional stage when there are not enough training samples. Second, we integrate batch-size independent Group Normalization (GN) into SqueezeNet to compute stable statistics based on a limited number of defective samples for training the classification model. Third, we add an auxiliary task to perform the similarity comparison of two defective samples during the training phase, significantly increasing the size of the training data and generating more distinctive defect-specific representations. The experimental results on the NEU-CLS dataset and USB-FS dataset verify the effectiveness of the proposed method. Our method can obtain accurate defect classification results when very few defective training samples are provided, i.e., about 97.69% and 82.92% average classification accuracy on the NEU-CLS and USB-FS datasets, respectively, under the 5-shot setting (5 images per defect category).
更多查看译文
关键词
Surface defect classification,SqueezeNet,Transfer learning,Group normalization,Two-stream training
AI 理解论文
溯源树
样例
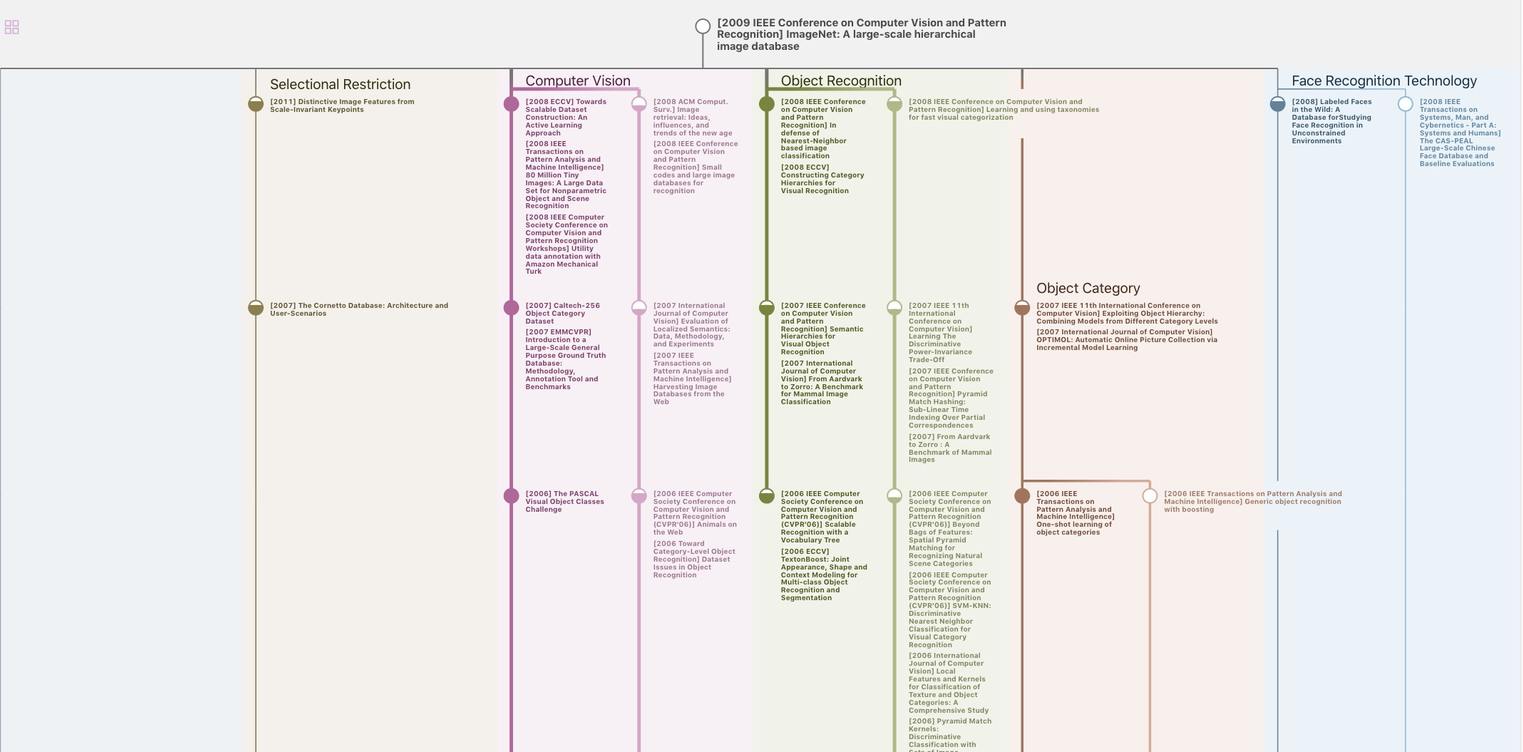
生成溯源树,研究论文发展脉络
Chat Paper
正在生成论文摘要