Experimental and Neural Networks Analysis on Elevated-Temperature Mechanical Properties of Structural Steels
Materials today communications(2022)
关键词
Neural network,Prediction,Structural steel,Elevated temperature,Mechanical property
AI 理解论文
溯源树
样例
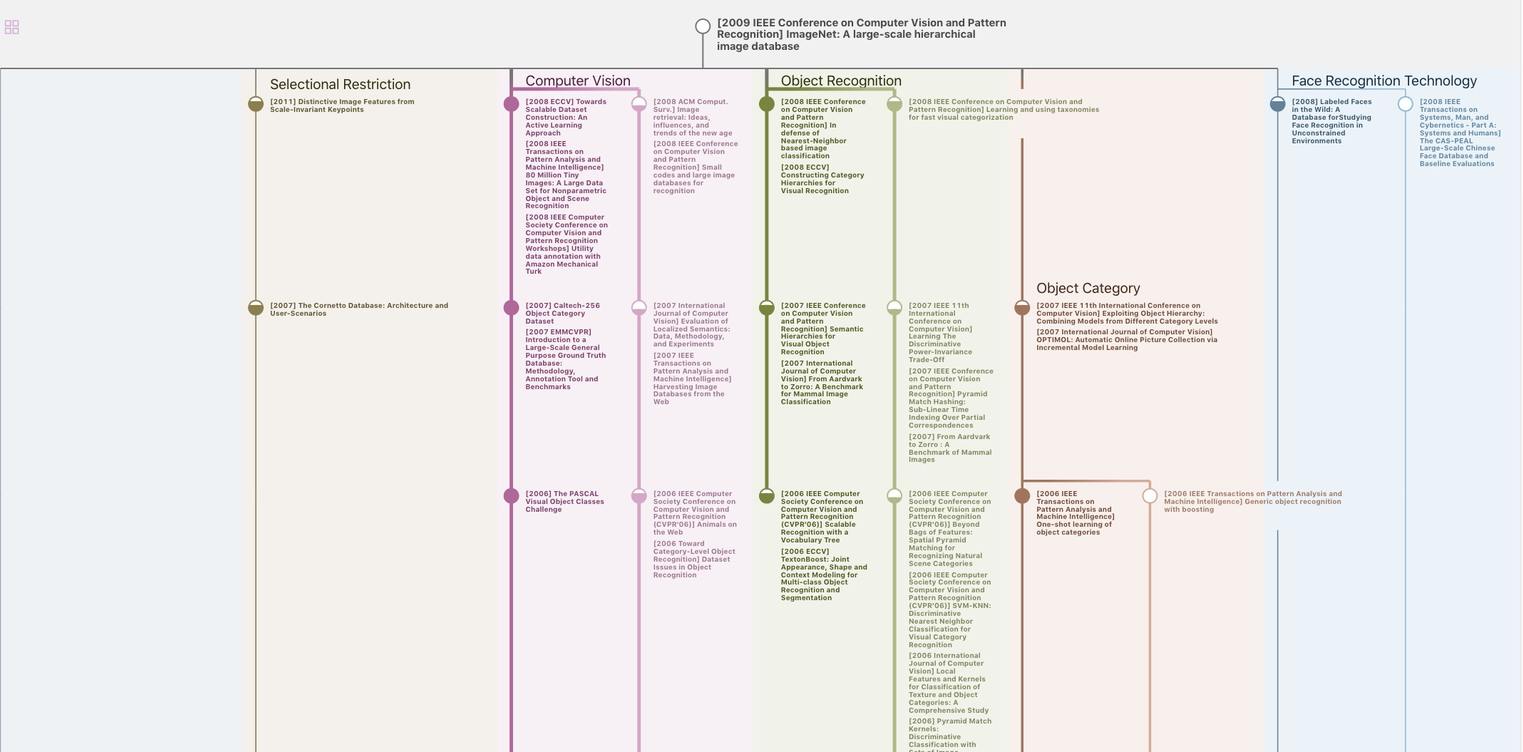
生成溯源树,研究论文发展脉络
Chat Paper
正在生成论文摘要