Dynamic Feature Selection Combining Standard Deviation and Interaction Information
International Journal of Machine Learning and Cybernetics(2022)
摘要
Feature selection achieves dimensionality reduction by selecting some effective features from the original feature set. However, in the process of feature selection, most conventional methods do not accurately describe various correlations between features and the dynamic changes of the relation, leading to an incomplete definition of the evaluation function and affecting the classification accuracy. In this study, a dynamic feature selection method combining standard deviation and interaction information (DFS-SDII) is proposed. In DFS-SDII, conditional mutual information is introduced to measure the changes in the importance of the selected features for classification. Then, the interaction information is used to measure the synergy between the candidate and selected features. In addition, candidate features with higher importance to the class are selected by standard deviation under the condition of the same score. To evaluate the performance of DFS-SDII, nine state-of-the-art feature selection methods are selected for comparison on 16 benchmark data sets based on the classification accuracy and F-measure. The experimental results show that the proposed method performs better in terms of feature selection and has a higher classification accuracy.
更多查看译文
关键词
Feature selection,Classification,Information theory,Feature interaction
AI 理解论文
溯源树
样例
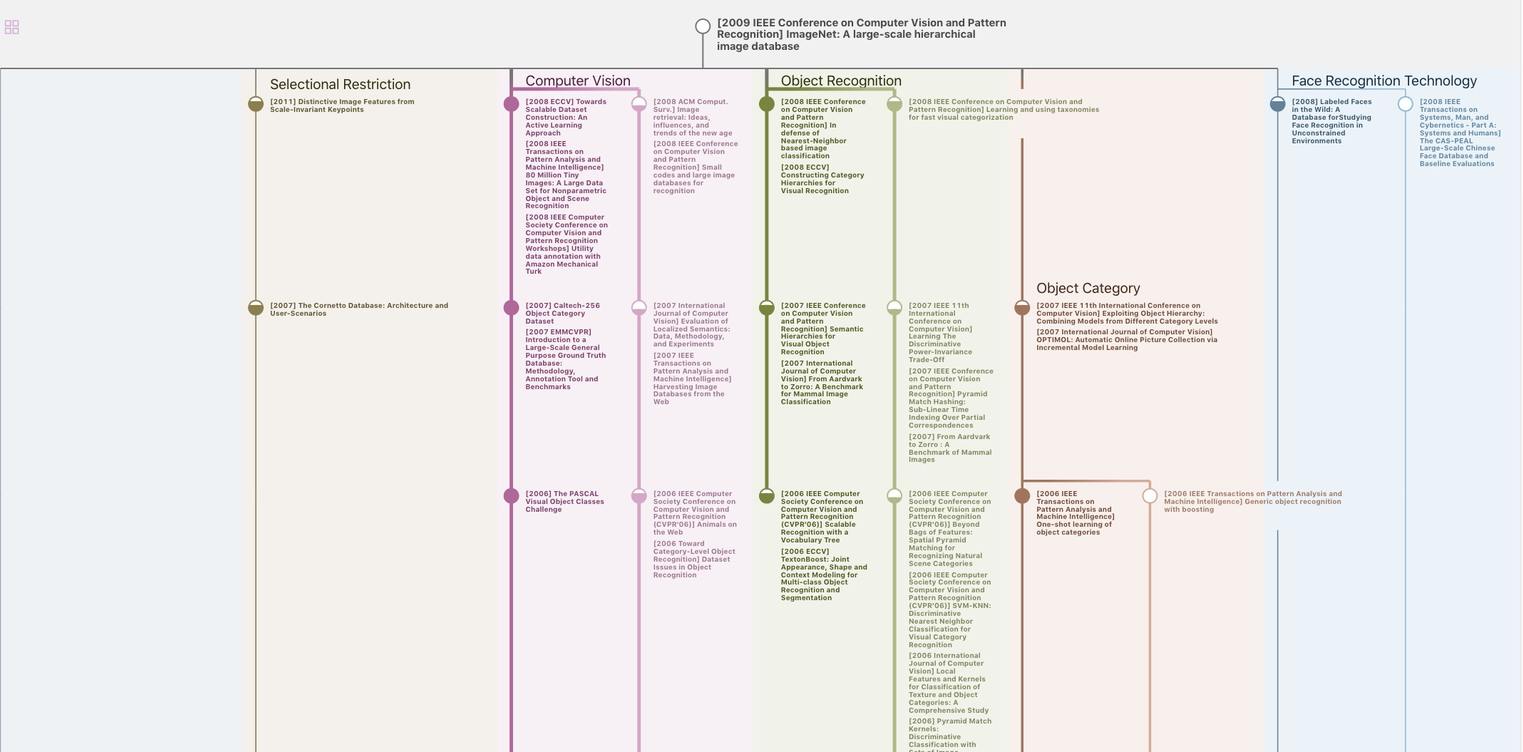
生成溯源树,研究论文发展脉络
Chat Paper
正在生成论文摘要