BKinD-3D: Self-Supervised 3D Keypoint Discovery from Multi-View Videos.
2023 IEEE/CVF Conference on Computer Vision and Pattern Recognition (CVPR)(2023)
摘要
Quantifying motion in 3D is important for studying the behavior of humans and other animals, but manual pose annotations are expensive and time-consuming to obtain. Self-supervised keypoint discovery is a promising strategy for estimating 3D poses without annotations. However, current keypoint discovery approaches commonly process single 2D views and do not operate in the 3D space. We propose a new method to perform self-supervised keypoint discovery in 3D from multi-view videos of behaving agents, without any keypoint or bounding box supervision in 2D or 3D. Our method, BKinD-3D, uses an encoder-decoder architecture with a 3D volumetric heatmap, trained to reconstruct spatiotemporal differences across multiple views, in addition to joint length constraints on a learned 3D skeleton of the subject. In this way, we discover keypoints without requiring manual supervision in videos of humans and rats, demonstrating the potential of 3D keypoint discovery for studying behavior.
更多查看译文
关键词
3D Human Pose,Unsupervised Learning,Motion Capture,Human Motion Data,Action Recognition
AI 理解论文
溯源树
样例
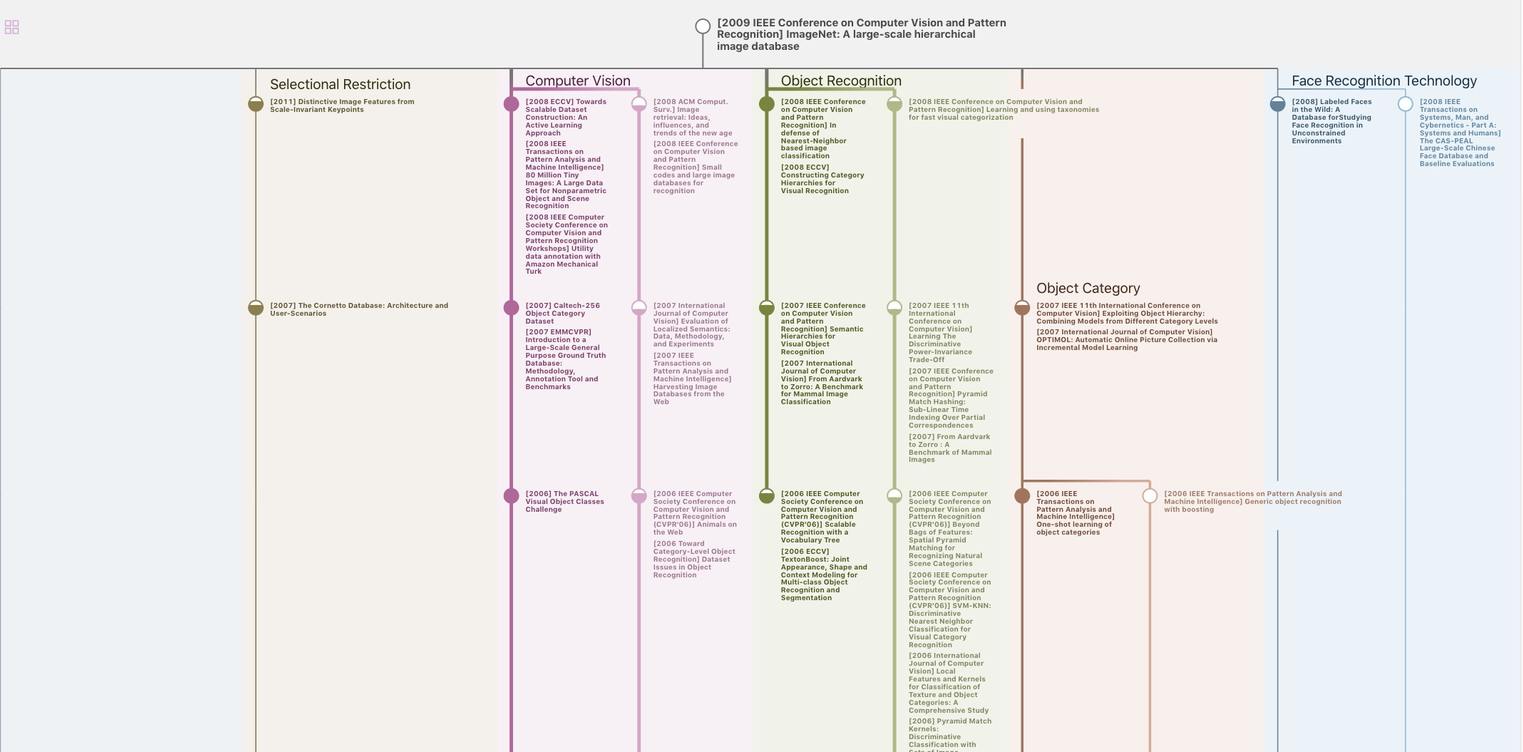
生成溯源树,研究论文发展脉络
Chat Paper
正在生成论文摘要