A clustering differential evolution algorithm with neighborhood-based dual mutation operator for multimodal multiobjective optimization
Expert Systems with Applications(2022)
Abstract
Multimodal multiobjective optimization problems (MMOPs) possess multiple equivalent Pareto optimal sets (PSs) corresponding to the same Pareto front (PF). Numerous multimodal multiobjective evolutionary algorithms (MMEAs) have been developed to solve MMOPs. However, how to locate multiple high quality equivalent PSs and obtain a uniformly distributed PF simultaneously remains a challenging task. This paper explores a novel multimodal multiobjective differential evolution algorithm, termed MMO_CDE, for solving MMOPs. In MMO_CDE, a neighborhood-based dual mutation strategy is proposed by developing two novel mutation operators. The two novel mutation operators provide a better tradeoff between exploration and exploitation in locating multiple equivalent PSs. In addition, a clustering-based environmental selection mechanism is designed by integrating an affinity propagation method. The affinity propagation method improves the distribution of solutions in decision and objective spaces, and a harmonic averaged distance is adopted to promote the diversity of a population. The proposed MMO_CDE is compared against state-of-the-art MMEAs on a test suite of CEC 2019 benchmark functions and a map-based practical application. Empirical results validate the superiority of the proposed MMO_CDE regarding locating multiple equivalent PSs and obtaining a well-distributed PF.
MoreTranslated text
Key words
Differential evolution,Multimodal multiobjective optimization problems,Multimodal multiobjective evolutionary algorithms
AI Read Science
Must-Reading Tree
Example
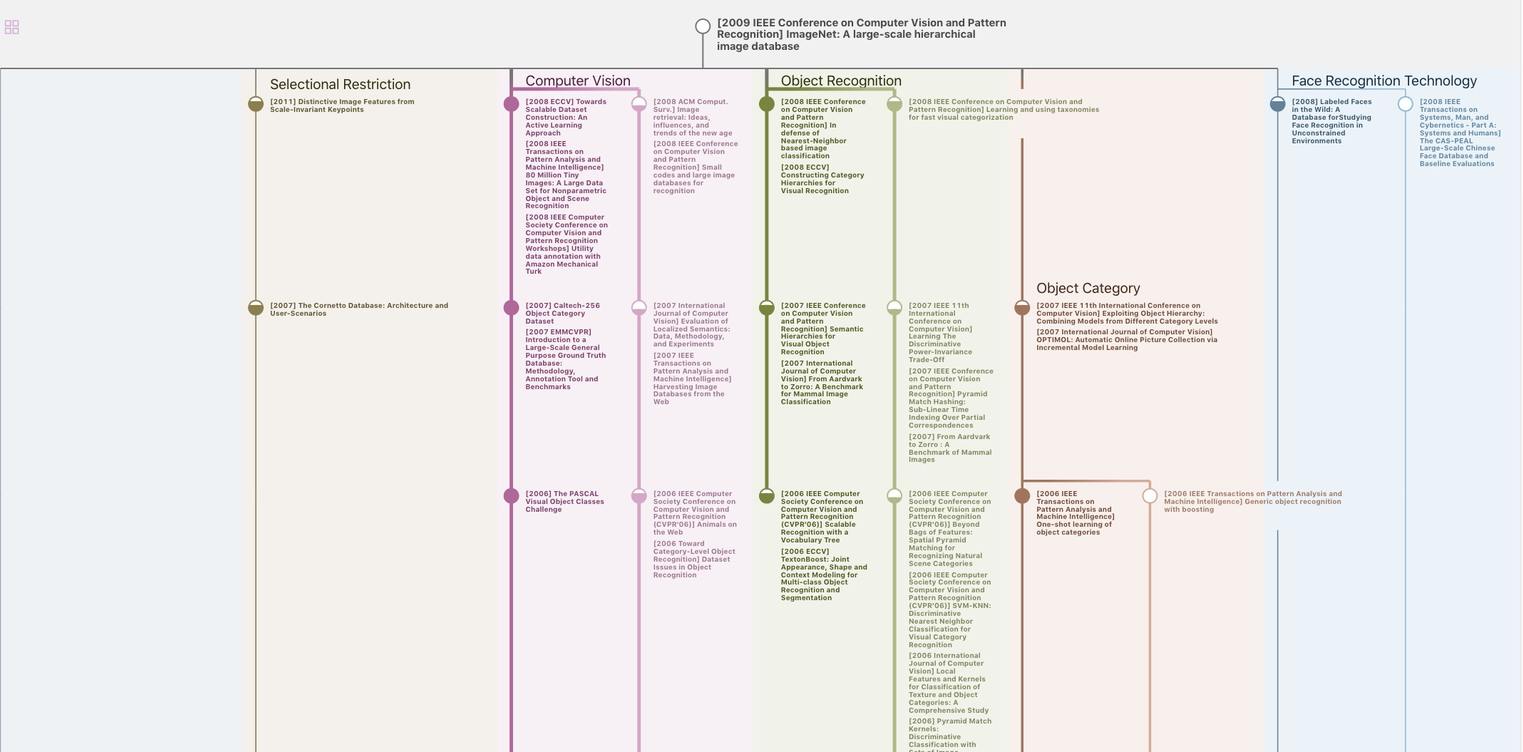
Generate MRT to find the research sequence of this paper
Chat Paper
Summary is being generated by the instructions you defined