Predictors of Pathological Complete Response Following Neoadjuvant Chemoradiotherapy for Rectal Cancer.
Journal of Cancer Research and Therapeutics(2022)
关键词
Neoadjuvant chemoradiotherapy,pathological complete response,rectal cancer,tumor regression
AI 理解论文
溯源树
样例
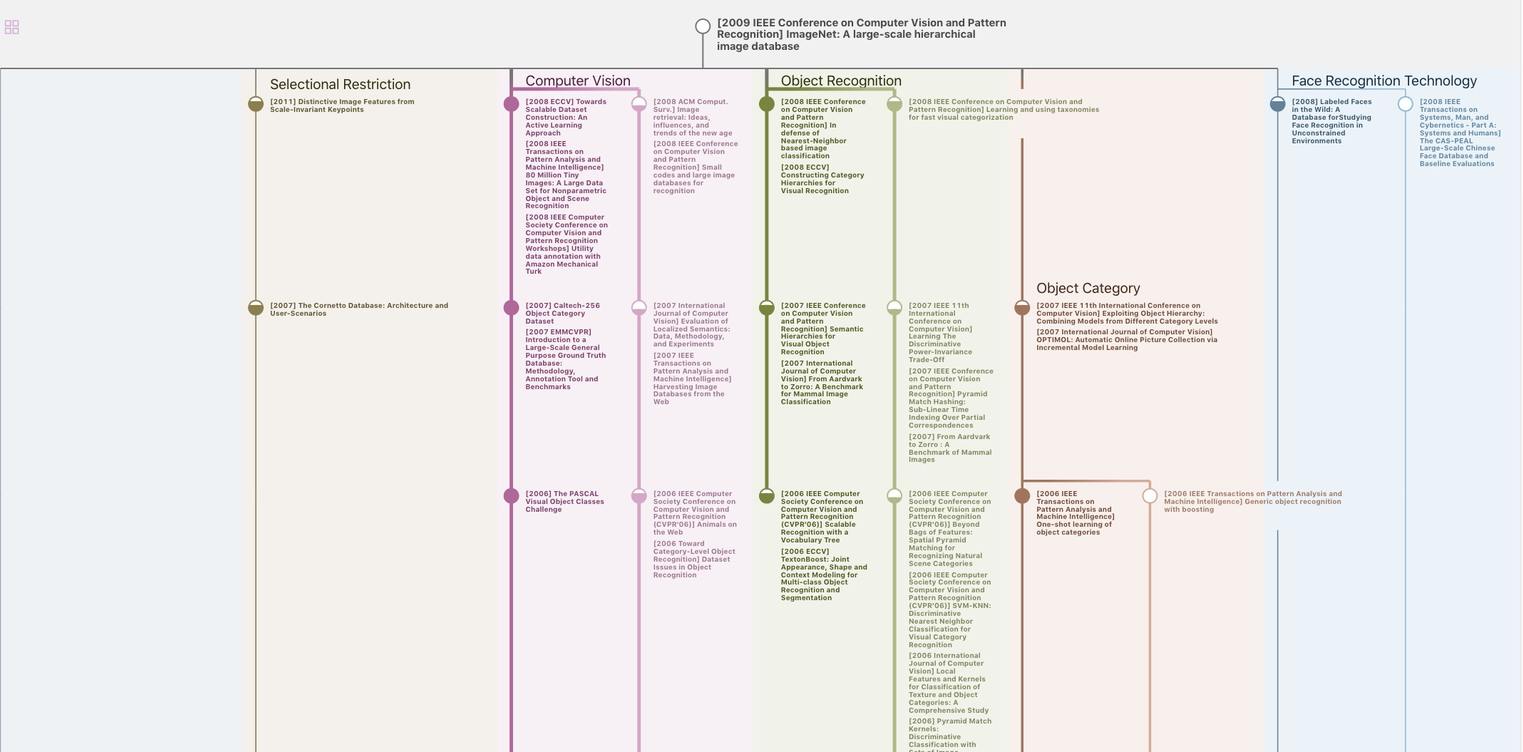
生成溯源树,研究论文发展脉络
Chat Paper
正在生成论文摘要