Radiomic-based Machine Learning Model for the Accurate Prediction of Prostate Cancer Risk Stratification
The British journal of radiology/British journal of radiology(2023)
摘要
Objectives: To precisely predict prostate cancer (PCa) risk stratification, we constructed a machine learning (ML) model based on magnetic resonance imaging (MRI) radiomic features. Methods: Between August 2016 and May 2021, patients with histologically proven PCa who underwent pre-operative MRI and prostate-specific antigen screening were included. The patients were grouped into different risk categories as defined by the European Association of Urology-European Association of Nuclear Medicine-European Society for Radiotherapy and Oncology-European Society of Urogenital Radiology-International Society of Geriatric Oncology guidelines. Using Artificial Intelligence Kit software, PCa regions of interest were delineated and radiomic features were extracted. Subsequently, predictable models were built by utilising five traditional ML approaches: support vector machine, logistic regression, gradient boosting decision tree, k-nearest neighbour and random forest (RF) classifiers. The classification capacity of the developed models was assessed by area under the receiver operating characteristic curve (AUC) analysis. Results: A total of 213 patients were enrolled, including 16 low-risk, 65 intermediate-risk, and 132 high-risk PCa patients. The risk stratification of PCa could be revealed by MRI radiomic features, and second-order features accounted for most of the selected features. Among the five established ML models, the RF model showed the best overall predictive performance (AUC = 0.87). After further analysis of the subgroups based on the RF model, the prediction of the high-risk group was the best (AUC = 0.89). Conclusion: This study demonstrated that the MR radiomics-based ML method could be a promising tool for predicting PCa risk stratification precisely. Advances in knowledge: The ML models have valuable prospect for accurate PCa risk assessment, which might contribute to customize treatment and surveillance strategies.
更多查看译文
AI 理解论文
溯源树
样例
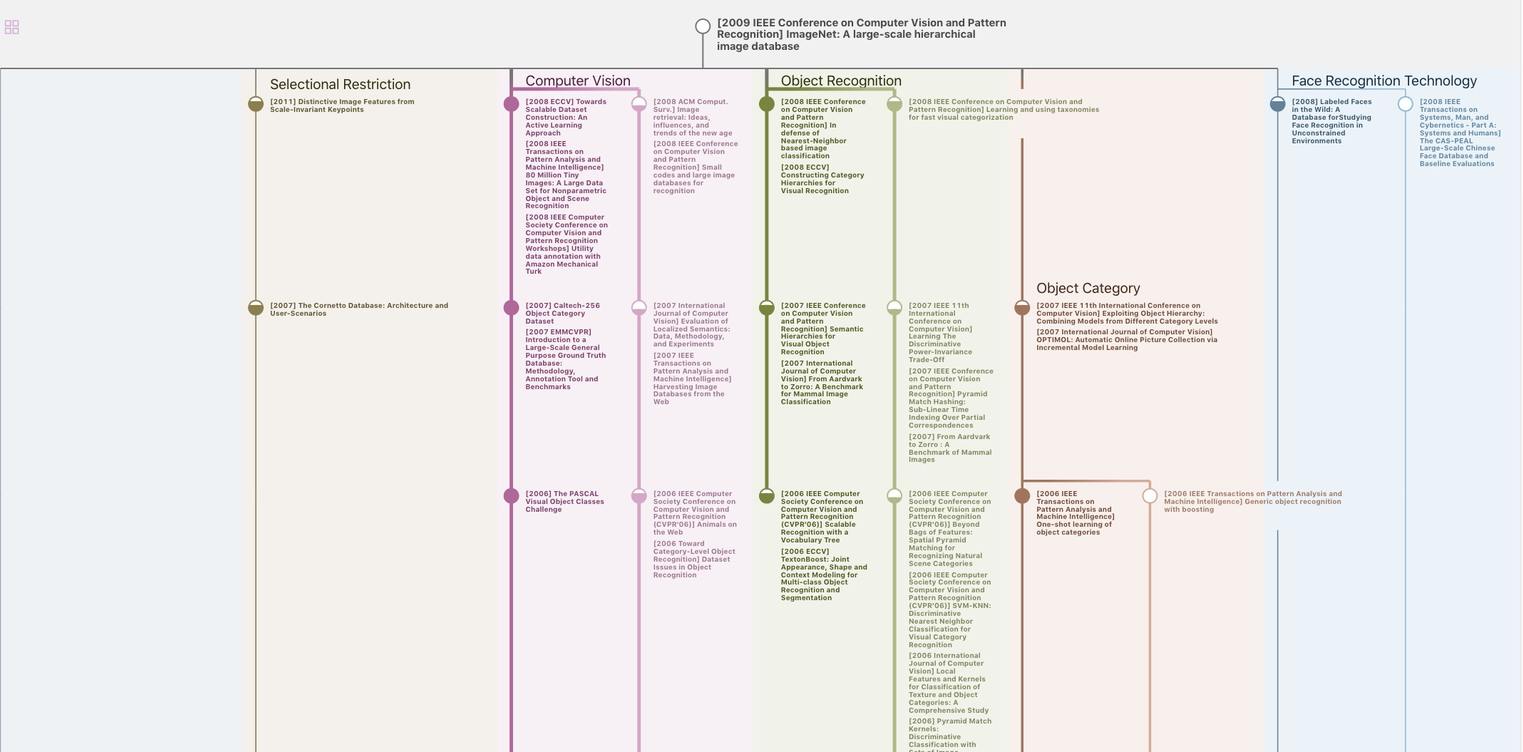
生成溯源树,研究论文发展脉络
Chat Paper
正在生成论文摘要