Distributed-Memory Randomized Algorithms for Sparse Tensor CP Decomposition.
PROCEEDINGS OF THE 36TH ACM SYMPOSIUM ON PARALLELISM IN ALGORITHMS AND ARCHITECTURES, SPAA 2024(2024)
摘要
Candecomp / PARAFAC (CP) decomposition, a generalization of the matrix singular value decomposition to higher-dimensional tensors, is a popular tool for analyzing multidimensional sparse data. On tensors with billions of nonzero entries, computing a CP decomposition is a computationally intensive task. We propose the first distributed-memory implementations of two randomized CP decomposition algorithms, CP-ARLS-LEV and STS-CP, that offer nearly an order-of-magnitude speedup at high decomposition ranks over well-tuned non-randomized decomposition packages. Both algorithms rely on leverage score sampling and enjoy strong theoretical guarantees, each with varying time and accuracy tradeoffs. We tailor the communication schedule for our random sampling algorithms, eliminating expensive reduction collectives and forcing communication costs to scale with the random sample count. Finally, we optimize the local storage format for our methods, switching between analogues of compressed sparse column and compressed sparse row formats. Experiments show that our methods are fast and scalable, producing 11x speedup over SPLATT by decomposing the billion-scale Reddit tensor on 512 CPU cores in under two minutes.
更多查看译文
关键词
Sparse Tensors,CP Decomposition,Randomized Linear Algebra,Leverage Score Sampling
AI 理解论文
溯源树
样例
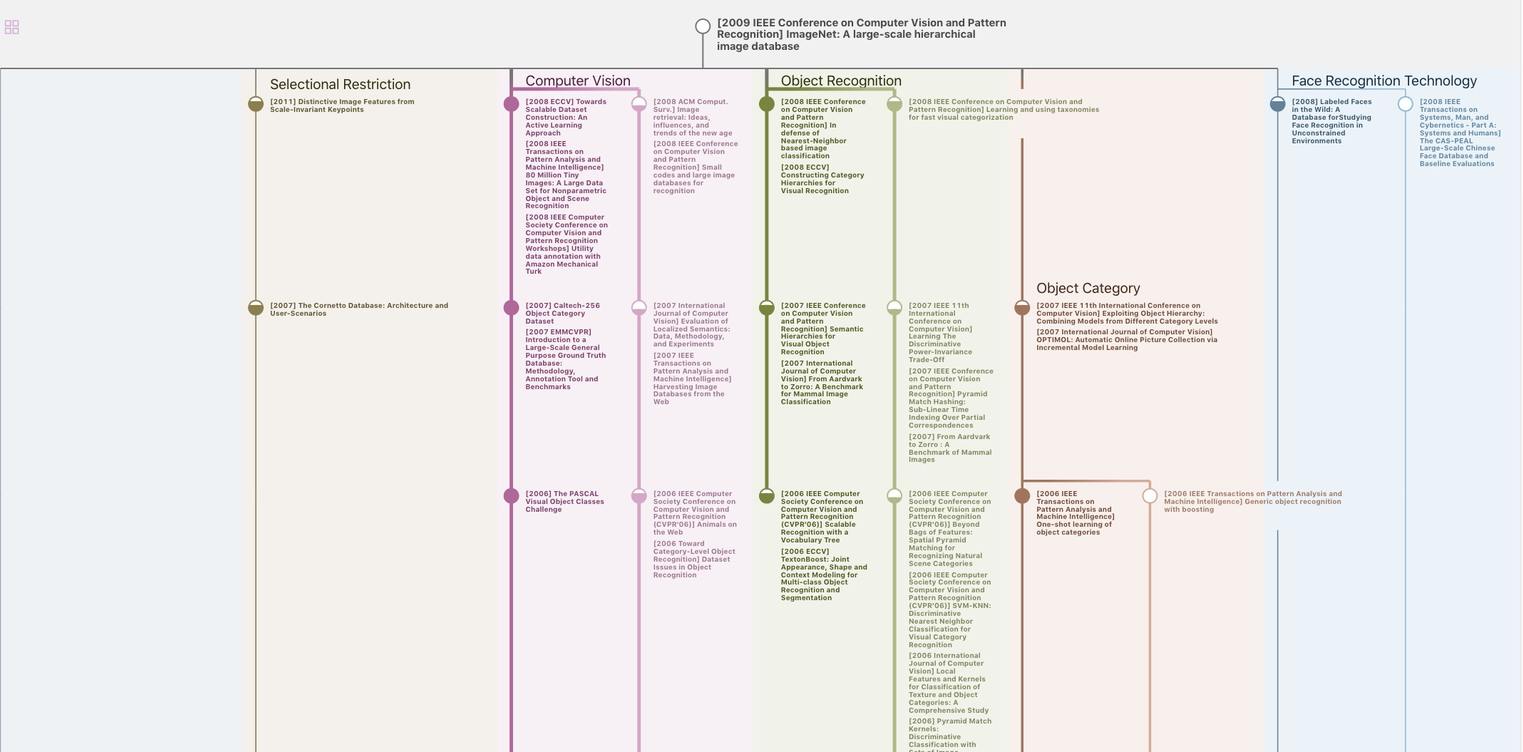
生成溯源树,研究论文发展脉络
Chat Paper
正在生成论文摘要