Decoding Individual Differences in Expressing and Suppressing Anger from Structural Brain Networks: A Supervised Machine Learning Approach.
Social Science Research Network(2022)
摘要
Anger can be broken down into different elements: a transitory state (state anger), a stable personality feature (trait anger), a tendency to express it (anger-out), or to suppress it (anger-in), and the ability to regulate it (anger control). These elements are characterized by individual differences that vary across a continuum. Among them, the abilities to express and suppress anger are of particular relevance as they determine outcomes and enable successful anger management in daily situations. The aim of this study was to demonstrate that anger suppression and expression can be decoded by patterns of grey matter of specific well-known brain networks. To this aim, a supervised machine learning technique, known as Kernel Ridge Regression, was used to predict anger expression and suppression scores of 212 healthy subjects from the grey matter concentration. Results show that individual differences in anger suppression were predicted by two grey matter patterns associated with the Default-Mode Network and the Salience Network. Additionally, individual differences in anger expression were predicted by a circuit mainly involving subcortical and fronto-temporal regions when considering whole brain grey matter features. These results expand previous findings regarding the neural bases of anger by showing that individual differences in specific anger-related components can be predicted by the grey matter features of specific networks.
更多查看译文
关键词
Anger,Anger suppression,Anger expression,Brain networks,Kernel Ridge Regression,Machine Learning
AI 理解论文
溯源树
样例
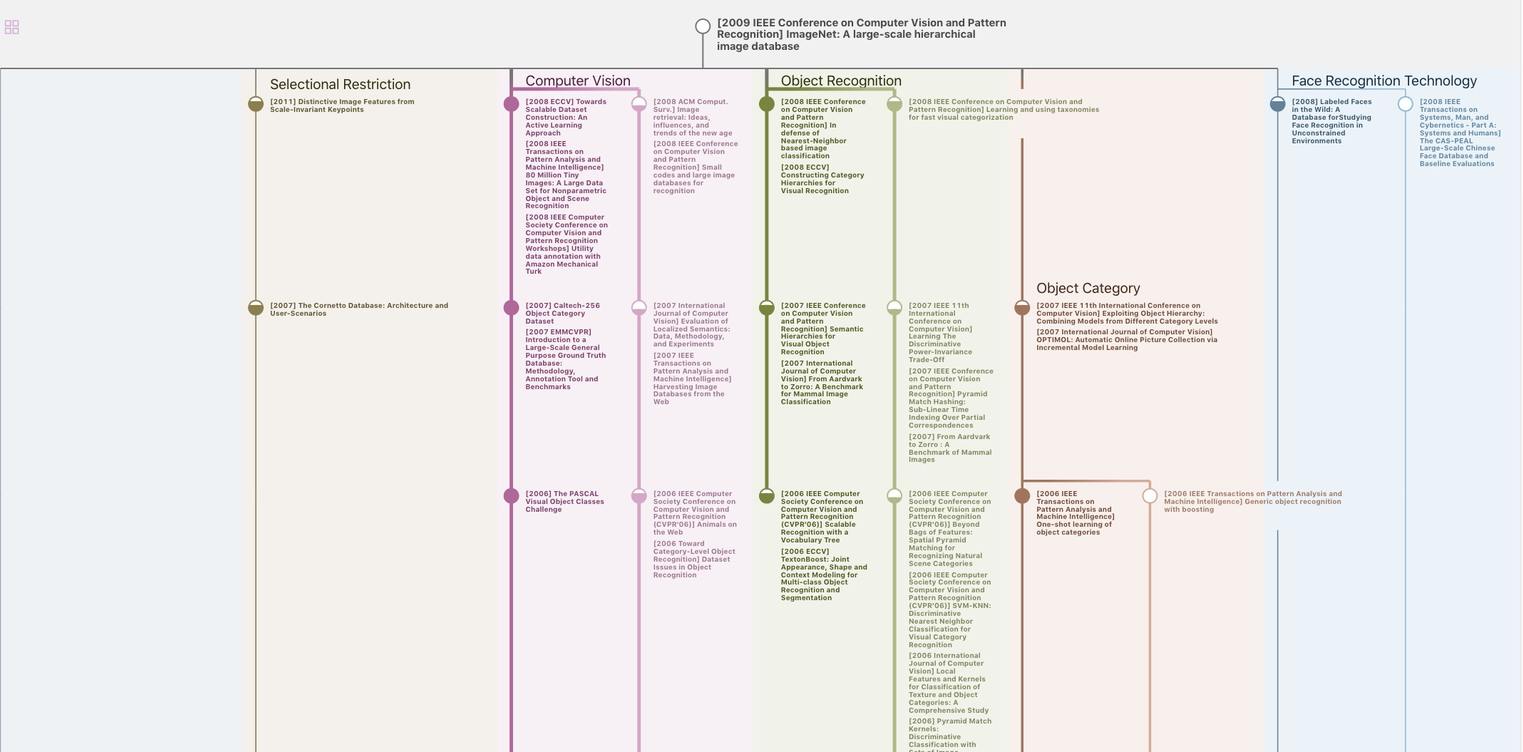
生成溯源树,研究论文发展脉络
Chat Paper
正在生成论文摘要