RHO ($\rho$): Reducing Hallucination in Open-domain Dialogues with Knowledge Grounding
conf_acl(2022)
摘要
Dialogue systems can leverage large pre-trained language models and knowledge to generate fluent and informative responses. However, these models are still prone to produce hallucinated responses not supported by the input source, which greatly hinders their application. The heterogeneity between external knowledge and dialogue context challenges representation learning and source integration, and further contributes to unfaithfulness. To handle this challenge and generate more faithful responses, this paper presents RHO ($\rho$) utilizing the representations of linked entities and relation predicates from a knowledge graph (KG). We propose (1) local knowledge grounding to combine textual embeddings with the corresponding KG embeddings; and (2) global knowledge grounding to equip RHO with multi-hop reasoning abilities via the attention mechanism. In addition, we devise a response re-ranking technique based on walks over KG sub-graphs for better conversational reasoning. Experimental results on OpenDialKG show that our approach significantly outperforms state-of-the-art methods on both automatic and human evaluation by a large margin, especially in hallucination reduction (17.54% in FeQA).
更多查看译文
关键词
knowledge grounding,rho,hallucination,open-domain
AI 理解论文
溯源树
样例
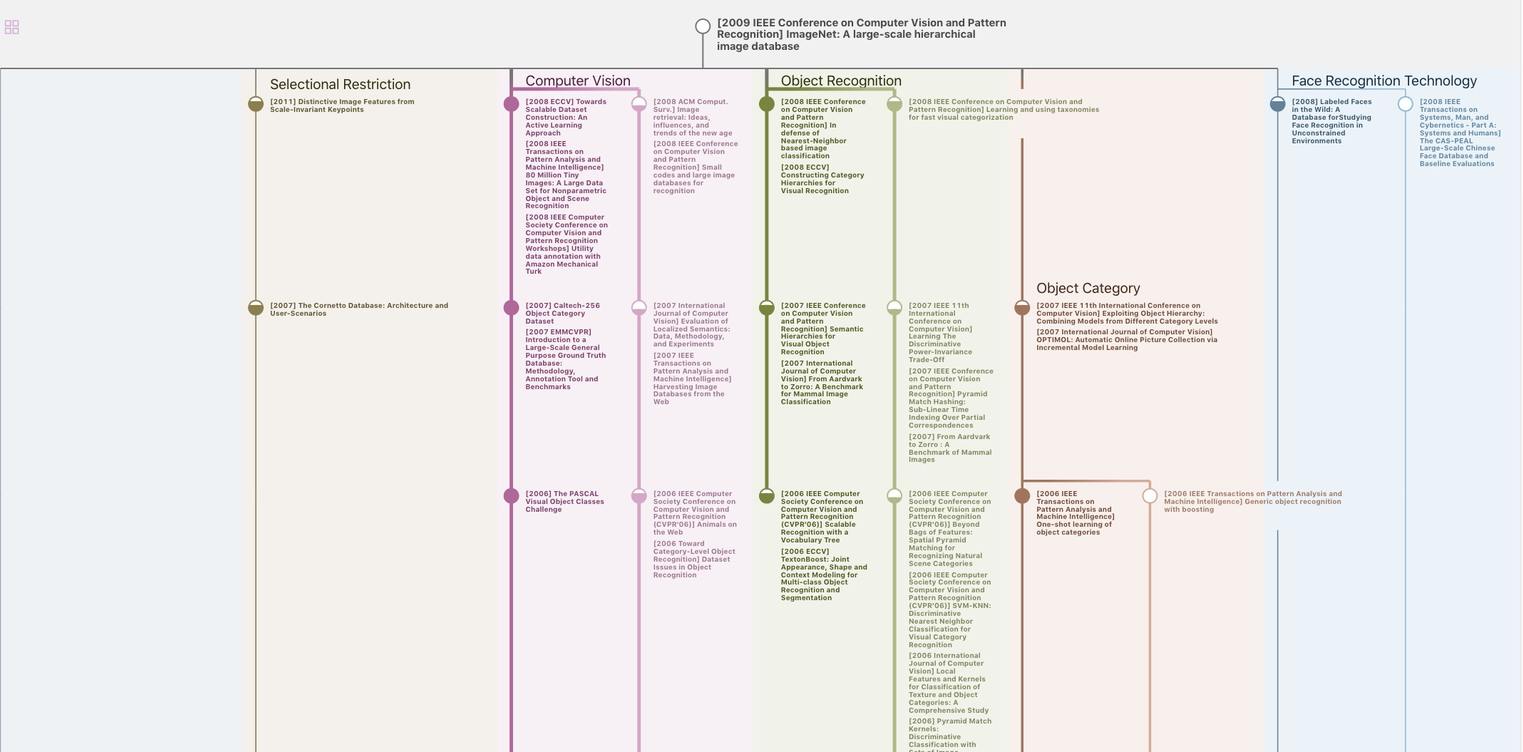
生成溯源树,研究论文发展脉络
Chat Paper
正在生成论文摘要