Towards a Human-like Chatbot using Deep Adversarial Learning
2022 14th International Conference on Knowledge and Systems Engineering (KSE)(2022)
摘要
Conversational agents are getting more popular and applied in a wide range of practical application areas. The main task of these agents is not only to generate context-appropriate responses to a given query but also to make the conversation human-like. Thanks to the ability of deep learning based models in natural language modeling, recent studies have made progress in designing conversational agents that can provide more semantically accurate responses. However, the naturalness in such conversation setting has not been given adequate attention in these studies. This paper aims to incorporate both important criteria of accuracy and naturalness of conversation in developing a new model for conversational agents. To this end, inspired by the idea of Turing test and the idea of adversarial learning strategy, we propose to design a model based on generative deep neural networks that interestingly allow to generate accurate responses optimized by the mechanics of imitating human-generated conversations. Experimental results demonstrate that the proposed models produce more natural and accurate responses, yielding significant gains in BLEU scores.
更多查看译文
关键词
BERT,Chatbot,Conversational agent,Sequence to sequence,Generative Adversarial Nets
AI 理解论文
溯源树
样例
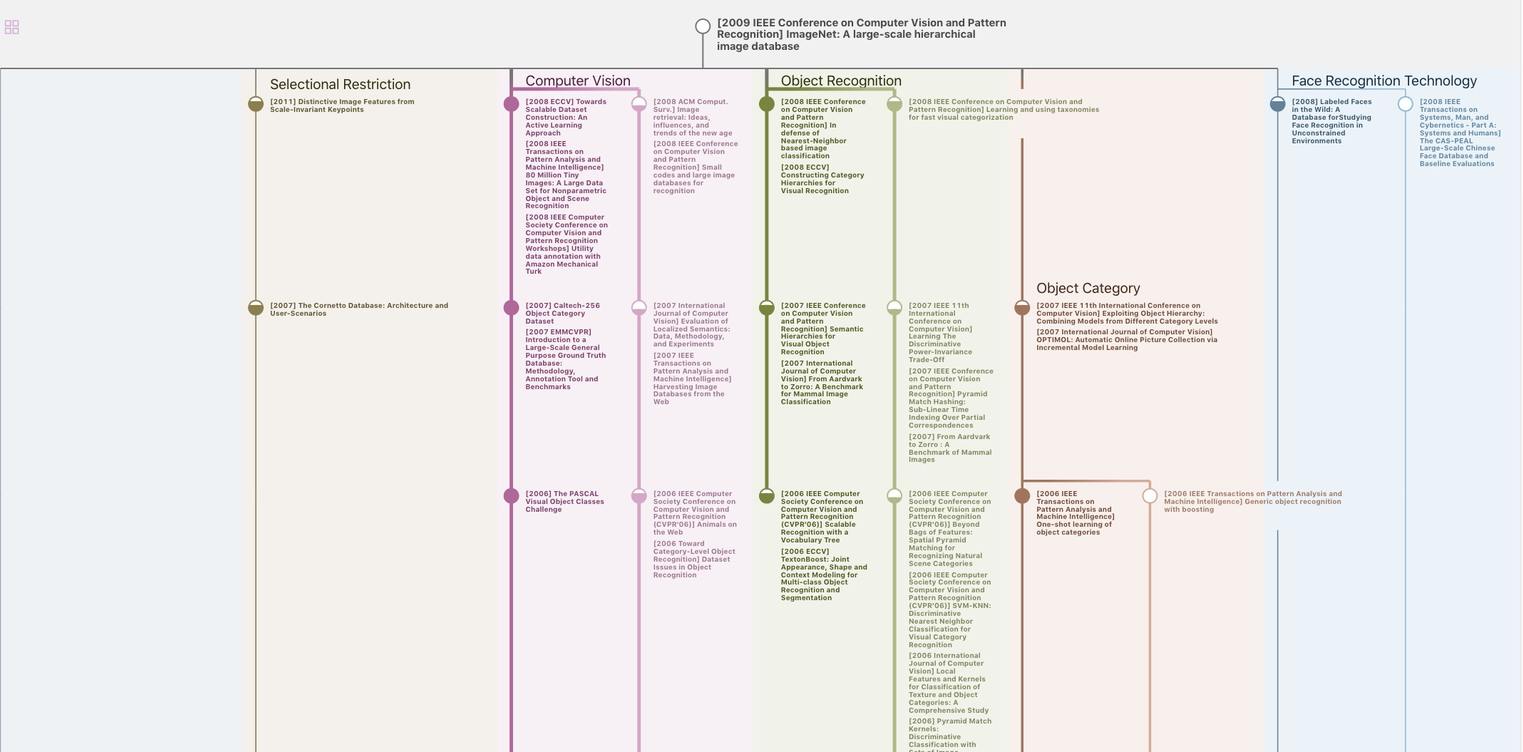
生成溯源树,研究论文发展脉络
Chat Paper
正在生成论文摘要