PAINT: Path Aware Iterative Network Tomography for Link Metric Inference
2022 IEEE 30th International Conference on Network Protocols (ICNP)(2022)
摘要
Understanding link-level performance is key to assuring the quality of cloud-based and OTT services, optimal path selection, robust network operations and beyond. However, direct measurement of each link not only incurs high overhead at the Internet-scale but also is infeasible due to lack of access to network measurement information beyond AS boundaries and functional limitations at relay nodes. Although network tomography is well suited, existing approaches are insufficient due to their unrealistic assumptions with respect to stability, controllability, and visibility. Motivated by this, we propose PAINT, an online iterative algorithm that estimates and refines link-level performance metrics based on path-level measurement. In PAINT, the link metrics are iteratively estimated by minimizing their least square error (LSE) and calibrated based on the comparison of weight between the estimated shortest paths (SPs) and best-known paths from end-to-end path measurements. The key insight is that when there is inconsistency between these paths, then weights of links on the estimated SP are likely mis-estimated, triggering a further round of estimation to refine the estimated link metrics. Evaluation of PAINT, focusing on link delay estimation, using four different real network topologies and two real-world measurement datasets (including one we collected) shows that relative to existing approaches, it yields up to 3x gain in absolute link delay estimation accuracy and improves decisions dependent on link delay estimation by up to 5x in relative error.
更多查看译文
AI 理解论文
溯源树
样例
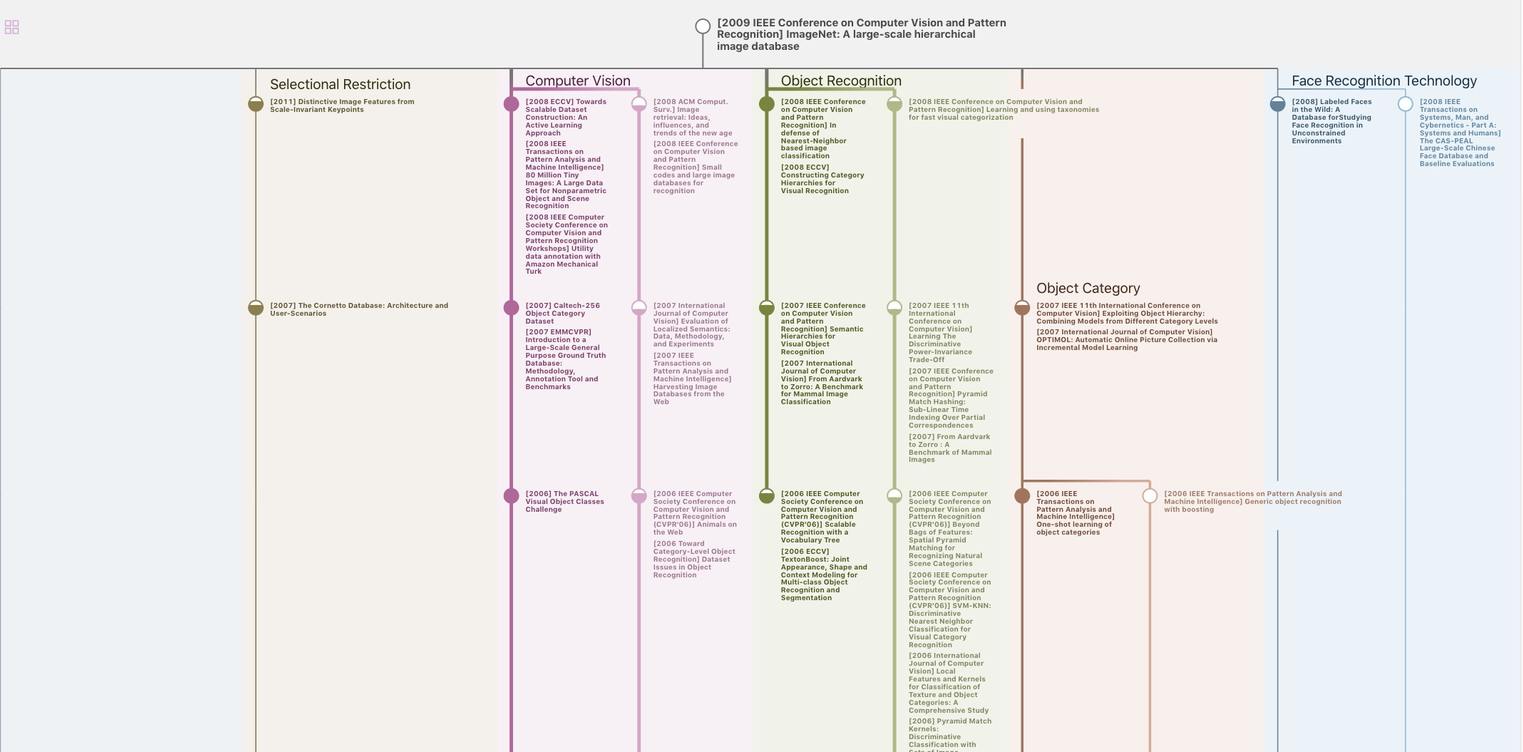
生成溯源树,研究论文发展脉络
Chat Paper
正在生成论文摘要