UEIN: A User Evolving Interests Network for Click-Through Rate Prediction
2021 IEEE 15th International Conference on Big Data Science and Engineering (BigDataSE)(2021)
摘要
The Click-Through Rate (CTR) prediction via modeling the change of user interest is of great significance task to industrial applications. Most of the existing methods based on collaborative filtering (CF) and recurrent neural network (RNN) that focus on capturing user's long-term and short-term interests separately, or combine these interests in a tough way. However, the effect of long- and short-term interests accounts different ratios to the next-item decision of user, rather than equally. To address this issue, we propose UEIN, a novel User Evolving Interest Network for CTR prediction task, that can capture the change of user preference via adaptively fusion the user's long-term and short-term interests over time. To explicitly learn the user preference on item category, we add the item category information into user behavior sequence, by clustering the items into different categories. Then we divide user historical behaviors into two sequence parts, long-term and short-term behaviors sequence. Based on this, we leverage the attention network to learn the user's current interest from the short-term behaviors sequence, while a dense network is adopted to extract the user's general interest from the long-term sequence. After that, an adaptive fusion mechanism is proposed to combine the current and general interest adaptively, and the prediction result is generated based on the user's combined interest. Extensive experimental results on industrial datasets demonstrated that the superiority of our proposed model, compared to the state-of-the-art models.
更多查看译文
关键词
recommendation system,neural networks,deep learning,sequence recommendation
AI 理解论文
溯源树
样例
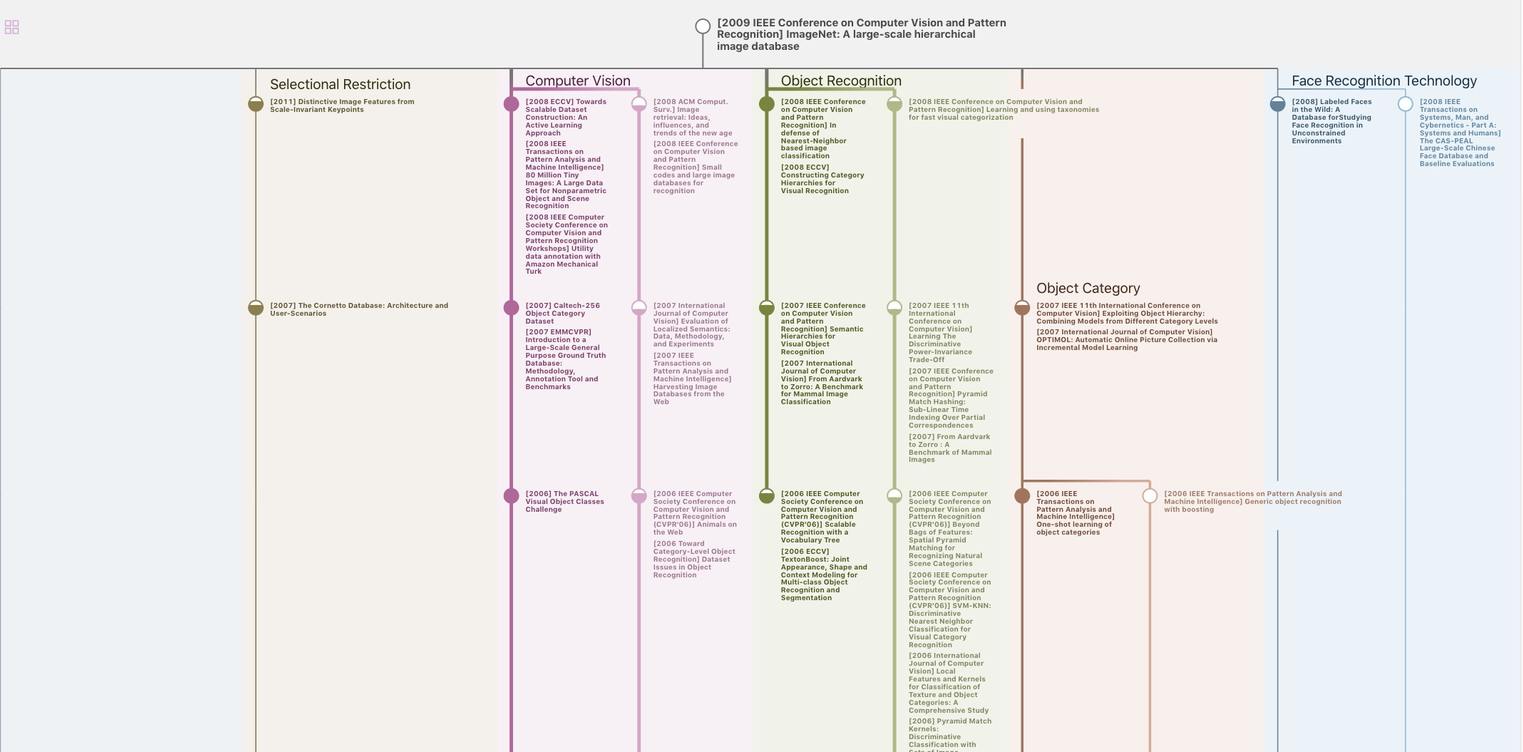
生成溯源树,研究论文发展脉络
Chat Paper
正在生成论文摘要