Using Machine Learning to Predict Multiphase Flow Through Complex Fractures
Energies(2022)
摘要
Multiphase flow properties of fractures are important in engineering applications such as hydraulic fracturing, evaluating the sealing capacity of caprocks, and the productivity of hydrocarbon-bearing tight rocks. Due to the computational requirements of high fidelity simulations, investigations of flow and transport through fractures typically rely on simplified assumptions applied to large fracture networks. These simplifications ignore the effect of pore-scale capillary phenomena and 3D realistic fracture morphology (for instance, tortuosity, contact points, and crevasses) that lead to macro-scale effective transport properties. The effect of these properties can be studied through lattice Boltzmann simulations, but they require high performance computing clusters and are generally limited in their domain size. In this work, we develop a technique to represent 3D fracture geometries and fluid distributions in 2D without losing any information. Using this innovative approach, we present a specialized machine learning model which only requires a few simulations for training but still accurately predicts fluid flow through 3D fractures. We demonstrate our technique using simulations of a water filled fracture being displaced by supercritical CO2. By generating highly efficient simulations of micro-scale multiphase flow in fractures, we hope to investigate a wide range of fracture types and generalize our method to be incorporated into larger discrete fracture network simulations.
更多查看译文
关键词
machine learning,multiphase flow,unsteady-state,time-dependency,hydraulic fractures,lattice-Boltzmann,CO2
AI 理解论文
溯源树
样例
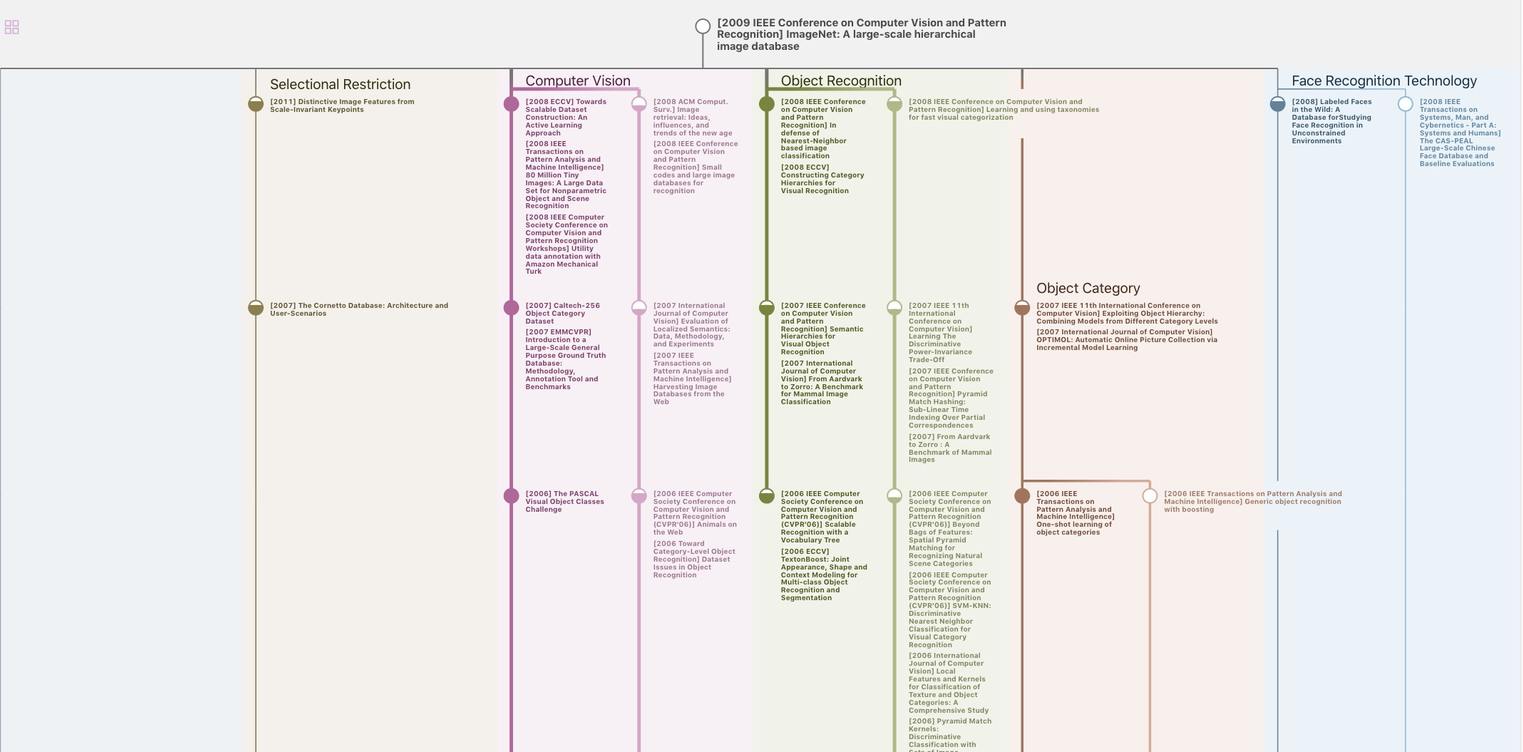
生成溯源树,研究论文发展脉络
Chat Paper
正在生成论文摘要