Parametric Approximation to Optimal Averaging in Superimposed Training Schemes under Realistic Time-Variant Channels
2022 13th International Symposium on Communication Systems, Networks and Digital Signal Processing (CSNDSP)(2022)
Abstract
Superimposed Training (ST) with orthogonal frequency division multiplexing (OFDM) scheme has become an attractive solution to meet the goals of the fifth generation (5G) of mobile communications, by improving the channel estimation performance, which is one of the main challenge in multiple input multiple output (MIMO) systems. This technique does not hinder the throughput, however, it introduces an intrinsic interference since the data and the reference symbols are sent together. In order to mitigate it, several studies propose a time averaging over several OFDM received symbols, where the optimal length of this averaging can be analytically computed by solving a transcendental equation. In this paper, this optimal averaging is approximated by a low complexity parametric approach based on a multiple linear regression model that inputs two parameters, the signal-to-noise ratio (SNR) and the relative speed between the transmitter and receiver, which effectively represents the variability of the channel in time. Results show that the approximated solutions give an error of 0.05% on average and 7% at most in terms of the provided mean square error (MSE) of the channel estimation.
MoreTranslated text
Key words
OFDM,Superimposed Training,Time-Variant Channel,Channel Estimation,Least Squares,Averaging,Multiple Linear Regression
AI Read Science
Must-Reading Tree
Example
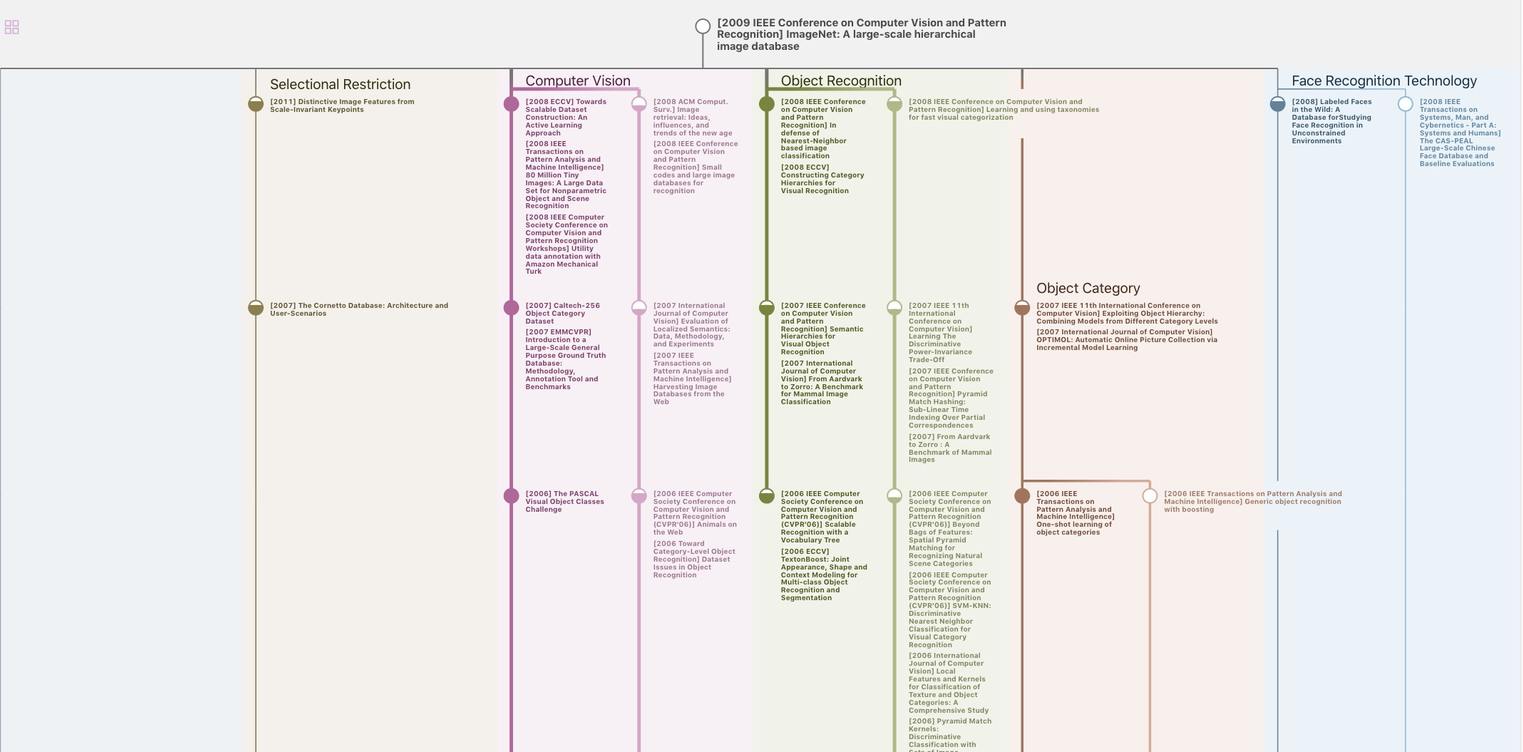
Generate MRT to find the research sequence of this paper
Chat Paper
Summary is being generated by the instructions you defined