CDDSA: Contrastive domain disentanglement and style augmentation for generalizable medical image segmentation.
Medical image analysis(2023)
摘要
Generalization to previously unseen images with potential domain shifts is essential for clinically applicable medical image segmentation. Disentangling domain-specific and domain-invariant features is key for Domain Generalization (DG). However, existing DG methods struggle to achieve effective disentanglement. To address this problem, we propose an efficient framework called Contrastive Domain Disentanglement and Style Augmentation (CDDSA) for generalizable medical image segmentation. First, a disentangle network decomposes the image into domain-invariant anatomical representation and domain-specific style code, where the former is sent for further segmentation that is not affected by domain shift, and the disentanglement is regularized by a decoder that combines the anatomical representation and style code to reconstruct the original image. Second, to achieve better disentanglement, a contrastive loss is proposed to encourage the style codes from the same domain and different domains to be compact and divergent, respectively. Finally, to further improve generalizability, we propose a style augmentation strategy to synthesize images with various unseen styles in real time while maintaining anatomical information. Comprehensive experiments on a public multi-site fundus image dataset and an in-house multi-site Nasopharyngeal Carcinoma Magnetic Resonance Image (NPC-MRI) dataset show that the proposed CDDSA achieved remarkable generalizability across different domains, and it outperformed several state-of-the-art methods in generalizable segmentation. Code is available at https://github.com/HiLab-git/DAG4MIA.
更多查看译文
关键词
Contrastive learning,Disentanglement,Domain generalization,Medical image segmentation
AI 理解论文
溯源树
样例
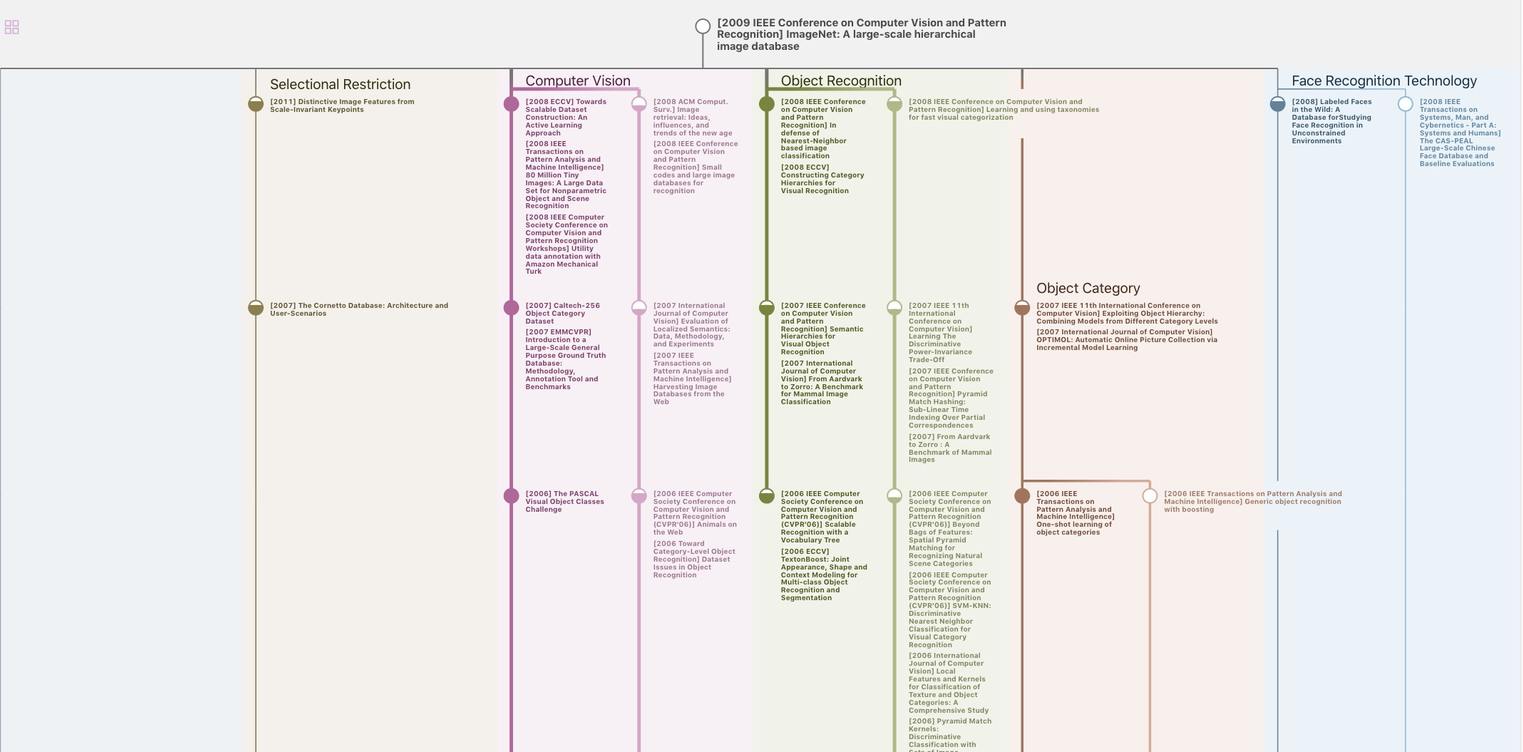
生成溯源树,研究论文发展脉络
Chat Paper
正在生成论文摘要