Variational Hyperparameter Inference for Few-Shot Learning Across Domains
IEEE Transactions on Circuits and Systems for Video Technology(2022)
摘要
The focus of few shot learning research has been on the development of meta-learning recently, where a meta-learner is trained on a variety of tasks in hopes of being generalizable to new tasks. Tasks in meta training and meta test are usually assumed to be from the same domain, which would not necessarily hold in real world scenarios. In this paper, we propose variational hyperparameter inference for few-shot learning across domains. Based on an especially successful algorithm named model agnostic meta learning, the proposed variational hyperparameter inference integrates meta learning and variational inference into the optimization of hyperparameters, which enables the meta-learner with adaptivity for generalization across domains. In particular, we choose to learn adaptive hyperparameters including the learning rate and weight decay to avoid the failure in the face of few labeled examples across domain. Moreover, we model hyperparameters as distributions instead of fixed values, which will further enhance the generalization ability by capturing the uncertainty. Extensive experiments are conducted on two benchmark datasets including few shot learning dataset within-domain and across-domain. The results demonstrate that our methods outperforms previous approaches consistently, and comprehensive ablation studies further validate its effectiveness on few shot learning both within domains and across domains.
更多查看译文
关键词
Meta learning,few-shot learning,domain adaptation,latent variable model,variational inference
AI 理解论文
溯源树
样例
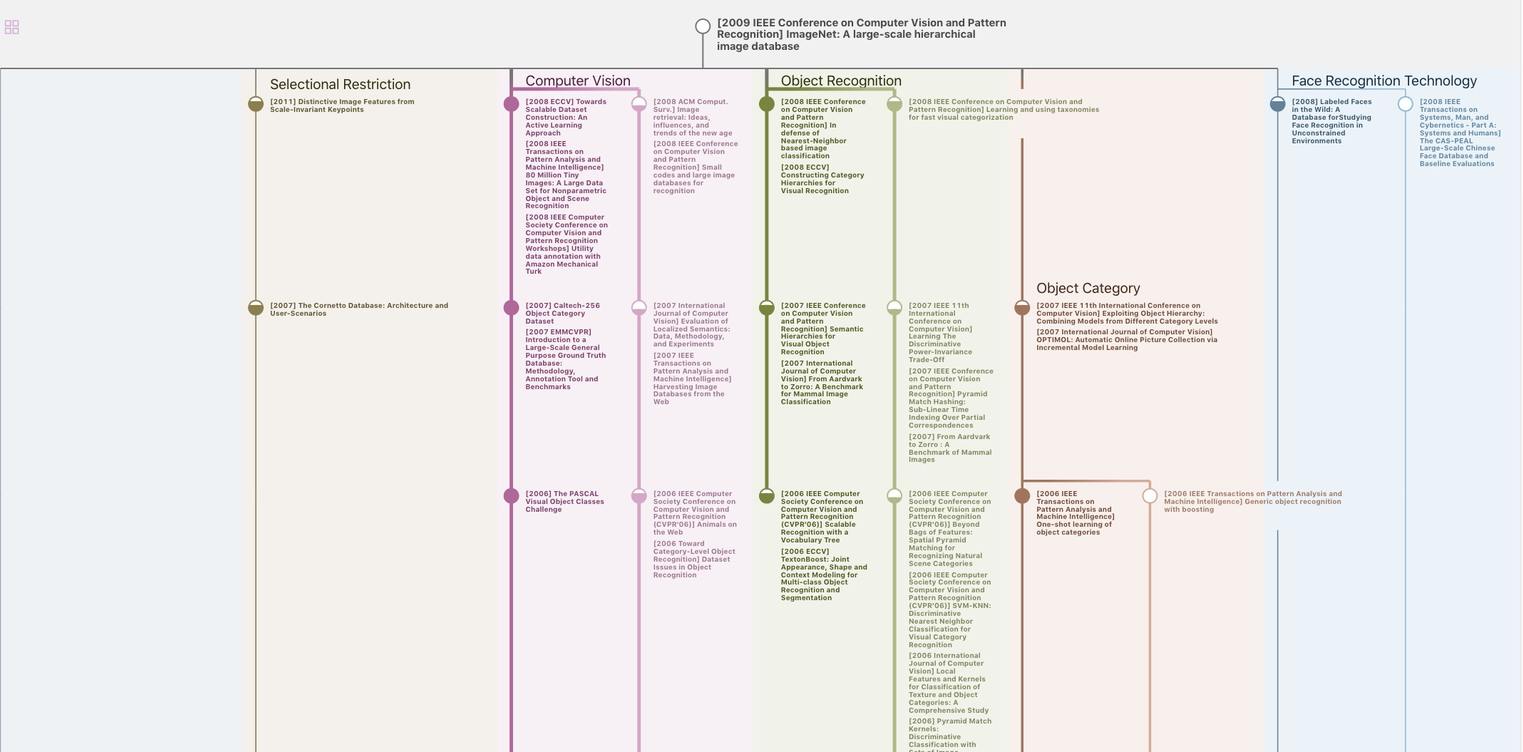
生成溯源树,研究论文发展脉络
Chat Paper
正在生成论文摘要