Fast Uncertainty Estimates in Deep Learning Interatomic Potentials
arxiv(2022)
摘要
Deep learning has emerged as a promising paradigm to give access to highly accurate predictions of molecular and materials properties. A common short-coming shared by current approaches, however, is that neural networks only give point estimates of their predictions and do not come with predictive uncertainties associated with these estimates. Existing uncertainty quantification efforts have primarily leveraged the standard deviation of predictions across an ensemble of independently trained neural networks. This incurs a large computational overhead in both training and prediction that often results in order-of-magnitude more expensive predictions. Here, we propose a method to estimate the predictive uncertainty based on a single neural network without the need for an ensemble. This allows us to obtain uncertainty estimates with virtually no additional computational overhead over standard training and inference. We demonstrate that the quality of the uncertainty estimates matches those obtained from deep ensembles. We further examine the uncertainty estimates of our methods and deep ensembles across the configuration space of our test system and compare the uncertainties to the potential energy surface. Finally, we study the efficacy of the method in an active learning setting and find the results to match an ensemble-based strategy at order-of-magnitude reduced computational cost.
更多查看译文
关键词
fast uncertainty estimates,interatomic potentials,deep learning
AI 理解论文
溯源树
样例
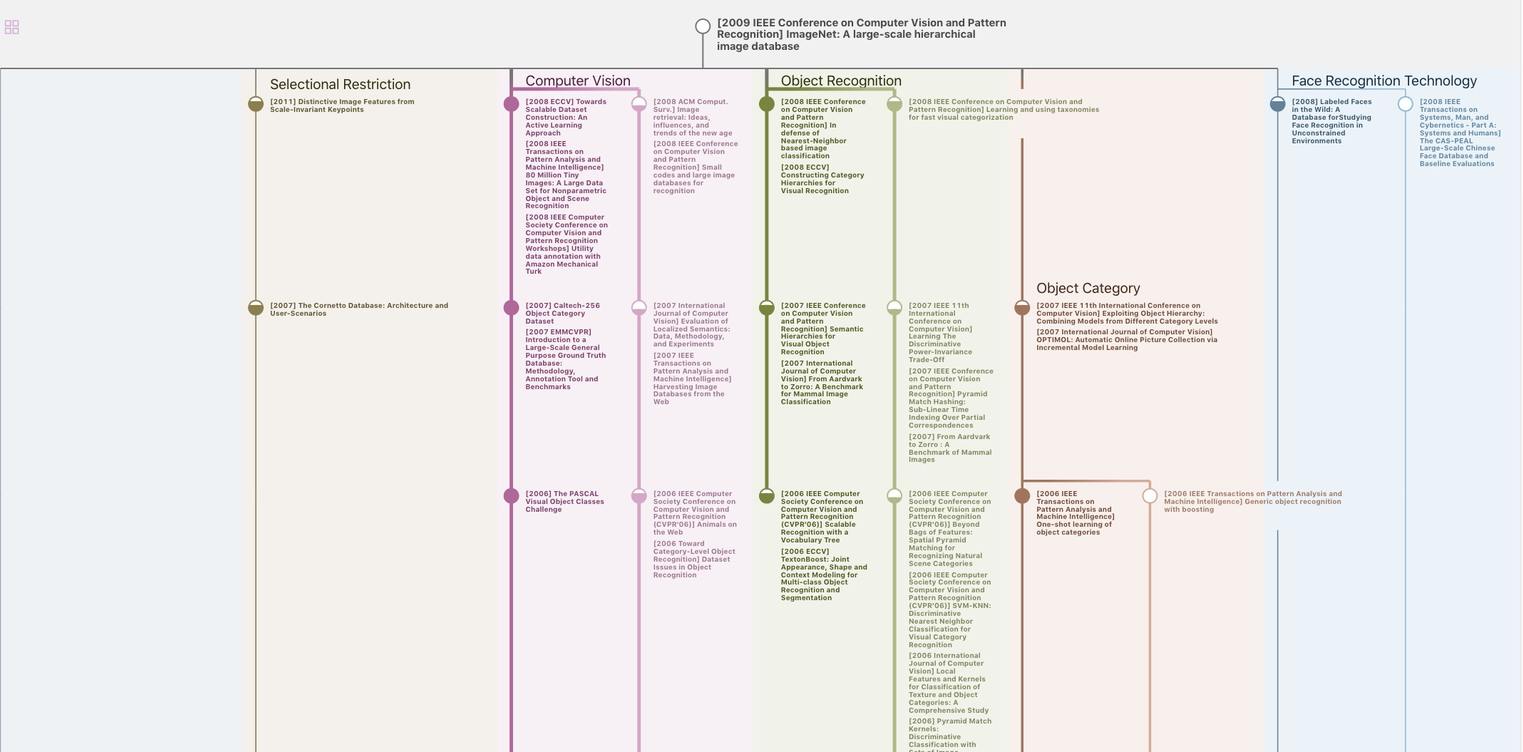
生成溯源树,研究论文发展脉络
Chat Paper
正在生成论文摘要