Dimensional Homogeneity Constrained Gene Expression Programming for Discovering Governing Equations
Journal of fluid mechanics(2024)
Abstract
Data-driven discovery of governing equations is of great significance for helping us understand intrinsic mechanisms and build physical models. Recently, numerous highly innovative algorithms have emerged, aimed at inversely discovering the underlying governing equations from data, such as sparse regression-based methods and symbolic regression-based methods. Along this direction, a novel dimensional homogeneity constrained gene expression programming (DHC-GEP) method is proposed in this work. The DHC-GEP simultaneously discovers the forms and coefficients of functions using basic mathematical operators and physical variables, without requiring preassumed candidate functions. The constraint of dimensional homogeneity is capable of filtering out the overfitting equations effectively. The key advantages of DHC-GEP compared with the original gene expression programming, including being more robust to hyperparameters, the noise level and the size of datasets, are demonstrated on two benchmark studies. Furthermore, DHC-GEP is employed to discover the unknown constitutive relations of two representative non-equilibrium flows. Galilean invariance and the second law of thermodynamics are imposed as constraints to enhance the reliability of the discovered constitutive relations. Comparisons, both quantitative and qualitative, indicate that the derived constitutive relations are more accurate than the conventional Burnett equations in a wide range of Knudsen numbers and Mach numbers, and are also applicable to the cases beyond the parameter space of the training data.
MoreTranslated text
Key words
machine learning
AI Read Science
Must-Reading Tree
Example
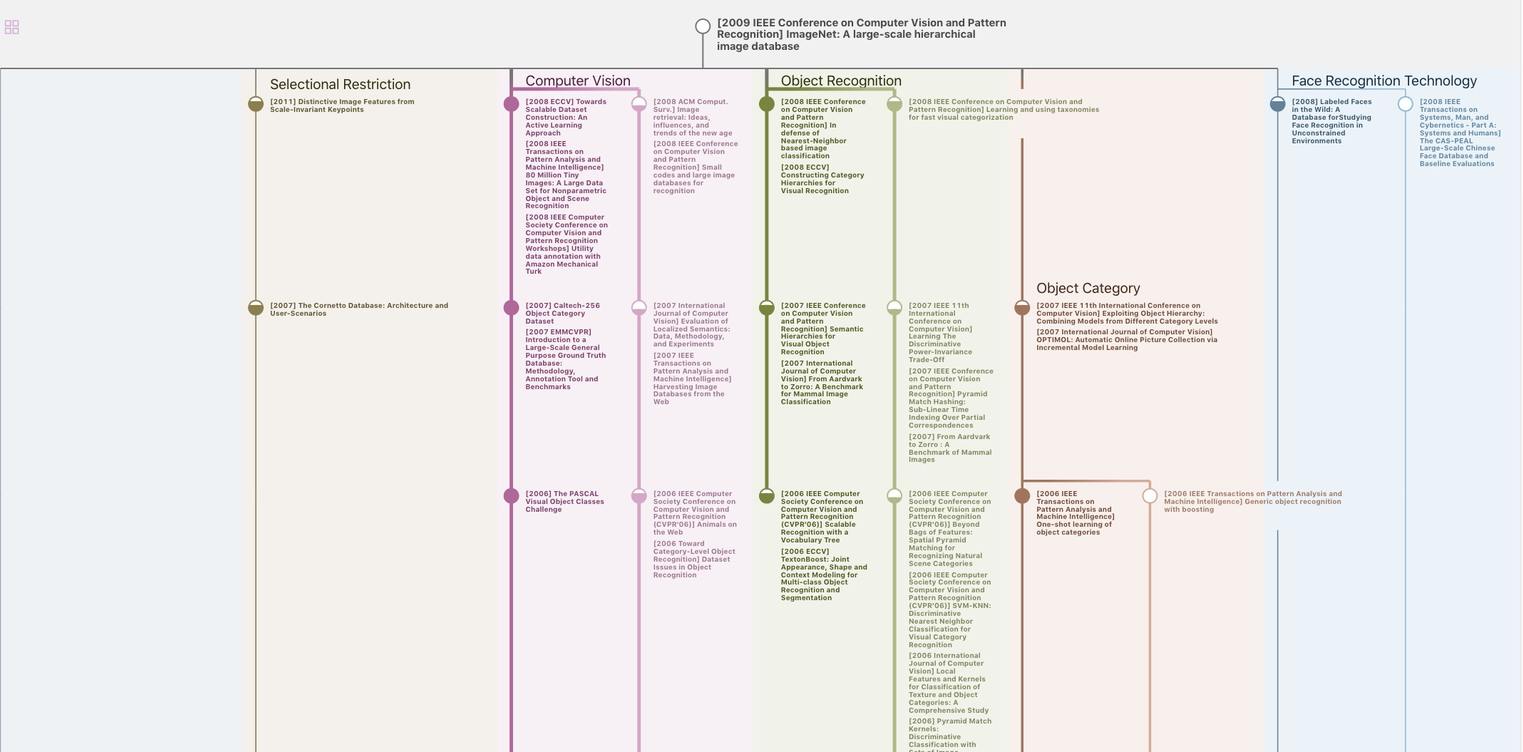
Generate MRT to find the research sequence of this paper
Chat Paper
Summary is being generated by the instructions you defined