European Collaborative and Interprofessional Capability Framework for Prevention and Management of Frailty—a Consensus Process Supported by the Joint Action for Frailty Prevention (ADVANTAGE) and the European Geriatric Medicine Society (Eugms)
WOS(2020)
Department of Internal Medicine | Health Service Executive of Ireland | Company of Psychosocial Research and Intervention | National Health Services Orkney | Regional Ministry of Health of Andalusia | Catholic University of Louvain | National Center of Public Health and Analyses | Croatian Institute of Public Health | Ministry of Health of the Republic of Cyprus | Finnish Institute for Health and Welfare | Ministry of Health and Social Solidarity | Medical University of Hannover | Ministry of Human Capacities | Italian National Health Institute | Lithuanian University of Health Sciences | San Vincent De Paule Long Term Care Facility | National Institute for Public Health and the Environment | Norwegian Institute of Public Health | National Institute of Geriatrics | Ministry of Health | Babeș-Bolyai University | National Institute of Public Health | National Health Service Lanarkshire | European Geriatric Medicine Society (EuGMS) | Hospital Universitario de Getafe
- Pretraining has recently greatly promoted the development of natural language processing (NLP)
- We show that M6 outperforms the baselines in multimodal downstream tasks, and the large M6 with 10 parameters can reach a better performance
- We propose a method called M6 that is able to process information of multiple modalities and perform both single-modal and cross-modal understanding and generation
- The model is scaled to large model with 10 billion parameters with sophisticated deployment, and the 10 -parameter M6-large is the largest pretrained model in Chinese
- Experimental results show that our proposed M6 outperforms the baseline in a number of downstream tasks concerning both single modality and multiple modalities We will continue the pretraining of extremely large models by increasing data to explore the limit of its performance
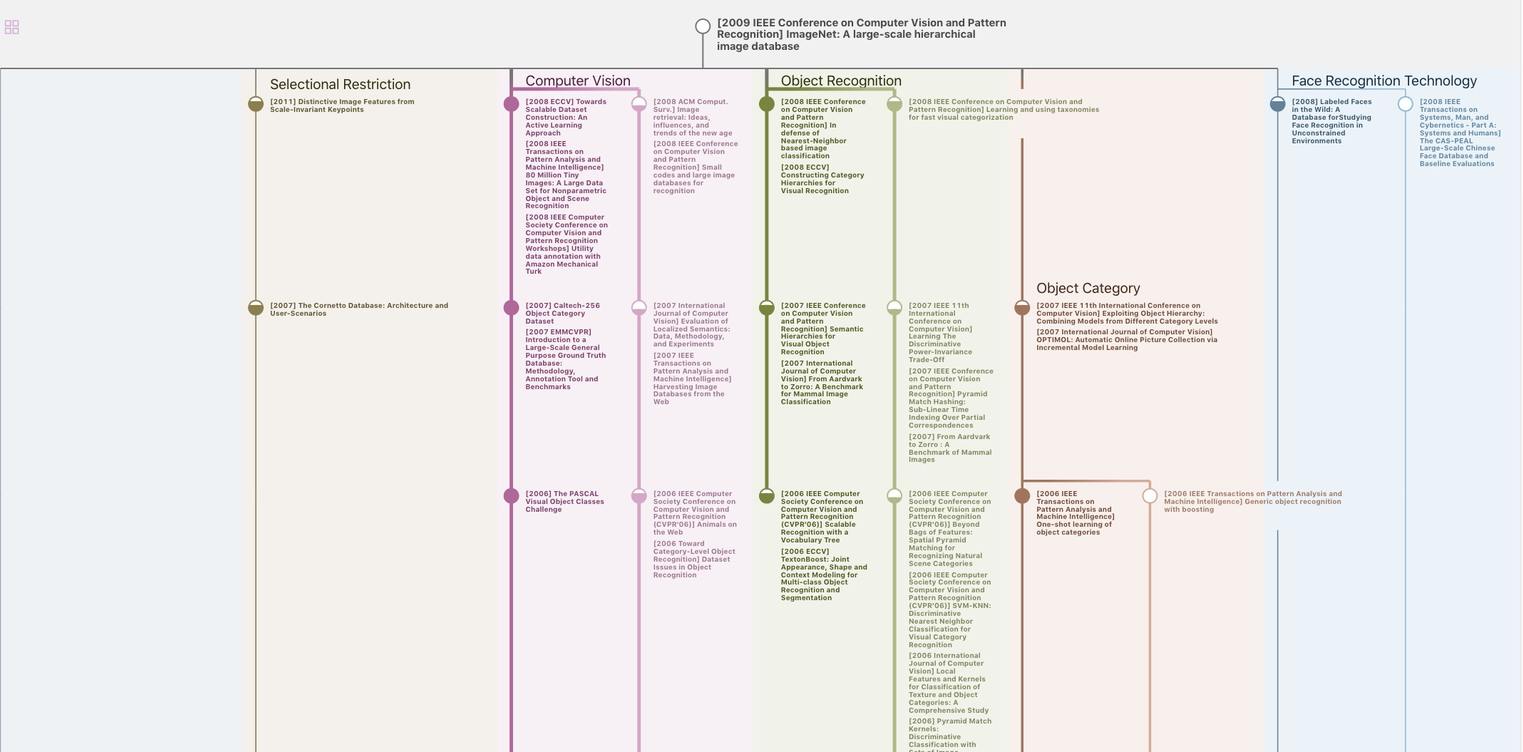
被引用48 | 浏览
被引用6 | 浏览
被引用2 | 浏览
被引用6 | 浏览
被引用14 | 浏览
被引用0 | 浏览
被引用0 | 浏览