Seminal Stacked Long Short-Term Memory (SS-LSTM) Model for Forecasting Particulate Matter (PM2.5 and PM10)
ATMOSPHERE(2022)
摘要
With increased industrialization and urbanization, sustainable smart environments are becoming more concerned with particulate matter (PM) forecasts that are based on artificial intelligence (AI) techniques. The intercorrelation between multiple pollutant components and the extremely volatile PM pattern changes are the key impediments to effective prediction. For accurate PM forecasting with the benefit of federated learning, a new architecture incorporating seminal stacked long short-term memory networks (SS-LSTM) is presented in this research. The historical data are analyzed using SS-LSTM to reveal the location-aware behavior of PM, and a new prediction model is generated that takes into account the most prevalent pollutants and weather conditions. The stacking of LSTM units adds hierarchical levels of knowledge that help to tune the forecast model with the most appropriate weighting to the external features that contribute toward PM. The suggested SS-LSTM model is compared with traditional machine learning approaches and deep learning models to see how well it performs in predicting PM2.5 and PM10. The suggested strategy outperforms all other models tested in experiments carried out for the data collected from Delhi in India.
更多查看译文
关键词
forecasting,air pollution,particulate matter,PM2,5,PM10,LSTM,deep learning
AI 理解论文
溯源树
样例
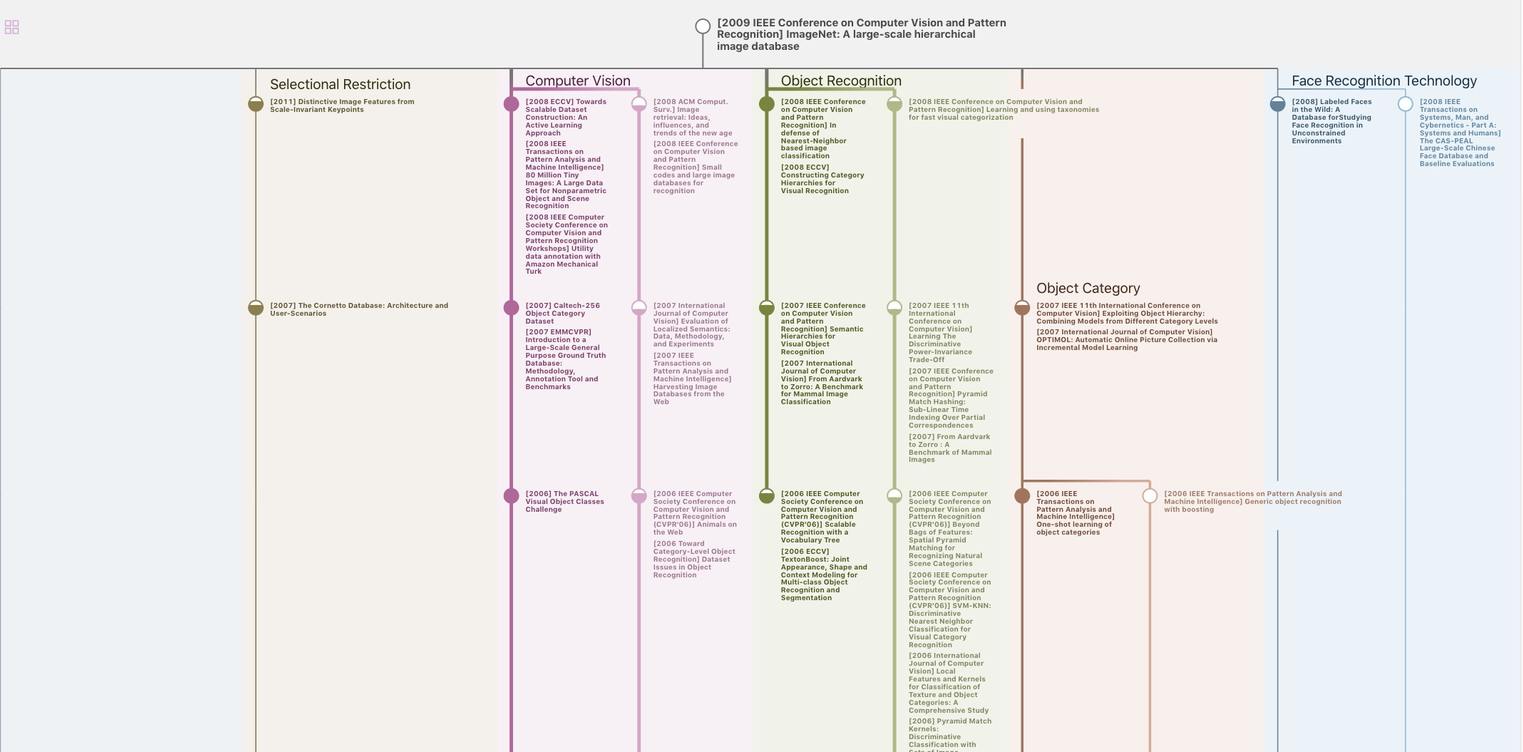
生成溯源树,研究论文发展脉络
Chat Paper
正在生成论文摘要