Managing Shaping Complexity in Reinforcement Learning with State Machines - Using Robotic Tasks with Unspecified Repetition As an Example
2022 IEEE International Conference on Mechatronics and Automation (ICMA)(2022)
Abstract
Reinforcement learning (RL) has been widely used in the field of robotics in recent years. It is an optimization process that finds a policy to maximize the expected reward. Thus, designing a suitable reward function is critical. Sparse reward is easier to design, but for complex tasks, such as robot operations with unspecified repetition, the agent can rarely receive useful reward signals. Dense reward with shaping may be used, but current practices are mostly ad hoc. In this paper, we demonstrate that reward shaping may be done in a more systematic way by using a set of design principles together with the reward machine. We applied the method to train neural networks with RL that can perform block stacking and block lining up tasks with unspecified repetition. The experimental results show that the resultant neural networks can achieve success rates up to 66% and 81% for block stacking and block lining up tasks respectively, which are far better than those using ad hoc sparse rewards.
MoreTranslated text
Key words
Machine learning,Reinforcement learning,Reward design,Reward shaping,Robotic control
AI Read Science
Must-Reading Tree
Example
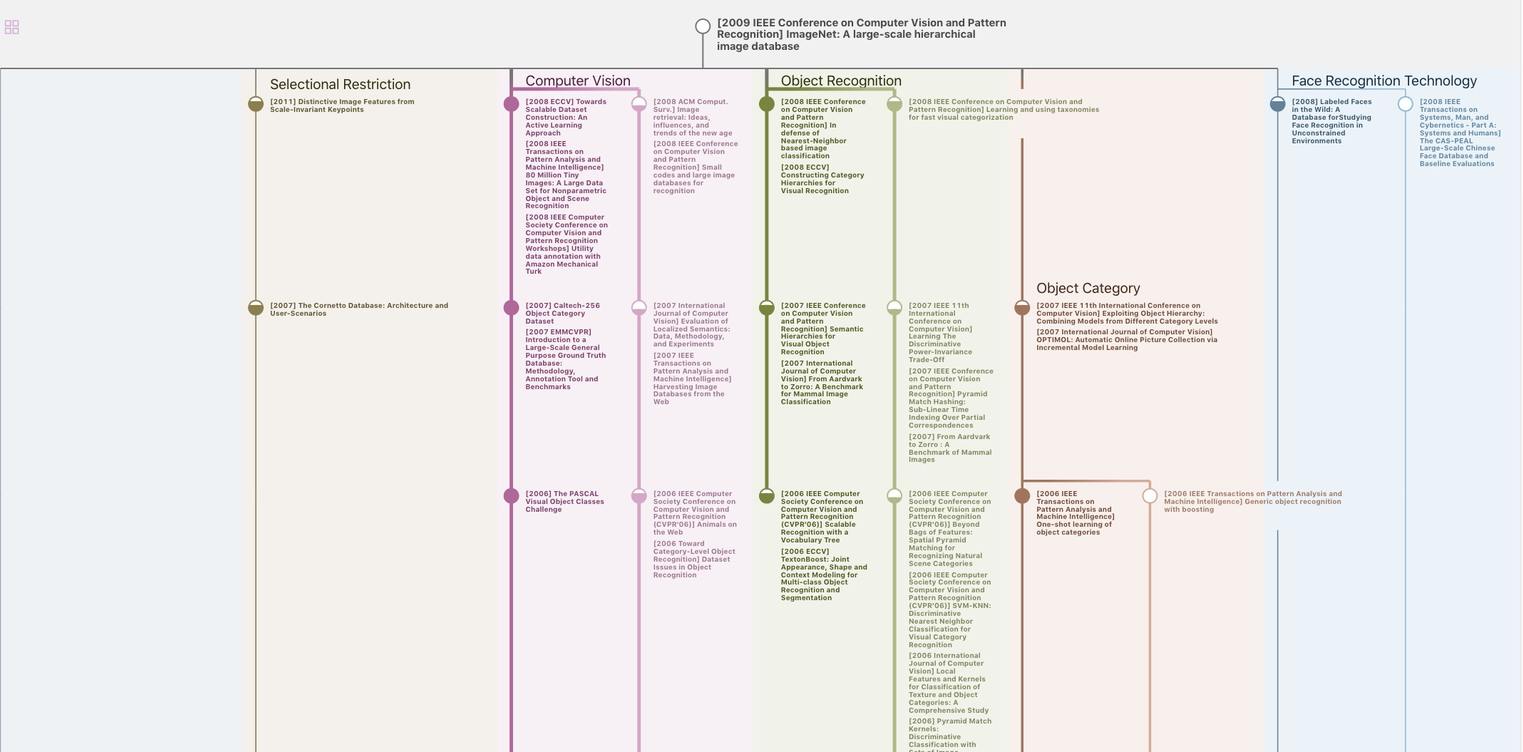
Generate MRT to find the research sequence of this paper
Chat Paper
Summary is being generated by the instructions you defined