A Comparison of Global Sensitivity Analysis Methods for Explainable AI With an Application in Genomic Prediction
IEEE ACCESS(2022)
Abstract
Explainable Artificial Intelligence (XAI) is an increasingly important field of research required to bring AI to the next level in real-world applications. Global sensitivity analysis (GSA) methods play an important role in XAI, as they can provide an understanding of which (groups of) parameters have high influence in the predictions of machine learning models and the output of simulators and real-world processes. In this paper, we conduct a survey into global sensitivity methods in an XAI context and present both a qualitative and a quantitative analysis of these methods under different conditions. In addition to the overview and comparison, we propose an open source application, GSAreport, that allows you to easily generate extensive reports using a carefully selected set of global sensitivity analysis methods depending on the number of dimensions and samples, to gain a deep understanding of the role of each feature for a given model or data set. We finally present the methods discussed in a complex real-world application of genomic prediction and draw conclusions about when to use which GSA methods.
MoreTranslated text
Key words
Analytical models,Sensitivity analysis,Biological system modeling,Machine learning,Uncertainty,Predictive models,Robustness,Explainable artificial intelligence,global sensitivity analysis,machine learning,plant breeding,genomic prediction
AI Read Science
Must-Reading Tree
Example
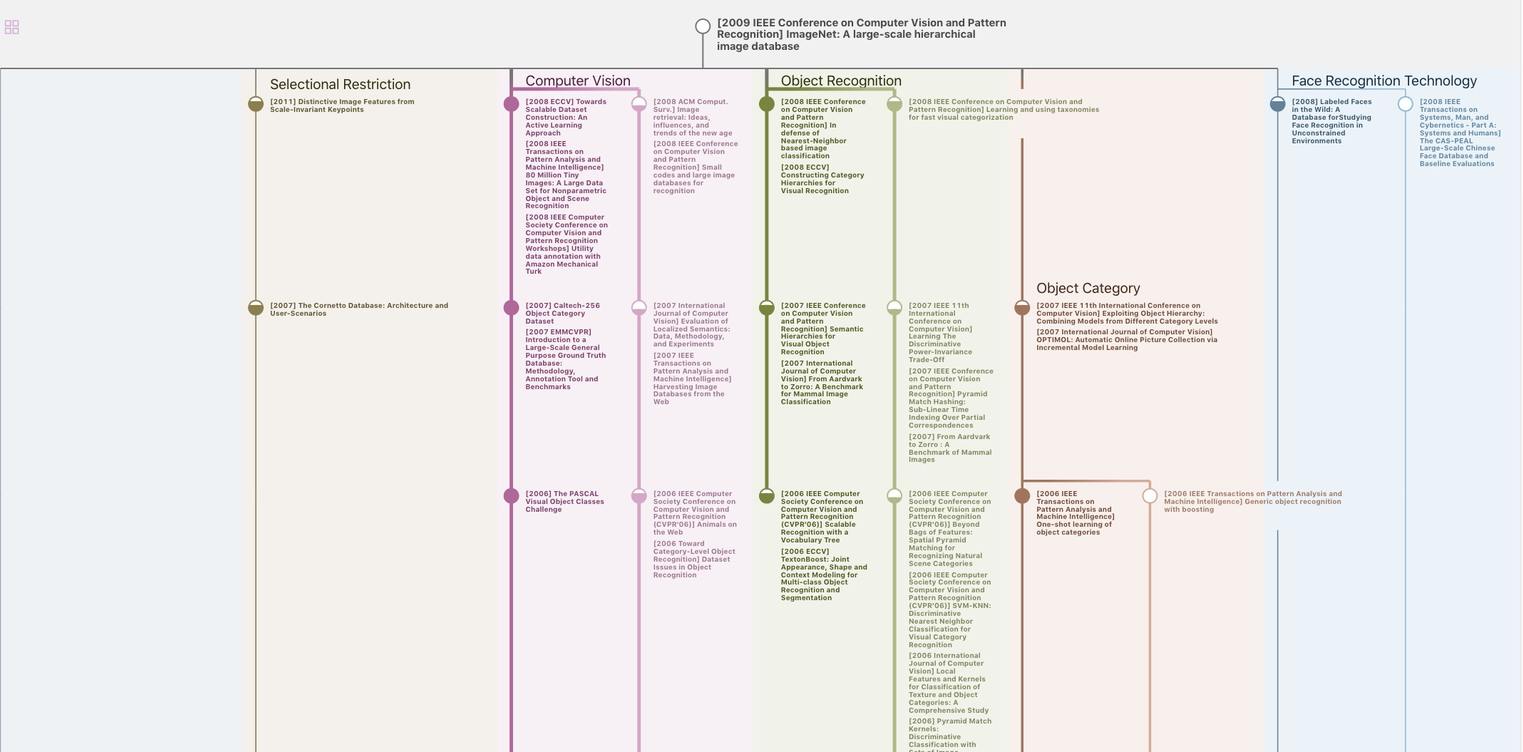
Generate MRT to find the research sequence of this paper
Chat Paper
Summary is being generated by the instructions you defined