Online Malware Classification with System-Wide System Calls in Cloud IaaS
2022 IEEE 23RD INTERNATIONAL CONFERENCE ON INFORMATION REUSE AND INTEGRATION FOR DATA SCIENCE (IRI 2022)(2022)
摘要
Accurately classifying malware in an environment allows the creation of better response and remediation strategies by cyber analysts. However, classifying malware in a live environment is a difficult task due to the large number of system data sources. Collecting statistics from these separate sources and processing them together in a form that can be used by a machine learning model is difficult. Fortunately, all of these resources are mediated by the operating system's kernel. User programs, malware included, interacts with system resources by making requests to the kernel with system calls. Collecting these system calls provide insight to the interaction with many system resources in a single location. Feeding these system calls into a performant model such as a random forest allows fast, accurate classification in certain situations. In this paper, we evaluate the feasibility of using system call sequences for online malware classification in both low-activity and heavy-use Cloud IaaS. We collect system calls as they are received by the kernel and take n-gram sequences of calls to use as features for tree-based machine learning models. We discuss the performance of the models on baseline systems with no extra running services and systems under heavy load and the performance gap between them.
更多查看译文
关键词
Malware Classification,Cloud Computing Security,Dynamic Malware Analysis,Machine Learning
AI 理解论文
溯源树
样例
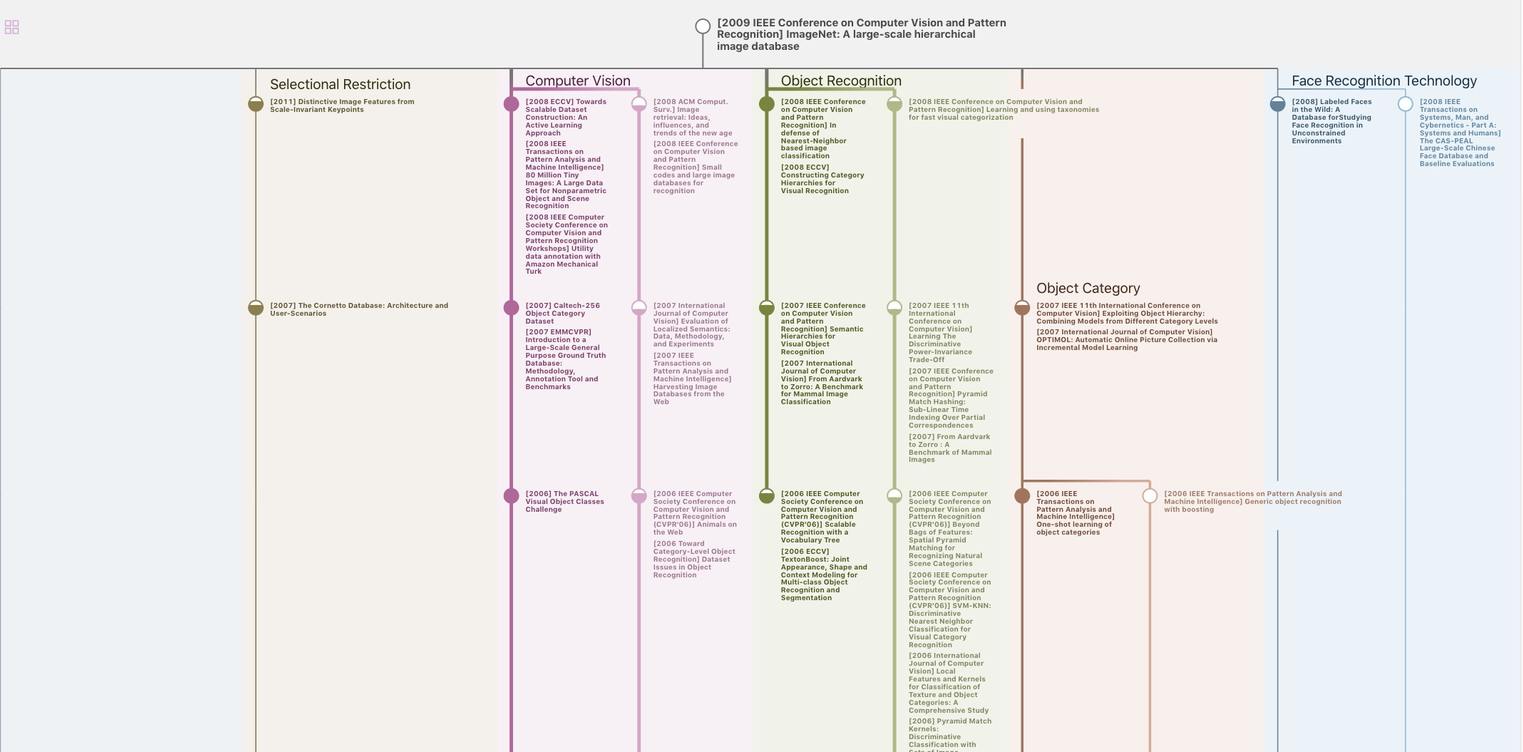
生成溯源树,研究论文发展脉络
Chat Paper
正在生成论文摘要