DATA-ADAPTIVE EFFICIENT ESTIMATION STRATEGIES FOR BIOMARKER STUDIES EMBEDDED IN RANDOMIZED TRIALS
ANNALS OF APPLIED STATISTICS(2022)
摘要
Predictive and prognostic biomarkers are increasingly important in clini-cal research and practice. Biomarker studies are frequently embedded in ran-domized clinical trials with biospecimens collected at baseline and assayed for biomarkers, either in real time or retrospectively. This article proposes efficient estimation strategies for two study settings in terms of biomarker ascertainment: a complete-data setting in which the biomarker is measured for all subjects in the trial, and a two-phase sampling design in which the biomarker is measured retrospectively for a random subsample of subjects se-lected in an outcome-dependent fashion. In both settings, efficient estimating functions are characterized using semiparametric theory and approximated using data-adaptive machine learning methods, leading to estimators that are consistent, asymptotically normal and (approximately) efficient under gen-eral conditions. The proposed methods are evaluated in simulation studies and applied to real data from two biomarker studies, one in each setting.
更多查看译文
关键词
Augmentation, precision medicine, semiparametric theory, super learner, two-phase sampling
AI 理解论文
溯源树
样例
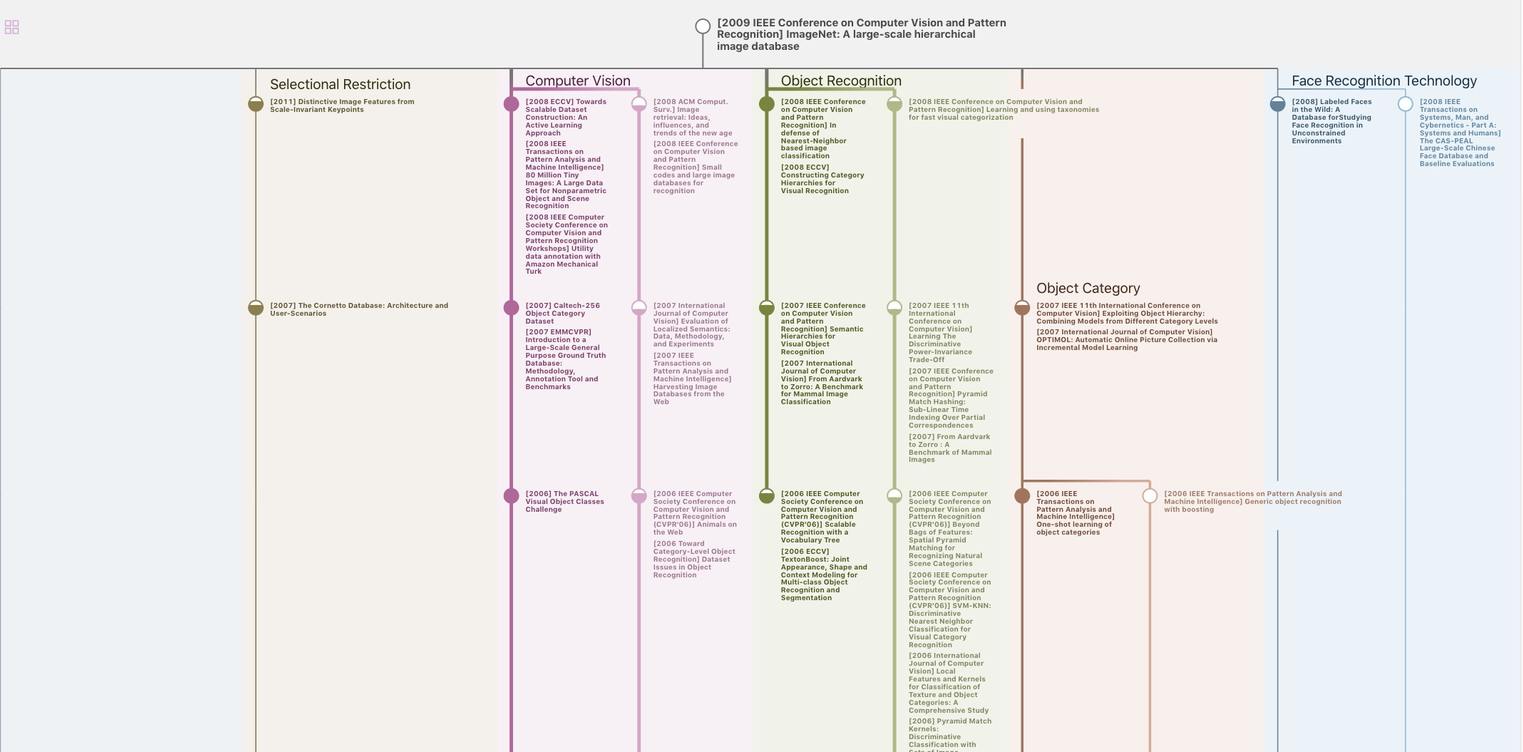
生成溯源树,研究论文发展脉络
Chat Paper
正在生成论文摘要