External Validation of Machine Learning Models for Prediction of Survival in Undifferentiated Pleomorphic Sarcoma.
MUSCULOSKELETAL SURGERY(2023)
摘要
e13551 Background: Machine learning (ML) algorithms to predict cancer survival have recently been reported for a number of sarcoma subtypes, but none have investigated undifferentiated pleomorphic sarcoma (UPS). ML is a powerful tool that has the potential to better prognosticate UPS. Methods: The Surveillance, Epidemiology, and End Results (SEER) database was queried from 2004 to 2015 for cases of histologically confirmed undifferentiated pleomorphic sarcoma (UPS) and malignant fibrous histiocytoma (MFH). Patient, tumor, and treatment characteristics were recorded, and various machine learning (ML) models were built to predict 1-, 3-, and 5-year survival. The best performing ML models were externally validated using an institutional cohort of UPS patients. Results: All ML models performed best at the 1-year time point and worst at the 5-year time point. On internal validation within the SEER cohort, the best models had c-statistics of 0.71 to 0.73 at the 5-year time point. Similarly, all ML models performed best at 1-year and worst at 5-year on external validation. The best performing models had c-statistics of 0.81 at the 5-year time point on external validation, demonstrating good performance in survival prediction. Conclusions: Machine learning models perform well for survival prediction in UPS, though this sarcoma subtype may be more difficult to prognosticate than other subtypes. Future studies are needed to further validate the machine learning approach for UPS prognostication. [Table: see text]
更多查看译文
关键词
Genomic Characterization
AI 理解论文
溯源树
样例
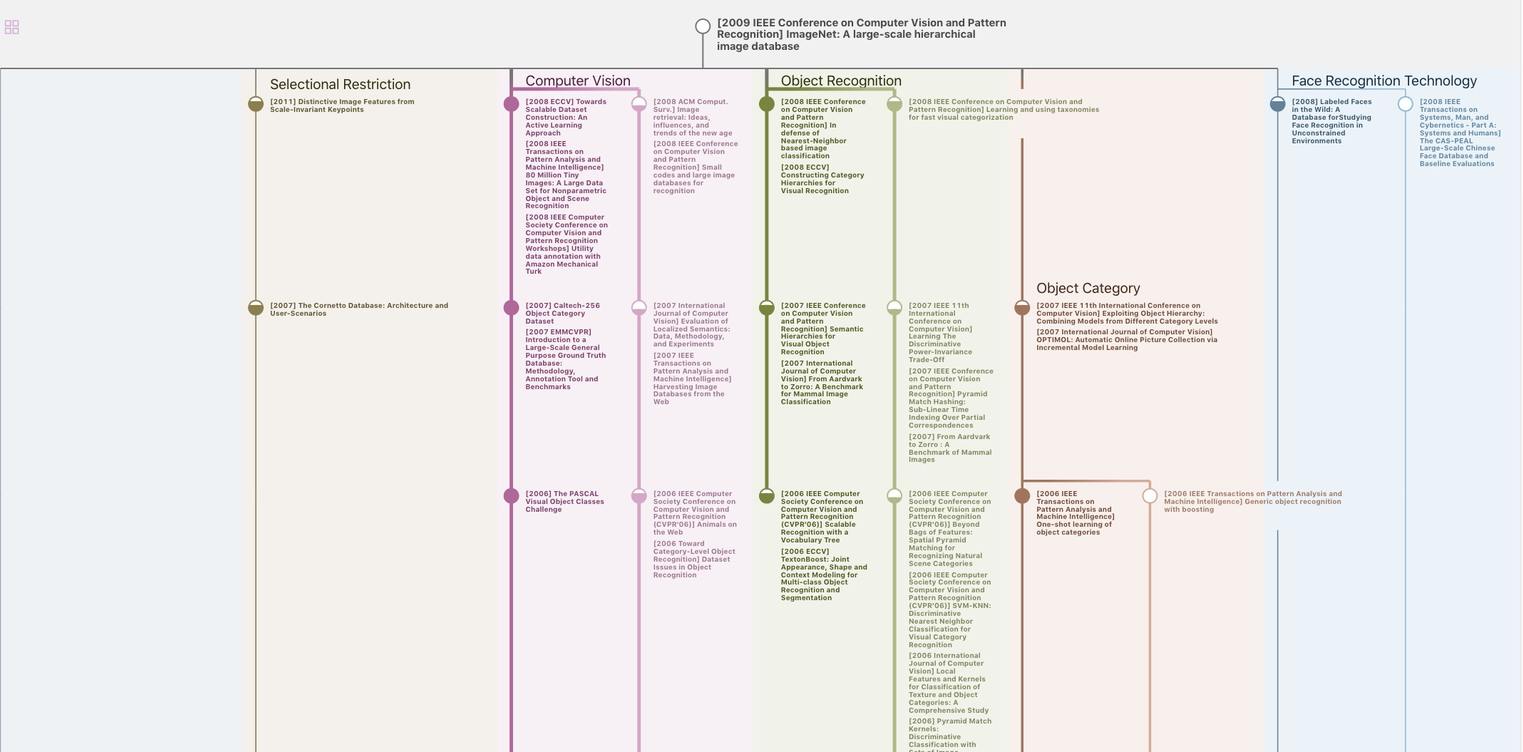
生成溯源树,研究论文发展脉络
Chat Paper
正在生成论文摘要