AI in NSCLC: PET-CT & Histology Model.
Journal of clinical oncology(2022)
摘要
e21044 Background: Almost 70% of cases of lung cancer are diagnosed at advanced stage, albeit with sophisticated imaging modalities available. Of these, 35% are tiny nodules that are often missed at initial radiological screening, owing to limitations of resolution of human vision.Dramatic shift in therapeutic paradigm in the management of lung cancer in the last decade has ushered an era of stratified medicine. In India, one-third population inhabits rural lands;lack of awareness for molecular medicine poses challenges to treating oncologists. AI-based cancer detection and prediction of potential oncogenic driver at diagnosis, will help alleviate patient anxiety caused due to diagnostic delays, aiding in prompt initiation of optimal therapy.Here, a comprehensive deep-CNN based diagnosis of lung adenocarcinoma (LUAD) was implemented using both PET/CT and histopathology images of 100 patients. Methods: Data collection: 143 whole-slide images were downloaded from Dartmouth Lung Cancer Histology Dataset from Biomedical Informatics Research and Data Science website and PET/CT dataset-A Large-Scale CT and PET/CT Dataset for Lung Cancer Diagnosis (Lung-PET-CT-Dx) from The Cancer Imaging Archive. This comprises 251,135 images from 355 participants across 436 studies. Creation of Deep-CNN model: Residual Neural Network - 18 was used for histopathlogic classification followed by Detectron2’s region-based deep-CNN for detection of presence of tumours in lung PET/CT images. Properties such as size, morphology, attenuation, malignancy, and spiculation were also measured. The 18-layer deep network, ResNet-18, detected the histologic patterns using whole-slide histopathology images. Results: Results for 100 patients whose PET/CT images, histopathology images, and mutational status of three genes (EGFR, ALK, ROS) were readily available. The histologic patterns of tumour cells appear to be related to the mutational status of genes. The ResNet-18 CNN could predict various histologic patterns (Acinar, Lepidic, Papillary, Micropapillary, and Solid patterns). Conclusions: CNN models can help in early diagnosis and prompt treatment of lung cancer, if fairly accurate. Larger datasets are needed to improve the same. The final validation of the model is underway and will be reported in near future.[Table: see text]
更多查看译文
AI 理解论文
溯源树
样例
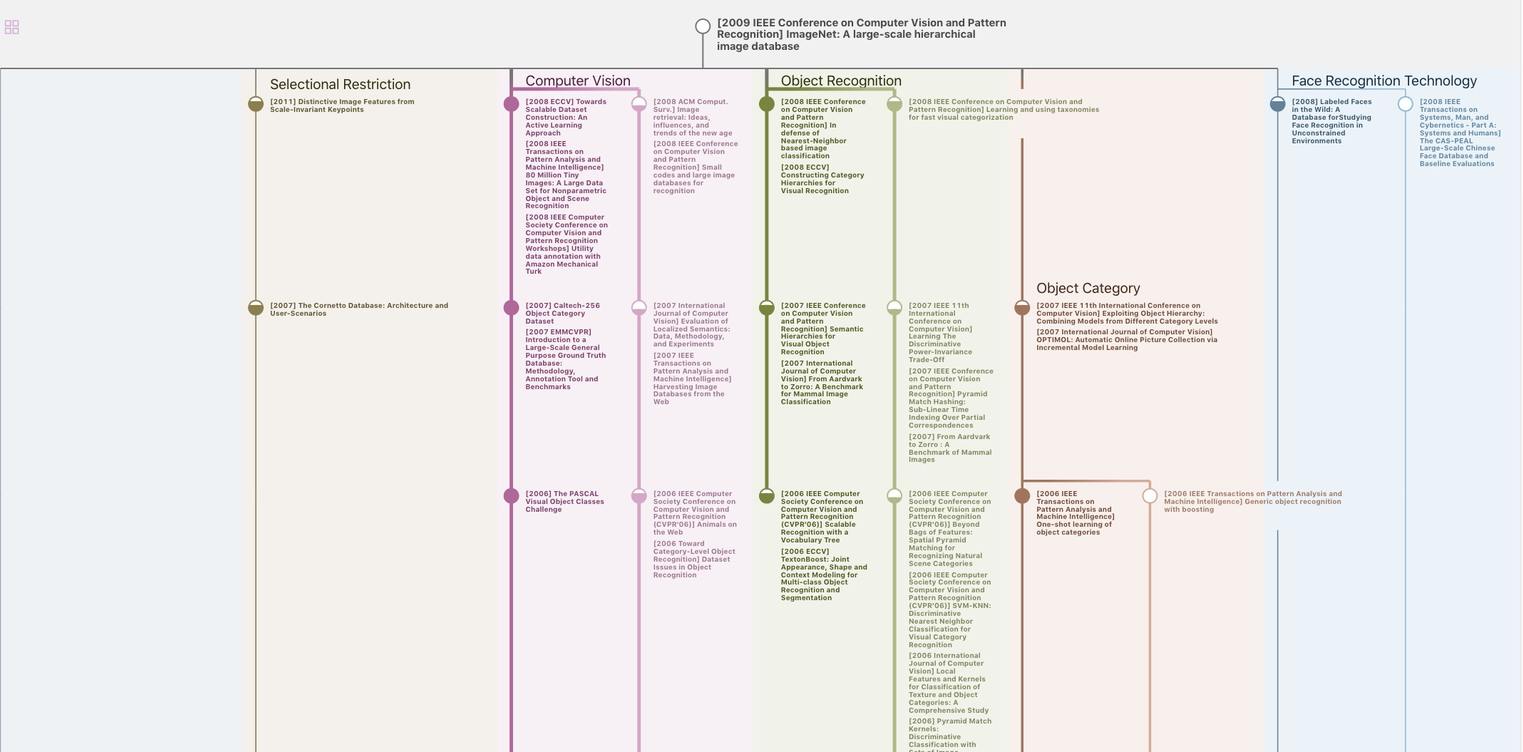
生成溯源树,研究论文发展脉络
Chat Paper
正在生成论文摘要