Constraint Violation Probability Minimization for Norm-Constrained Linear Model Predictive Control
2022 EUROPEAN CONTROL CONFERENCE (ECC)(2022)
摘要
In autonomous driving, it is essential to be able to avoid any type of collision with the environment by appropriate control. Therefore, the distance between vehicle and obstacles needs to be sufficiently large, providing a norm constraint e.g. for optimal control of the vehicle. In general, future positions of dynamic obstacles are highly uncertain and thus predictions are e.g. made using a stochastic model of the obstacle dynamics. We propose an application-independent framework that extends Linear Model Predictive Control to minimize the probability of norm constraint violation in the prediction horizon. Thus, for the autonomous driving application, the probability of collision is minimized In contrast to Robust Model Predictive Control approaches, the proposed approach can deal with unexpected behavior of the obstacle without loss of feasibility.The applicability of the method is demonstrated in simulation of a vehicle that is successfully avoiding a suddenly emerging pedestrian.
更多查看译文
关键词
constraint violation probability minimization,norm-constrained Linear Model Predictive Control,norm constraint e.g,optimal control,general positions,future positions,dynamic obstacles,stochastic model,obstacle dynamics,application-independent framework,norm constraint violation,prediction horizon,autonomous driving application,Robust Model Predictive Control
AI 理解论文
溯源树
样例
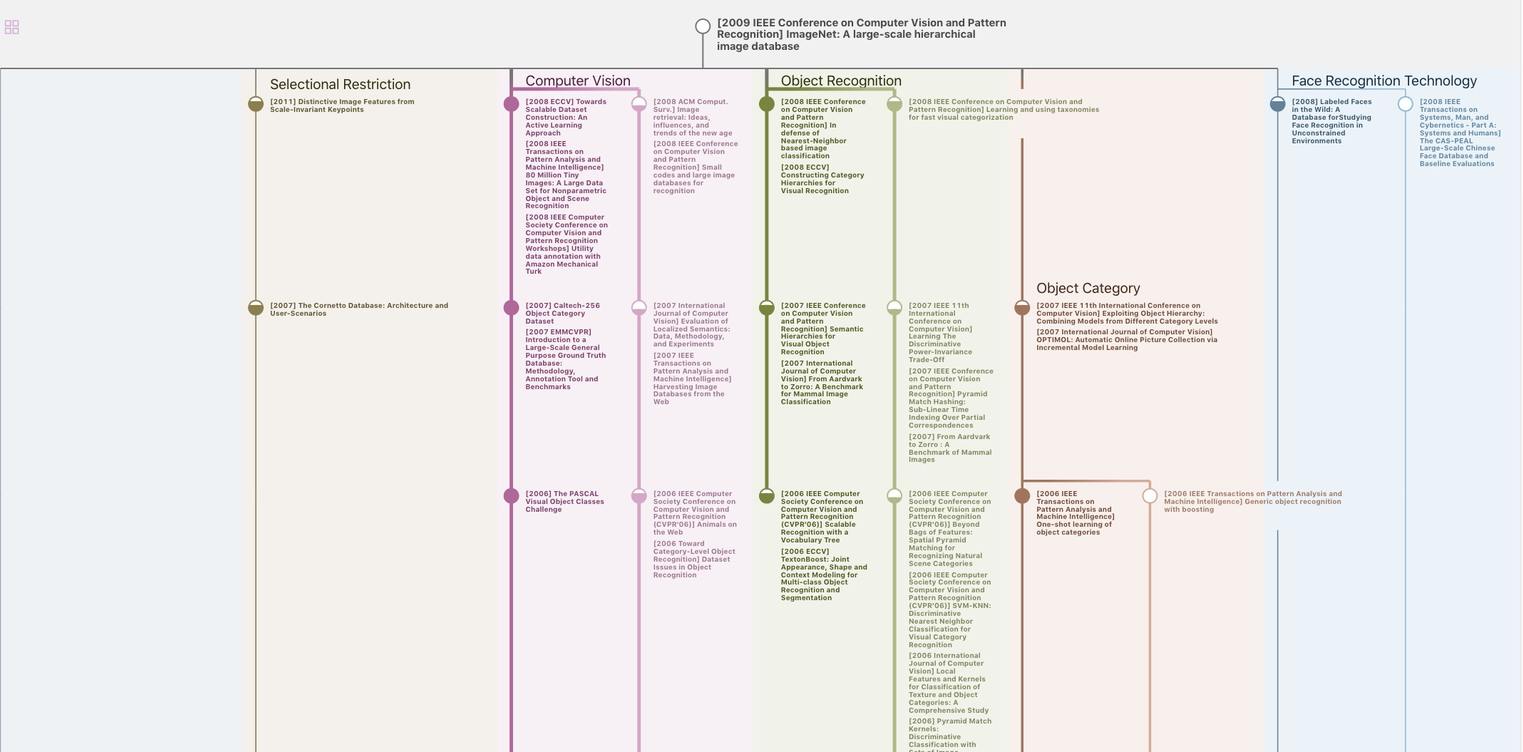
生成溯源树,研究论文发展脉络
Chat Paper
正在生成论文摘要