Robust Training of Graph Neural Networks via Noise Governance
WSDM '23 Proceedings of the Sixteenth ACM International Conference on Web Search and Data Mining(2023)
摘要
Graph Neural Networks (GNNs) have become widely-used models for semi-supervised learning. However, the robustness of GNNs in the presence of label noise remains a largely under-explored problem. In this paper, we consider an important yet challenging scenario where labels on nodes of graphs are not only noisy but also scarce. In this scenario, the performance of GNNs is prone to degrade due to label noise propagation and insufficient learning. To address these issues, we propose a novel RTGNN (Robust Training of Graph Neural Networks via Noise Governance) framework that achieves better robustness by learning to explicitly govern label noise. More specifically, we introduce self-reinforcement and consistency regularization as supplemental supervision. The self-reinforcement supervision is inspired by the memorization effects of deep neural networks and aims to correct noisy labels. Further, the consistency regularization prevents GNNs from overfitting to noisy labels via mimicry loss in both the inter-view and intra-view perspectives. To leverage such supervisions, we divide labels into clean and noisy types, rectify inaccurate labels, and further generate pseudo-labels on unlabeled nodes. Supervision for nodes with different types of labels is then chosen adaptively. This enables sufficient learning from clean labels while limiting the impact of noisy ones. We conduct extensive experiments to evaluate the effectiveness of our RTGNN framework, and the results validate its consistent superior performance over state-of-the-art methods with two types of label noises and various noise rates.
更多查看译文
关键词
graph neural networks,robust training,noise governance
AI 理解论文
溯源树
样例
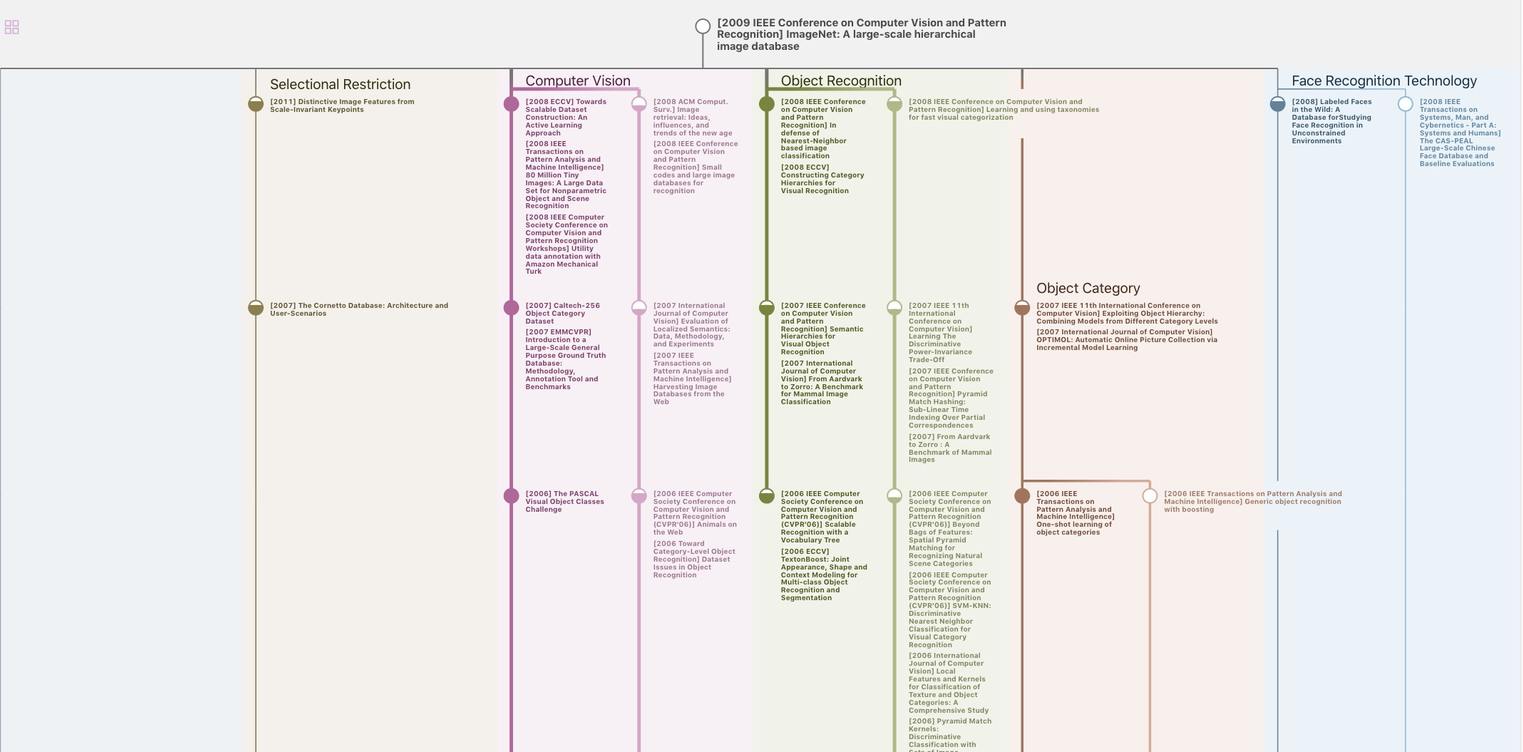
生成溯源树,研究论文发展脉络
Chat Paper
正在生成论文摘要