慢性丙型肝炎病毒感染患者获得持续病毒学应答后肝细胞癌发生的危险因素
wf(2022)
Abstract
目的:探讨慢性丙型肝炎病毒(hepatitis C virus, HCV)感染患者进行抗病毒治疗获得持续病毒学应答(sustained virologic response,SVR)后肝细胞癌发生的危险因素。方法:纳入2012年1月至2019年4月在天津市第二人民医院治疗的慢性HCV感染患者,回顾性分析其肝细胞癌新发情况。采用Cox比例风险模型筛选影响患者发生肝细胞癌的危险因素。结果:644例慢性HCV感染患者中,慢性丙型肝炎421例(65.4%),丙型肝炎肝硬化223例(34.6%),新发肝细胞癌34例,均发生于肝硬化患者。Cox比例风险多因素分析显示:Child-Pugh分级B级以上[风险比(hazard ratio, HR)=6.050,95%可信区间(confidence interval, CI) 2.658~13.771, P<0.001]、饮酒史( HR=3.077, 95% CI 1.428~6.634, P=0.004)、恶性肿瘤家族史( HR=2.376,95% CI 1.155~4.888, P=0.019)、年龄≥60岁( HR=3.301,95% CI 1.563~6.974, P=0.002)、受控衰减参数>292 dB/m( HR=3.842, 95% CI 1.543~9.565, P=0.004)是肝细胞癌的危险因素。 结论:慢性丙型肝炎尤其是肝硬化患者获得SVR后仍有发生肝细胞癌的风险。对于年龄≥60岁、Child-Pugh分级B级以上、存在重度脂肪肝、有饮酒史或恶性肿瘤家族史等高危因素的患者,更需加强肝细胞癌监测。
MoreTranslated text
Key words
Carcinoma, hepatocellular,Hepatitis C virus,Sustained virologic response,Risk factors
求助PDF
上传PDF
View via Publisher
AI Read Science
AI Summary
AI Summary is the key point extracted automatically understanding the full text of the paper, including the background, methods, results, conclusions, icons and other key content, so that you can get the outline of the paper at a glance.
Example
Background
Key content
Introduction
Methods
Results
Related work
Fund
Key content
- Pretraining has recently greatly promoted the development of natural language processing (NLP)
- We show that M6 outperforms the baselines in multimodal downstream tasks, and the large M6 with 10 parameters can reach a better performance
- We propose a method called M6 that is able to process information of multiple modalities and perform both single-modal and cross-modal understanding and generation
- The model is scaled to large model with 10 billion parameters with sophisticated deployment, and the 10 -parameter M6-large is the largest pretrained model in Chinese
- Experimental results show that our proposed M6 outperforms the baseline in a number of downstream tasks concerning both single modality and multiple modalities We will continue the pretraining of extremely large models by increasing data to explore the limit of its performance
Upload PDF to Generate Summary
Must-Reading Tree
Example
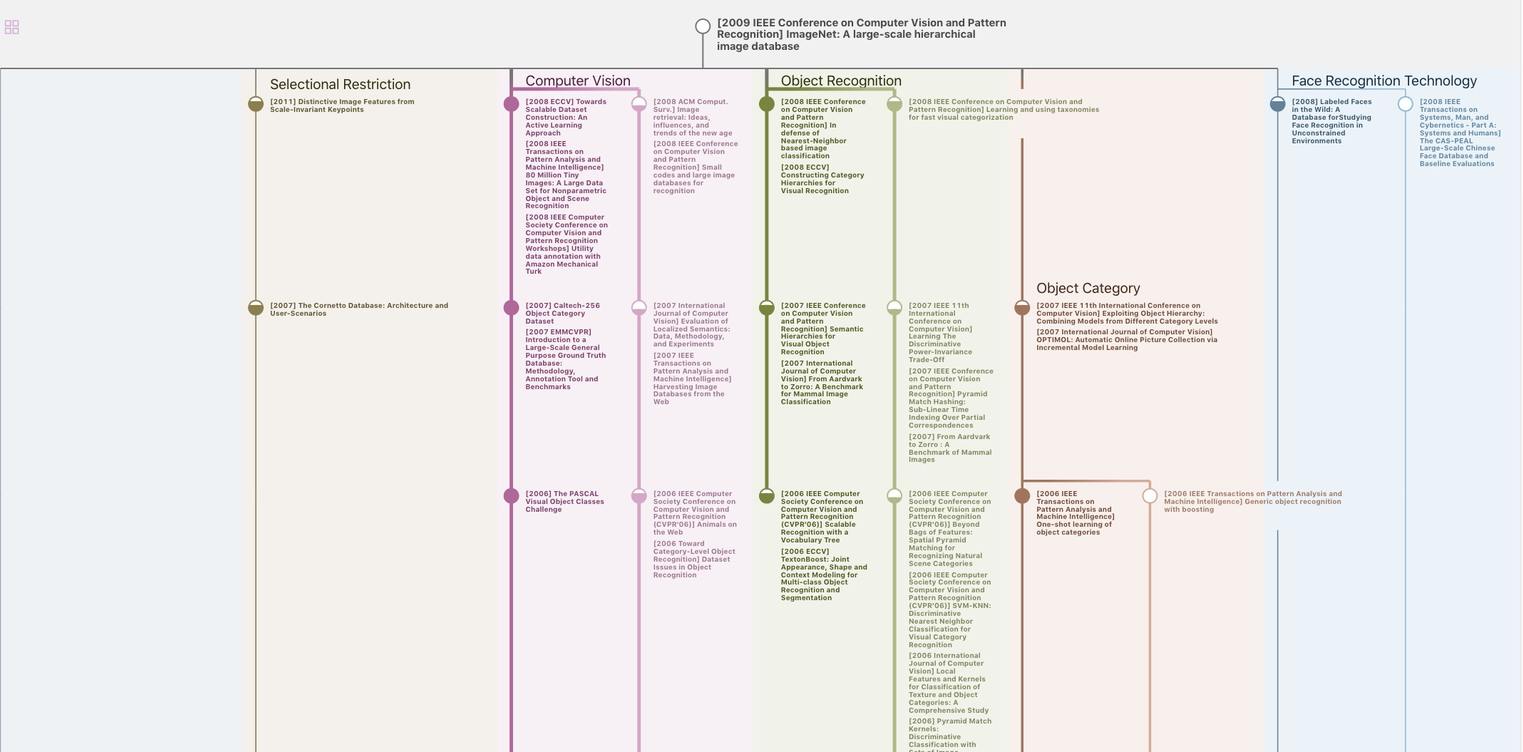
Generate MRT to find the research sequence of this paper
Data Disclaimer
The page data are from open Internet sources, cooperative publishers and automatic analysis results through AI technology. We do not make any commitments and guarantees for the validity, accuracy, correctness, reliability, completeness and timeliness of the page data. If you have any questions, please contact us by email: report@aminer.cn
Chat Paper
GPU is busy, summary generation fails
Rerequest