TransFlowNet: A Physics-Constrained Transformer Framework for Spatio-Temporal Super-Resolution of Flow Simulations.
Journal of computational science(2022)
摘要
We propose TransFlowNet, a novel physics-constrained deep learning framework that focuses on the spatio-temporal super-resolution (STSR) of flow simulations. A key insight is how to combine both statistical and physical properties in the process of an STSR network. Therefore, we elaborately design stacked convolutional layers and Transformer blocks to extract shallow and deep features. Besides, we employ an automatic differentiation process for solving the physical constraints. Unlike existing physics-informed solutions, our method is able to solve flow processes with uncertain boundary and initial conditions. Based on two typical flow simulations, we compare our method with the state-of-the-art physics-constrained model and a CNN-based baseline model. Our framework outperforms these methods in both PSNR and SSIM metrics and produces visually the best results. We also test our method at the large spatio-temporal scale, and the high-resolution outputs present stable performances.
更多查看译文
关键词
Flow simulations,Spatio-temporal super-resolution,Swin Transformer,Implicit feature grid,Physics-constrained framework
AI 理解论文
溯源树
样例
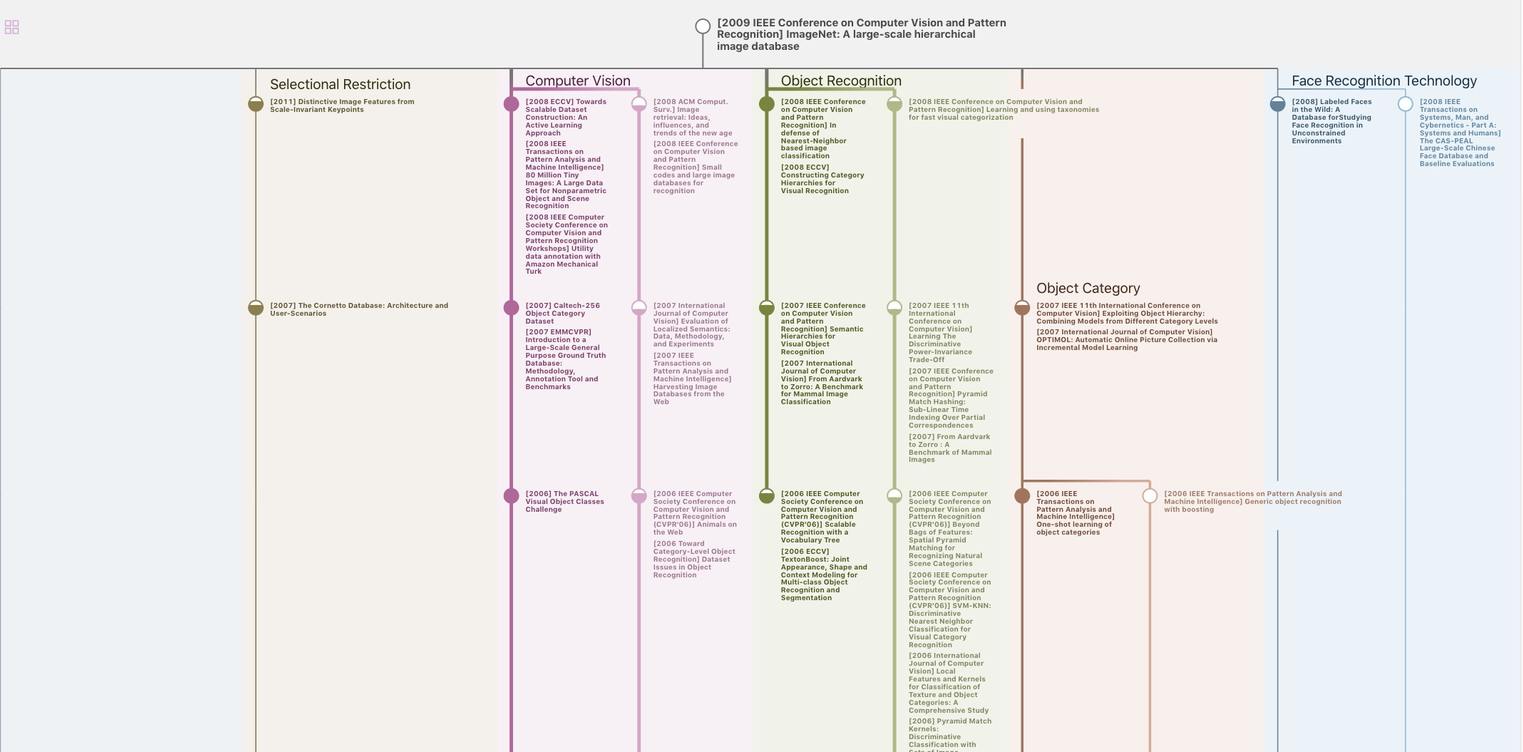
生成溯源树,研究论文发展脉络
Chat Paper
正在生成论文摘要