A Note on Task-Aware Loss via Reweighing Prediction Loss by Decision-Regret
arxiv(2022)
摘要
In this short technical note we propose a baseline for decision-aware learning for contextual linear optimization, which solves stochastic linear optimization when cost coefficients can be predicted based on context information. We propose a decision-aware version of predict-then-optimize. We reweigh the prediction error by the decision regret incurred by an (unweighted) pilot estimator of costs to obtain a decision-aware predictor, then optimize with cost predictions from the decision-aware predictor. This method can be motivated as a finite-difference, iterate-independent approximation of the gradients of previously proposed end-to-end learning algorithms; it is also consistent with previously suggested intuition for end-to-end learning. This baseline is computationally easy to implement with readily available reweighted prediction oracles and linear optimization, and can be implemented with convex optimization so long as the prediction error minimization is convex. Empirically, we demonstrate that this approach can lead to improvements over a "predict-then-optimize" framework for settings with misspecified models, and is competitive with other end-to-end approaches. Therefore, due to its simplicity and ease of use, we suggest it as a simple baseline for end-to-end and decision-aware learning.
更多查看译文
关键词
prediction loss,task-aware,decision-regret
AI 理解论文
溯源树
样例
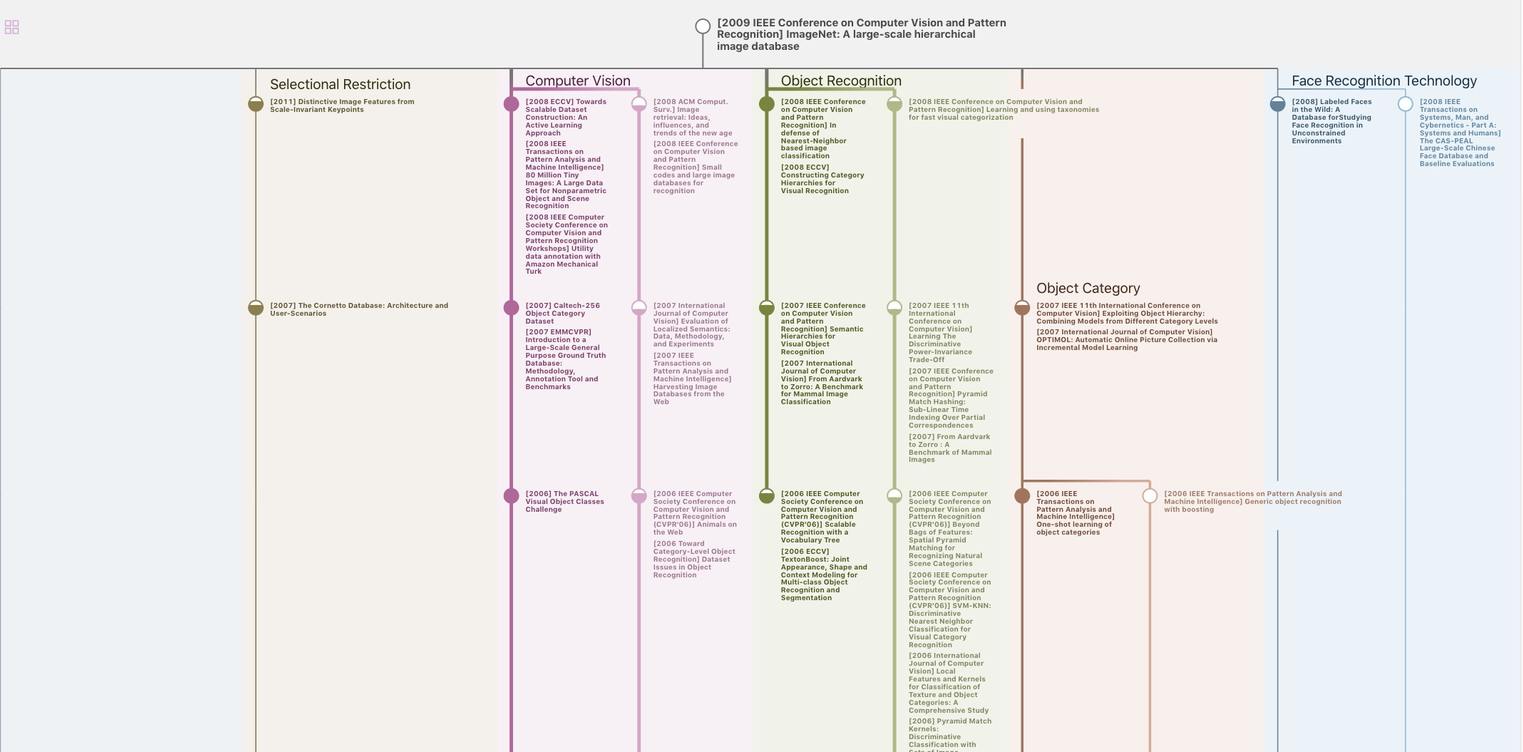
生成溯源树,研究论文发展脉络
Chat Paper
正在生成论文摘要