FAST3: Front-End Electronics to Read out Thin Ultra-Fast Silicon Detectors for Ps Resolution
2022 IEEE LATIN AMERICAN ELECTRON DEVICES CONFERENCE (LAEDC)(2022)
关键词
Silicon radiation detectors,Timing Jitter,UFDS,Ultra-Fast electronics,CMOS
AI 理解论文
溯源树
样例
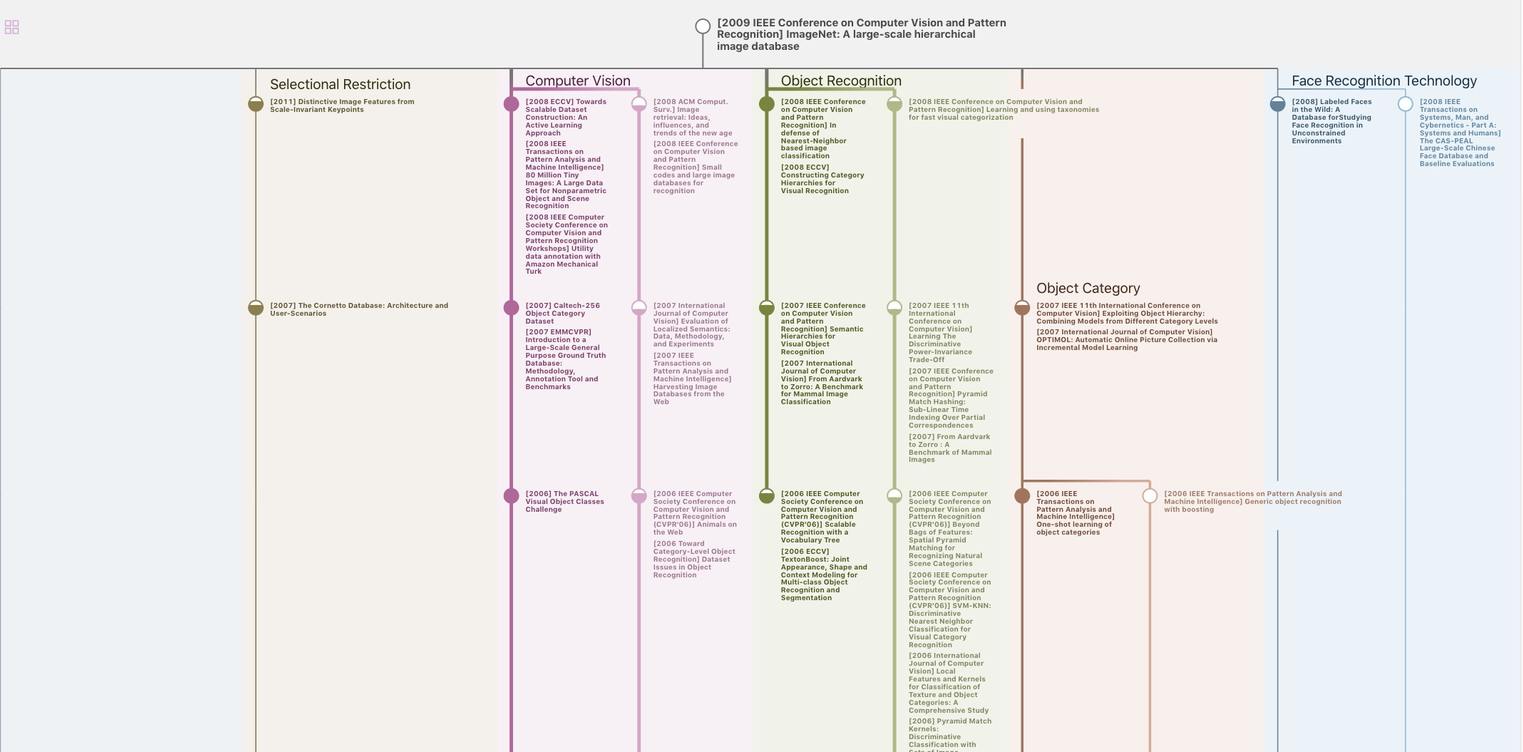
生成溯源树,研究论文发展脉络
Chat Paper
正在生成论文摘要