Trajectory Prediction with Heterogeneous Graph Neural Network.
Pacific Rim International Conference on Artificial Intelligence (PRICAI)(2022)
摘要
Trajectory prediction with dense traffic is a challenging task. The heterogeneity caused by multi-type of road agents complicates the mutual and dynamic relationship between agents. Besides, scene context will affect the trajectory of agents. To address the aforementioned challenges, we present a novel model named HTFNet. Specifically, we use a heterogeneous graph network to model multi-type of agents in traffic. In order to handle varying influence between nodes, interactions between nodes are modelled by a heterogeneous transformer neural network, which uses mate-relation-dependent parameters to distinguish heterogeneous attention over each edge. In addition, scene contexts are considered in multi-model destinations prediction. Through extensive experiments on Stanford Drone Dataset, the results show that our model achieves superior performance on the heterogeneous traffic dataset and produces more reasonable trajectories for different types of road agents.
更多查看译文
关键词
Trajectory prediction,Heterogeneous graph transformer,Multi-type agents
AI 理解论文
溯源树
样例
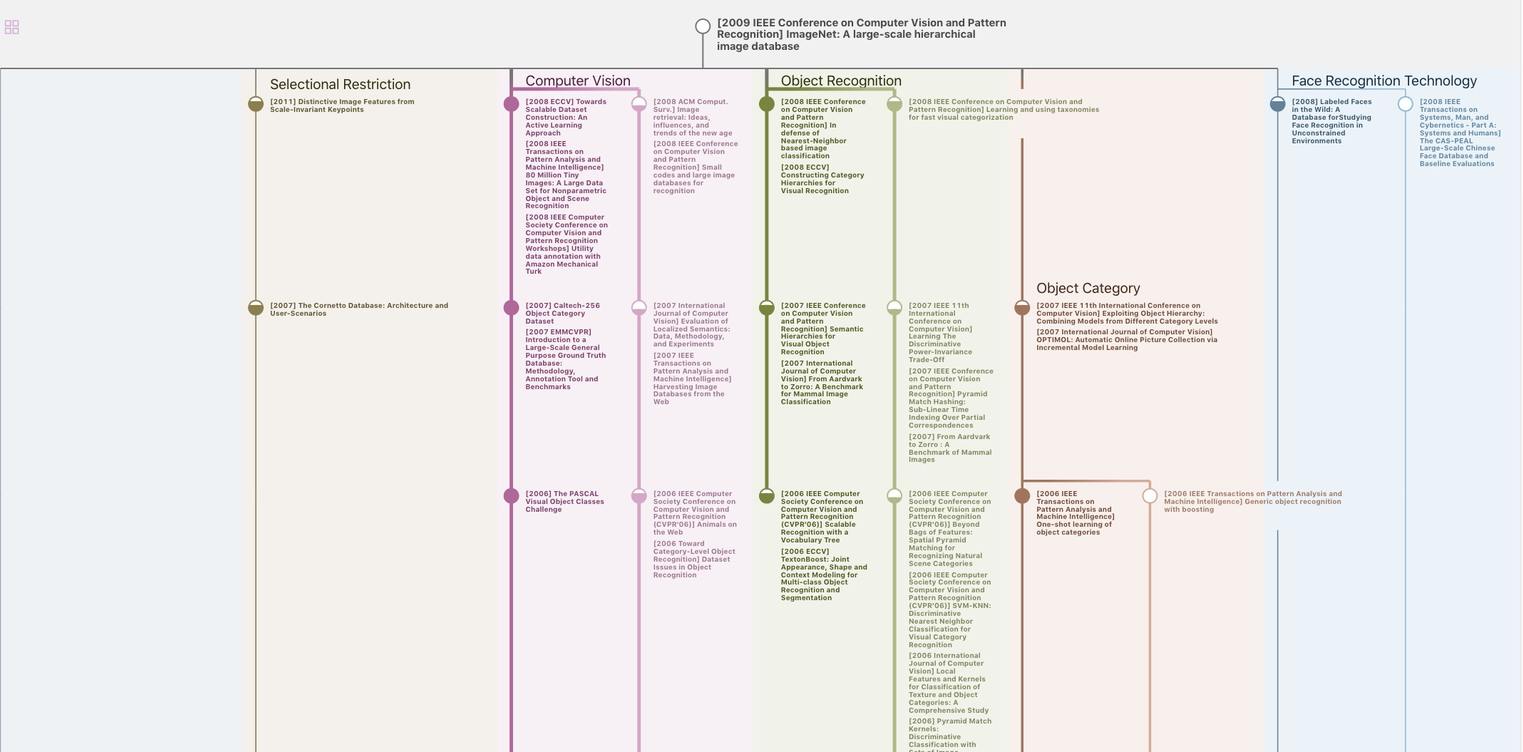
生成溯源树,研究论文发展脉络
Chat Paper
正在生成论文摘要