Convex Clustering through MM: An Efficient Algorithm to Perform Hierarchical Clustering
CoRR(2022)
摘要
Convex clustering is a modern method with both hierarchical and $k$-means clustering characteristics. Although convex clustering can capture the complex clustering structure hidden in data, the existing convex clustering algorithms are not scalable to large data sets with sample sizes greater than ten thousand. Moreover, it is known that convex clustering sometimes fails to produce hierarchical clustering structures. This undesirable phenomenon is called cluster split and makes it difficult to interpret clustering results. In this paper, we propose convex clustering through majorization-minimization (CCMM) -- an iterative algorithm that uses cluster fusions and sparsity to enforce a complete cluster hierarchy with reduced memory usage. In the CCMM algorithm, the diagonal majorization technique makes a highly efficient update for each iteration. With a current desktop computer, the CCMM algorithm can solve a single clustering problem featuring over one million objects in seven-dimensional space within 70 seconds.
更多查看译文
关键词
hierarchical clustering,efficient algorithm
AI 理解论文
溯源树
样例
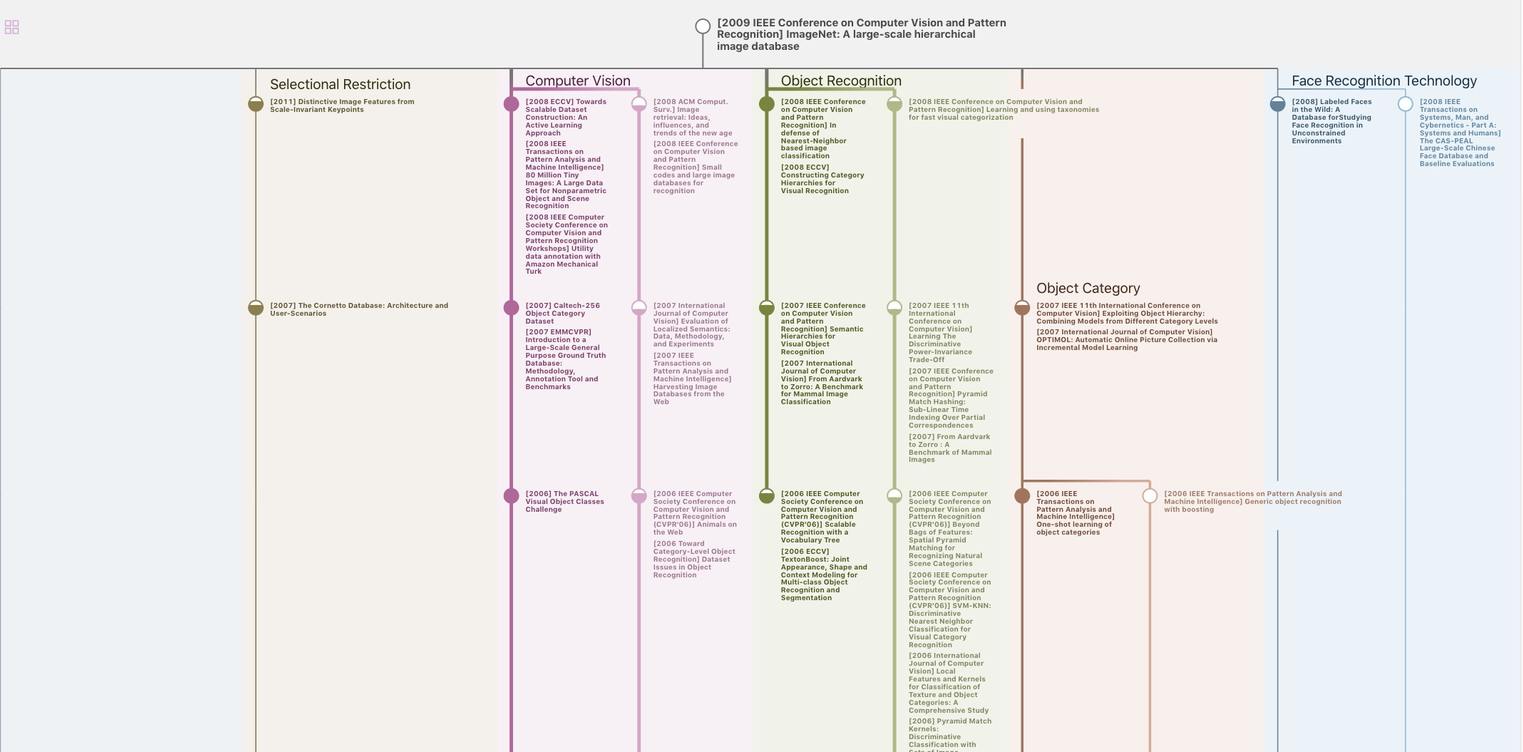
生成溯源树,研究论文发展脉络
Chat Paper
正在生成论文摘要